- Best Practices for Equipment O&M
-
O&M Best Practice Issue Discussions
- Advanced Maintenance Approach: Reliability Centered Maintenance
- Applying Key Performance Indicators
- Comprehensive O&M Program
- Contract Challenges and Improvements
- Cybersecurity for O&M Systems
- Existing Building Commissioning Procurement
- Healthy Building O&M
- Integrating and Analyzing Building Information to Support O&M
- Maintenance Approaches
- OMETA: An Integrated Approach to Operations, Maintenance, Engineering, Training, and Administration
- Prioritizing O&M Actions
- Re-tuning Buildings
- Tools
- Glossary
Integrating and Analyzing Building Information to Support O&M
Table of Contents
- Introduction
- Data Types and Sources
- Data Logistics
- Analysis Approaches
- Data Analytics and Applications
- EIS Cost Structures
- Data Application Case Studies
- Conclusion and Next Steps
- Additional Resources
- Definitions
- References
Introduction
This operations and maintenance (O&M) Best Practice serves to clarify the processes, tools, and actions recommended for integrating and analyzing building information data to make it actionable for a functioning O&M program. Analyzing building information data involves a progression from data to information, and from information to action.
Today’s buildings are experiencing a challenging relationship between sophistication and complexity. This holds true for most new buildings and now, via technology retrofits, many existing buildings as well. This sophistication-complexity trade-off has many potential benefits, with many falling into the category of improved operational efficiency. However, potential downfalls can result from a lack of training, not enough relevant analytical skills, and/or data-driven information overload. To make the best use of these building system improvements, site staff should have access to system training and the relevant tools that allow data to be transformed to actionable information.
At the outset, it should be stated that all buildings and data sources are not created equal—far from it. Each building and building system is unique in its application, environment, and moment-to-moment operation. However, the data type and format can be consistent, and it is these data that need to be understood, analyzed, and used productively.
This Best Practice starts with common taxonomy and definitions, followed by the technologies that provide the data and its format(s), and finally useful paths, techniques, and tools to analyze and make use of these sources. Relevant case studies are referenced as well.
Data Types and Sources
Data that are useful for analysis of building system performance can be collected in a variety of ways and are available from a variety of sources. The most readily available data are collected at the whole-building level by meters installed at the building service entrance. On the other end of the spectrum are data collected at a specific end use by a specialized metering system. Shown below are the major types of building energy-use data presented in increasing levels of intervention (FEMP 2011).
Whole-building. Data collected at the whole-building level are typically collected for billing or other cost-allocation purposes. These data are usually averaged over a utility-based interval (30 days, 1 hour, or 15 minutes) and can include electricity (kWh), natural gas (therms or hundreds of cubic feet CCF), and water (gallons). The source for whole-building data is usually the serving utility or building owner and may come by way of a smart meter connected to a server or cloud data service. Recent advances in smart meter systems, advanced metering systems, and AMR have afforded greater uses for these data.
Whole-panel/subpanel. Electrical metering can be further broken down to specific panels or subpanels when either particular areas of a building or an aggregation of a certain load type (e.g., all lighting) is desired. While not common, natural gas and water can be submetered as well. A source for these data can be the building owner/operator, who may have these metered, and/or via a building automation system (BAS) or a stand-alone data-acquisition system coupled to an energy information system (EIS).
Circuit. The circuit level offers the next level of data resolution. In this case, the circuit or breaker may be feeding a number of small appliances and equipment or could be allocated to just one device. A source for these data can be the building owner/operator; they may also come via the BAS or a stand-alone data-acquisition system coupled to an EIS. If these data are not accessible, metering systems are readily available to accomplish these functions.
Component/End Use. Data collected at the end-use level offers the greatest degree of metering resolution. A source for these data is usually the building owner via a building BAS or a stand-alone data-acquisition system coupled to an EIS. If these data are not accessible, metering systems are readily available to accomplish these functions.
Data Logistics
Data of any type has characteristics that should be identified and understood before analysis, and energy data is not an exception. Four of the most common attributes of data are characterized by data volume, velocity, variety, and value (Lee et al. 2016). These attributes are summarized below in terms of energy (or water) data.
Data Volume. In the energy world, data volume is driven by two factors—the number of data points and the data interval. The number of points relates to the number of fixed measurements that are being captured and stored. At times, these data points can have a multiplicative effect whereby one measurement is disaggregated or combined with another to store more points than the number that is collected.
The data interval can be defined as a period of time over which collected data are sampled, averaged, and then presented. The interval that data are presented in usually varies with the data source. Whole-building data are typically available at the standard 30–31-day billing interval; however, with the advent and use of smart meters, whole-building data are now routinely available at a 1-hour interval and have the capability of a 15-minute interval.
The data intervals for whole-panel, subpanel, and end-use data are typically much more flexible owing to the nature of the data-collection equipment. While hourly interval data are commonly available, panel and subpanel meters are more robust data-collection devices and are usually capable of 1-minute and even 1-second data.
The realities of data volume come down to trade-offs. The higher the resolution of the interval data, the more diagnostic the data become. However, these higher-resolution data come with the penalty of increased quantity; 1-minute data have 60 times the volume of 1-hour data. Fortunately, many modern metering systems allow for data intervals to be changed; therefore, once diagnostic needs are satisfied, the compressed intervals can be reset to less-data-intensive periods.
Higher data volumes require greater data storage and computing power to process. Judicious decisions on the number of data points and the required intervals are recommended for successful data management.
Data Velocity. The velocity of data refers to the speed at which data are transacted from the point of collection to the point of analysis and/or storage. As data-collection devices and systems become increasingly sophisticated and able to provide near-real-time access, the speed of data processing must also be realized. Whether wireless, networked, intranet, or internet based, the speed of data access must be matched by the ability to process it into meaningful metrics. Higher data speeds require appropriate computing and analytical capabilities. Judicious decisions on analytic outcomes and requirements are recommended for successful data management.
Data Variety. As buildings become more sophisticated, so too do their abilities to provide data of different types, forms, units, time stamps, and intervals. The analysis challenge becomes how to coalesce different data types and structures into meaningful relationships that provide useful information. Pre-processing of data is often necessary to align time stamps and intervals before any analysis can take place. Once uniform in time and interval, the data can be analyzed, often by using statistical methods to explore relationships through correlation and regression techniques.
The increasing availability and variety of data requires prudent decisions regarding the need for data pre-processing and conditioning before analysis.
Data Value. The transformation from data to information is where the value lies in integrating and analyzing building information data sources. The modern facility manager has very little time to understand and analyze data. The progression from data to information and then information to action allows for a proactive approach to energy and resource efficiency.
The value of data is only achieved through careful planning, system design, and an analytical approach. A well-planned and sensible approach to data value will help avoid the all-too-common outcome of a data-rich but information-poor system.
Analysis Approaches
With a goal of transforming data to information and ultimately information to action, there is a variety of analytic approaches that can be useful. While someone skilled in the art of spreadsheet and database management could develop the necessary routines and analytics to begin the analysis task, it is the volumes, velocities, and varieties of data that can become overwhelming.
There is a growing field of commercially available EISs that provide customer-facing reporting and energy analytics focused on energy data with actionable outcomes. An EIS can be defined as web-based analysis software, data-acquisition hardware, and communication systems used to collect, store, analyze, and display whole-building, system-level, or equipment-level energy use (Granderson and Lin 2016.; Motegi et al. 2003). Figure 1 highlights the main components of a typical EIS.

Whether an organization decides to develop an in-house EIS or purchase/license a third-party product, it is important to first determine the options and expectations of the EIS in the following areas (FEMP 2011):
- Definition of facility objectives—must be clear on how the EIS will be used, how it will improve resource efficiency, and what its contribution will be to the financial payback of the overall metering system.
- EIS integration with other IT systems—an important consideration before EIS selection and development is determining what existing infrastructure can be used. Before the EIS decision, a survey of existing facility software, hardware, communications, meters, and capabilities is recommended.
- Identify key reporting outputs—how will the outputs of this system be used to fulfill the objectives of the facility and its management? How will the outputs be made available (paper copy, email reporting, web-based reporting), and what sort of summary options are available?
- Data-collection needs—what are the data-collection requirements necessary to achieve the key reporting outputs? Are these available with existing metering elements?
- Data analytics—what analysis, statistical, and/or regression routines are needed to transform the data into the information desired? Are the chosen analytics capable of handling the size, frequency, and complexity of anticipated data?
- Management support—has a budget been developed addressing expenses such as ongoing training, periodic testing, technical assistance, and troubleshooting?
- Data security—any device that is network-connected internally and/or externally and has data and system access security risks. Any and all devices should be screened by proper IT staff for appropriateness and risk potential. Furthermore, the data generated by any metering device have potential privacy and other security risks associated with staff presence and absence, levels of activity, etc. These data should be treated with the same procedures and protocols that are now common with sensitive personal and financial information.
Data Analytics and Applications
Assembled below is a variety of EIS output options available with an in-house-developed or a commercially available EIS. These are grouped into the categories of graphical, analytical, system-specific, and utility outputs. While these categories are not all-inclusive, they should provide some guidance for identifying the advantageous capabilities and outputs of each system.
One additional caution to keep in mind as decisions are made about metering interventions, data points, etc., is the associated volume of data and the necessary time to process and act on the data. The recommendation is to start with a manageable set of outputs that can be useful in the expected time allocated. Then, as the system becomes more integrated, look to add features and other options. To start, the best advice—keep it simple.
Graphical Applications. These are the plots that facility O&M staff interact with daily, weekly, and monthly. Make certain that axes are scaled and labeled for intuitive understanding now and into the future. The O&M value lies in an ability to quickly view differences or unexpected profiles and then take investigatory and corrective actions.
- Daily profile: Time-series daily load profiles are displayed, with time intervals of an hour or less along the horizontal axis and load along the vertical axis (see Figure 2).
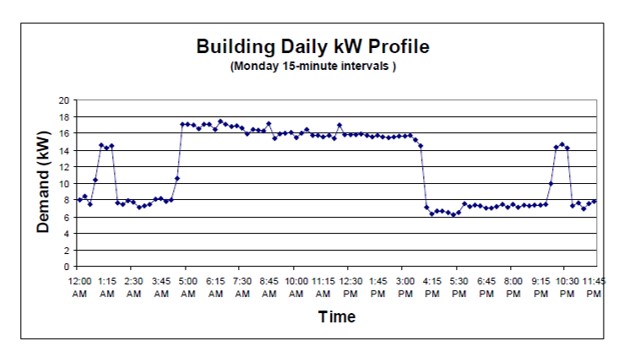
- Day overlay: Overlay plots display multiple daily profiles on a single 24-hour time-series graph.
- Multi-point overlay: Allows multiple time-series data points to be viewed on the same graph.
- 3-D surface chart: Three-dimensional surface charts often display the time of day, date, and variable for study.
- Calendar profile: View up to an entire month of consumption profiles on a single screen as one long time series.
- X-Y scatter plots: X-Y scatter plots are useful for visualizing correlations between two variables.
Analytical Applications. Consider which analyses will be most useful to facility O&M staff and incorporate those into the EIS as an automated function. The goal is to minimize the amount of exporting and re-analyses needed. The intrinsic O&M value is the prioritization of these analytics, affording quick assessment and action.
- Basic statistical analysis: Perform statistical calculations, such as mean, median, standard deviation, correlation, and regression.
- Benchmarking: Benchmark against building energy standards or public databases such as the EnergyStar Portfolio Manager (EPA 2016).
- Intra/interfacility comparisons: Benchmark against the building’s historical data or across multiple buildings in the enterprise (see Figure 3).
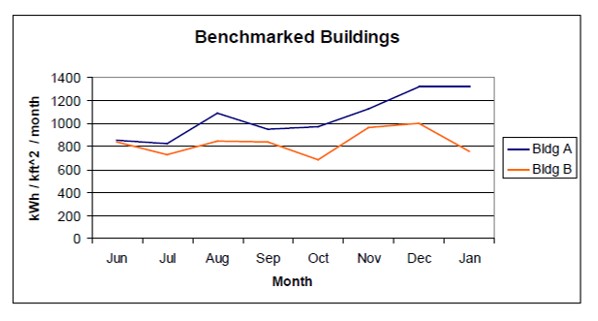
- Aggregation: Aggregate data among multiple data points. Integrate different energy units using energy conversions (e.g., kWh, therm, etc. into Btu).
- Data mining (data slice/drilldown): Sum-up/drilldown time-series data by monthly, weekly, daily, hourly, or trended intervals.
- Normalization: Normalize energy usage or demand by some factors such as building area, number of occupants, outside air temperature, and cooling or heating degree days (CDD, HDD) to make a fair comparison between buildings.
- Hierarchical summary: Summarize usage and cost information by different levels. For example: start from equipment energy cost, individual building energy cost, and site energy cost and go to regional energy cost.
System-Specific Applications. Look for customized analyses beyond the graphical and analytical outputs mentioned above. This type of analysis often takes multiple data points and uses more complicated algorithms. From an O&M perspective, narrowing and focusing on, as well as targeting, certain systems allows for a custom analytical approach and response to prioritized or problematic systems. There is a significant effort in the larger O&M field targeting fault detection and diagnostics (FDD) and recommissioning of building systems. Both of these efforts make use of the below analytics at a minimum and often employ powerful automated tools to facilitate the process (FEMP 2017).
- Power quality analysis: Monitor the voltage or current phases for conditions that could have adverse effects on electrical equipment.
- Measurement and verification: Analysis associated with the confirmation of energy savings resulting from a retrofit project activity (see Figure 4).
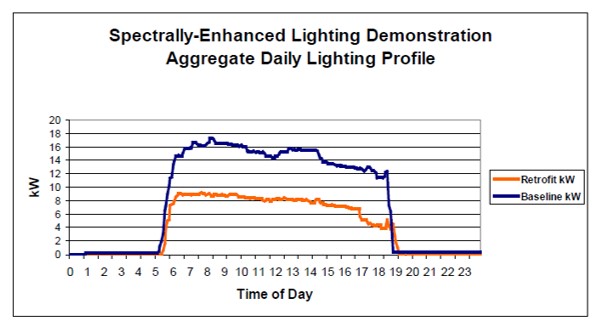
- Forecasting: Forecast future trends by historical data and related parameters such as building occupancy or weather.
- Validation, editing, and estimation: A process performed to assure quantities (kWh, kW, kVar, etc.) retrieved from meters are correct. The process includes validation of data within acceptable error tolerances, editing or correcting erroneous data, and estimating missing data.
- Equipment FDD: Diagnose equipment failure or degradation based on customized algorithms and parameters.
Utility applications. Consider how these data can be useful in interactions with your utilities. Typically, there are tools that allow rate comparisons, bill verification, etc. From an O&M standpoint, verifying utility bills allows for reduced errors and better operations planning.
- Invoice verification (bill validation): Utility bills are compared to meter readings (so-called “shadow” metering) to validate the accuracy of bills.
- Energy cost drilldown: Energy tariff and usage data are used to calculate daily or hourly energy cost breakdowns instead of the usual cost that can only be seen in monthly utility bills.
- Real-time cost tracking: Calculated electricity costs every day or hour using real-time meter reading and rate tariffs.
- End-use cost allocation: Estimated end-use energy consumption from whole-building energy according to user-defined parameters and algorithms. Generally used for cost allocation to building tenants. A common parameter definition is energy use per square foot.
EIS Cost Structures
The cost of a functional EIS is dependent on the development path, in-house versus commercial offering, and the level of intervention. An in-house EIS may have some cost advantage, provided existing staff have the capability and capacity to develop and maintain a functional system.
Commercially available EIS systems typically employ cost models composed of annual or monthly billing and scaled by level of intervention. As EIS systems become more prevalent, cost data are becoming more available. A 2016 study of EIS system costs and savings reported a median 5-year cost of ownership at $1,800 per point or $0.06 per square foot (Granderson and Lin 2016).
Data Application Case Studies
Using whole-building and system-level data for operational and economic efficiency advantages is becoming more common. The case studies below highlight both the opportunities and related economics.
I. Real-Time Energy Management: A Case Study of Three Large Commercial Buildings in Washington D.C. The Natural Resources Defense Council (NRDC) conducted a study of three commercial office buildings in Washington D.C. with the goal of documenting the outcomes and benefits of real-time energy management (NRDC 2013). The project focused on using real-time data to improve operational efficiency using measures that did not require construction, tenant disruption, or substantial capital investment.
Key Findings:
- The 12-month study reported annual electricity savings of 23%, 7%, and 17% for the three buildings and an average overall reported savings of 13.2%.
- The total project expenses for the three buildings was $144,320 for an average expense of $48,107 per building.
- The resulting annual energy dollar savings was reported at $58,352, $47,288, and $113,063 for an average annual savings of $72,901 per building.
Conclusions:
- Energy use in large commercial office buildings can be substantially reduced with attention to energy management to prevent operational stray.
- Energy management through data analytics provides a strong return on investment.
- Energy savings do not require major capital improvements or retrofits.
II. Energy Information Systems: Technology Costs, Benefits, and Best Practices. The Lawrence Berkeley National Laboratory (LBNL) conducted a large-scale assessment of EIS uses, costs, and energy benefits (LBNL 2013). The assessment reviewed 26 case studies of organizations spanning a diversity of climates, EIS technologies, and commercial building types including retail, healthcare, office, and education. The study reviewed year-over-year energy use and utility cost data for nine portfolios and 28 individual sites.
Key Findings:
- The EIS was cited as a critical component in achieving 17% median site annual energy savings and 8% median portfolio annual energy savings.
- The 5-year median cost of ownership was reported at $3,600 per building and $1,800 per point, or $0.06/ft2.
- In cases where sufficient cost and savings information was available, the calculated payback was less than 2 years.
Conclusions:
- The key benefits of EIS use fall into three categories: operational efficiency, utility billing verification, and actionable, customer-focused data and information.
- Best practices found to improve energy savings include adding submetering, employing energy-use benchmarking, and using load profiles for comparison and anomaly detection.
- The study found greater energy savings associated with establishing strong communication between operating and analysis teams.
Conclusion and Next Steps
The integration and analysis of building data to useful information can be a formidable task. Making use of tools and techniques developed either by in-house staff or by third-party EIS vendors can help mitigate this task.
Before any decisions on an analytical path are made, good planning on metering type and data point selection is recommended. It is important to keep in mind the logistics issues of data volume, velocity, variety, and value.
The analysis approaches for building-level data are wide and varied. Starting the analysis planning task with the end in mind, i.e., what result is planned and desired from the assessment, is recommended.
As a function of O&M, the data and resulting analytics relate to inputs for numerous operational efficiency metrics and indicators. There are many O&M key performance indictors (KPIs) that serve as the basis for a functional O&M program, including energy and water use intensities, resource-use trends, and a variety of data-centric, condition-monitoring metrics. Many of these same metrics and KPIs can also be used in the O&M fields of FDD and building Re-tuning™/recommissioning.
The analysis and resulting outcomes can be very powerful tools that lead to improved operational efficiency and economic performance. Recent work in this area has highlighted annual savings in the 13% to 17% range; reported cost data highlight paybacks of under 2 years.
Additional Resources
The Smart Energy Analytics Campaign is a public-private partnership that encourages the use of a wide variety of commercially available energy management and information system (EMIS) technologies and ongoing monitoring practices to help uncover those energy-saving opportunities and improve building performance. The Campaign is offered through the U.S. Department of Energy’s Better Buildings Alliance. Since its launch in 2016, the Campaign has brought together commercial building owners who are using or planning to use EISs, FDD, and Monitoring-Based Commissioning (MBCx). More information is available at https://smart-energy-analytics.org/.
Definitions (FEMP 2011; LBNL 2017; SmartGrid 2015)
To best understand building information data sources, it is important to define common terminology. The below terms, concepts, and technologies fall into the larger realm of energy-related data taxonomy. The sources of this information are found at the end of this section.
- Advanced Meters. Advanced meters are meters that have the capability to measure and record interval data (at least hourly for electricity) and communicate the data to a remote location in a format that can be easily integrated into an advanced metering system.
- Advanced Metering Infrastructure. An integrated system of smart meters, communications networks, and data management systems that enables two-way communication between utilities and customers.
- Advanced Metering System. A system that collects time-differentiated energy or water usage data from advanced meters via a network system either by request or on a defined schedule. The system is capable of providing usage information at least daily and can support desired features and functionality related to energy-use management, procurement, and operations.
- Application Service Provider. Third-party entities that manage and distribute software-based services and solutions (including energy and resource data) to customers across a wide-area network from a central data center.
- Automated Meter Reading (AMR). A form of advanced metering that uses communications devices to communicate from the meter to the meter data management system.
- Base Load. The minimum average electric load over a given period of time.
- Benchmarking. The process of comparing a building’s energy performance to that of similar buildings (cross-sectional benchmarking) or its own historical performance (longitudinal benchmarking). Benchmarking may be performed at the system or component level.
- Building Automation System (BAS). A system of digital controllers, communication architecture, and a user interface that monitors and controls a building’s mechanical and electrical equipment such as heating, ventilation, and air conditioning (HVAC), lighting, fire protection, vertical transport systems, and irrigation systems. The system can be used to optimize facility operation and reduce energy consumption. Also known as building control system or energy management system.
- Computerized Maintenance Management System (CMMS). A computerized system to assist with the effective and efficient management of maintenance activities through the application of computer technology. It generally includes elements such as a computerized work order system, capabilities for scheduling routine maintenance tasks, recording and storing standard jobs, bills of materials, application parts lists, and equipment manuals.
- Degree Day. A measure of the heating or cooling load on a building relative to a “base” outdoor air temperature (e.g., 65 °F). A degree day is commonly calculated as the difference between the mean daily temperature and the “base” temperature.
- Demand. The rate at which electricity is delivered by a system or part of a system, or to a load point or set of loads. Demand is measured in kilowatts, kilovolt amperes, or another suitable unit at a given instant or averaged over a designated period of time.
- End Use. A stand-alone energy-using system or device.
- Energy Information System (EIS). Software, data-acquisition hardware, and communication systems used to store, analyze, and display building energy data.
- Energy Management and Information System (EMIS). A broad family of tools and services to manage building energy use. These technologies offer a mix of capabilities to store, analyze, and display energy use and system data, and in some cases, provide control. EMIS is an umbrella term that covers both meter-level and system-level function.
- Energy Savings. A reduction in energy use from a specific action or set of actions, often quantified by accounting for key normalization factors such as weather or hours of operation.
- Energy Use Intensity. A metric of annual energy use per floor area that is commonly used in benchmarking energy use data.
- Fault Detection and Diagnostics (FDD) Tools. Software products that can automatically identify (detect) deviations from normal or expected operation (faults) and resolve (diagnose) the type of problem or its location.
- Firewall. A system designed to prevent unauthorized access to or from a private network. Firewalls can be implemented in both hardware and software or a combination of both.
- Interval Data. Measurements taken and collected at regular periods for the purposes of future pattern and use analyses.
- Load. The amount of electric power consumed at any specified point or points on a system. Load originates primarily in the power-consuming equipment of the customers.
- Load Aggregation. Aggregation of energy consumption from facilities that are geographically separate from each other for purposes of acquiring and billing utility services.
- Load Duration Curve. A graph showing a utility’s hourly demand, sorted by size, as well as by the amount of time a given level of demand is exceeded during the year.
- Load Factor. The ratio of the average load in kilowatts supplied during a given period to the peak or maximum load in kilowatts occurring during that period. Load factor may be calculated for a customer, customer class, or the entire electric system.
- Load Leveling. A process in which energy demand can be temporarily reduced during certain periods. Typical examples include the intermittent operation of certain electrical equipment and shutting off equipment when rooms or buildings are not in use.
- Peak Load. The maximum anticipated electrical demand for any given system during a specified period of time.
- Real-time Metering. Metering that records consumer use in the same time frame as pricing changes in the market, typically hourly or more frequently.
- Real-time Pricing. The instantaneous pricing of electricity based on the cost of electricity available for use at the time the electricity is demanded by the customer.
- Smart Meter. Resource metering devices that allow data capture, storage, and communication typically used for monitoring and billing purposes.
- Submetering. A method of using multiple meters to collect real-time energy data from any energy-consumption-related source in a building (electricity, water, gas, or other uses such as district steam and chilled water). Submeters capture information that is downstream of the main utility meter and can be used to disaggregate building loads and provide more granular visibility into energy consumption.
- Trend Log. A record of data that may be stored at regular, equal time intervals or upon change of value and that is often used in analyses of use and performance over time.
- Watt. The electrical unit of power. The rate of energy transfer equivalent to 1 ampere flowing under a pressure of 1 volt at unity power factor.
- Watt-hour. The total amount of energy used in one hour by a device that requires one watt of power for continuous operation. Electric energy is commonly sold by the kilowatt-hour (1,000 Watt-hour).
References
EPA – Environmental Protection Agency. 2016. An Overview of Portfolio Manager. U.S. Environmental Protection Agency, EnergyStar Program, Washington, D.C. https://www.energystar.gov/sites/default/files/tools/An%20Overview%20of%20Portfolio%20Manager.pdf
FEMP – Federal Energy Management Program. 2017. Fault Detection and Diagnosis (FDD) Tools for Retro-Commissioning and Continuous Commissioning of HVAC and Refrigeration Systems. U.S. Department of Energy, Federal Energy Management Program, Washington D.C. Available at: https://www.energy.gov/eere/buildings/downloads/fault-detection-and-diagnosis-fdd-tools-retro-commissioning-and-continuous
FEMP – Federal Energy Management Program. 2011. Metering Best Practices: A Guide to Achieving Utility Resource Efficiency. U.S. Department of Energy, Federal Energy Management Program, Washington D.C. https://www.energy.gov/sites/prod/files/2013/10/f3/mbpg.pdf
Granderson, J, G Lin. 2016. “Building Energy Information Systems: Synthesis of Costs, Savings, and Best-practice Uses.” Energy Efficiency 9(6):1369–1384. DOI: 10.1007/s12053-016-9428-9. https://www.osti.gov/pages/servlets/purl/1363638
LBNL – Lawrence Berkeley National Laboratory. 2017. Using EMIS to Identify Top Opportunities for Commercial Building Efficiency. LBNL-1007250, Lawrence Berkeley National Laboratory, Berkeley, CA. https://eta.lbl.gov/sites/all/files/publications/1007250.pdf
LBNL – Lawrence Berkeley National Laboratory. 2013. Energy Information Systems (EIS): Technology Costs Benefits, and Best Practice Uses. LBNL-6476E, Lawrence Berkeley National Laboratory, Berkeley, CA. https://eta-publications.lbl.gov/sites/default/files/6476e.pdf
Lee, C K M, Y Cao, K H Ng. Big Data Analytics for Predictive Maintenance Strategies. Published in Supply Chain Management in the Big Data Era. December, 2016. DOI: 10.4018/978-1-5225-0956-1.ch004 https://www.igi-global.com/gateway/chapter/171283
Motegi, N, M A Piette, S Kinney, and J Dewey. 2003. Case Studies of Energy Information Systems and Related Technology: Operational Practices, Costs, and Benefits. Prepared by Lawrence Berkeley National Laboratory for the California Energy Commission, Public Interest Energy Research, HPCBS # E5P2.2T1e. LBNL Report No. 53406. International Conference for Enhanced Building Operations. https://eta-publications.lbl.gov/sites/default/files/lbnl-53406.pdf
NRDC – Natural Resources Defense Council. 2013. Real Time Energy Management: A Case Study of Three Large Commercial Buildings in Washington, D.C. CS: 13-07-A, Natural Resources Defense Council, Washington D.C. https://www.nrdc.org/sites/default/files/tower-companies-case-study.pdf
Smart Energy Analytics Campaign. Welcome to the Smart Energy Analytics Campaign. U.S. Department of Energy’s Better Buildings Alliance, Washington, DC. https://smart-energy-analytics.org/.
SmartGrid. 2015. Advanced Metering Infrastructure and Customer Systems. U.S. Department of Energy, Office of Electricity Delivery and Energy Reliability, Washington D.C. https://www.smartgrid.gov/recovery_act/deployment_status/sdgp_ami_systems.html
Actions and activities recommended in this Best Practice should only be attempted by trained and certified personnel. If such personnel are not available, the actions recommended here should not be initiated.
Published April 2021