Thermal Dynamics Surrogate Modeling for Commercial Buildings
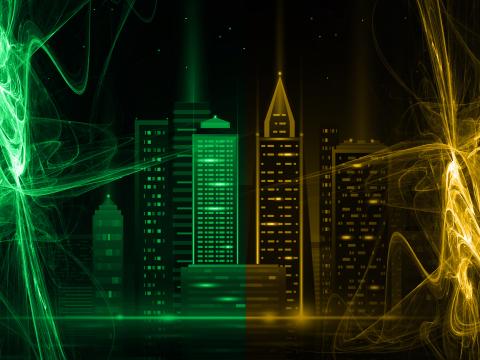
Team
Yunzhi Huang, leader; Jan Strube, Emily Saldanha, Malachi Schram, Vikas Chandan
Problem
Machine learning-based prediction techniques have been applied with success in various energy applications. However, the limitation of purely data-driven predictions has also been discovered. In this study, we use PIML techniques to improve 1) sample efficiency; 2) ability in long-term forecasting; 3) applicability to a real system; and 4) tolerance in error and variance of the inputs. These improvements largely enable the PIML prediction model to be used for predictive operation, fault prevention, smart control, and optimization.
Approach
This study uses the data from a commercial building on the PNNL campus to explore a couple PIML approaches, including 1) NeuralODE approach, 2) physics-informed neural network approach, and 3) pre-training with an uncalibrated white-box model. We compare these PIML approaches with a vanilla long short-term memory (LSTM) model as the baseline, and we explore the prediction accuracy.
Results
The outcome of the study focuses on developing a predictive model suitable for control—the first challenge of the given dataset. The given dataset includes 2 years of data. We will use the first year’s data for training and the second for performance verification. The trained physics-informed LSTM model’s performance of both proposed scenarios will be compared to the purely data-driven model and the purely physics-informed model (EnergyPlus). Accuracy of the power consumption prediction will meet the requirement of 85 percent accuracy (compared to ground truth) and error in modeling indoor temperatures <0.5°C during the prediction period.
Code, Data, and Related Links
In progress.
Publications
In progress.