Reduced-Order Modeling of Vadose Zone Transport in the Hanford 300 Area
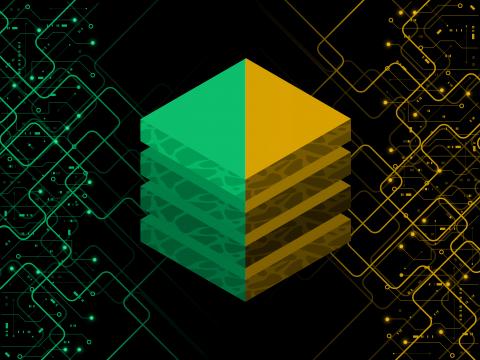
Team
Jason Hou, lead; Mike Friedel, Fred Zhang, Tim Johnson
Problem
Gigabytes of spatiotemporal geophysical and hydrogeologic data from the subsurface at the Hanford Site in Washington State have been collected and archived. When attempting to predict the transport of fluids and chemicals through the vadose zone, traditional physics-based (PB) processing of these data result in nonunique and uncertain property estimates that lead to ambiguous results. This task aims to develop and test physics-informed learning algorithms for improved subsurface characterization and prediction.
Approach
A set of ML algorithms are used to compare/contrast parameter estimates and uncertainty when using the traditional PB (baseline) method and when using the physics-informed learning (novel) method using the available vadose zone monitoring data consisting of spatiotemporal water, heat, solute properties as well as pressure head, temperature, and concentration measurements. The baseline PB code reflects the open-source solution of coupled water, heat, and solute transport into a multilayer vadose zone analogous to the Hanford 300 area. The algorithms couple multi-physics numerical modes (VST2D) and surrogate ML modes, such as random forest and gradient boosting.
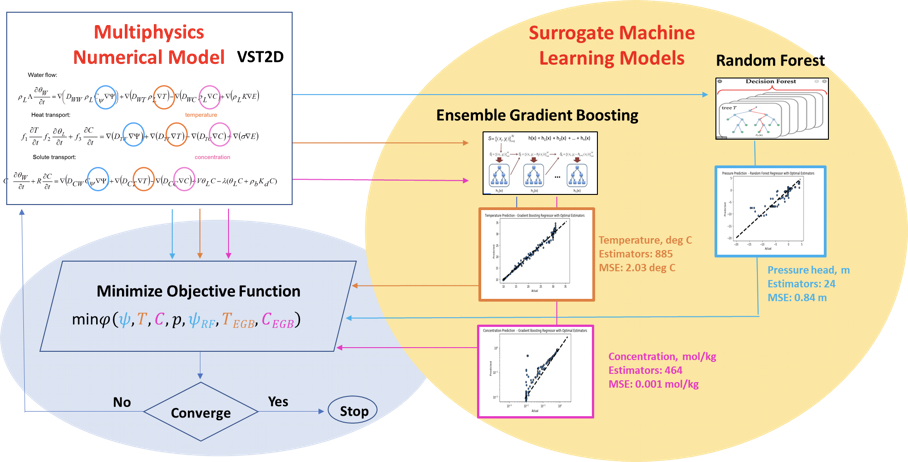
Results
A joint solution of PB code and ML kernel improved subsurface characterization through reduced parameter uncertainty. The introduction of PB constraints in the ML kernel results in a reduced-order model for simulating vadose zone transport without requiring traditional high-performance computing resources. Also, the introduction of ML into the numerical inverse process makes possible the addition of ancillary information for direct predictions of subsurface properties and/or features.
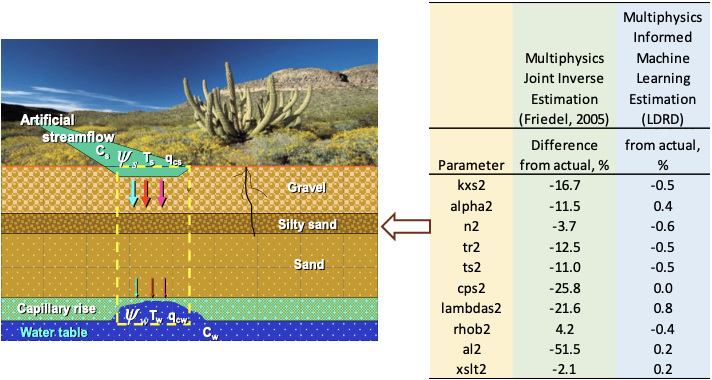
Code, Data, and Related Links
In progress.
Publications
In progress.