Research
Partial differential equation-based modeling of macroscales (task lead: Mark Ainsworth) develops numerical methods for partial differential equations that remove the tyranny of grids, provide controlled accuracy, are suitable for nonlocal interactions, and allow flexibility in moving across scales.
Stochastic modeling of mesoscales (task lead: Panos Stinis) focuses on coarse-graining and model reduction based on the "stochastic" Mori-Zwanzig formulation enhanced by using deep learning techniques.
Bridging methods to connect the scales (task lead: Pavel Bochev) develops coupling by active learning of the microstructure in subdomains and the hidden physics of interfaces in adjacent multiscale domains with no scale separation.
Statistical Learning (task lead: Eric Darve) studies uncertainty, high-dimensionality, robustness, efficiency, and learnability in general neural and adversarial networks to greatly enhance their performance for PhILMs.
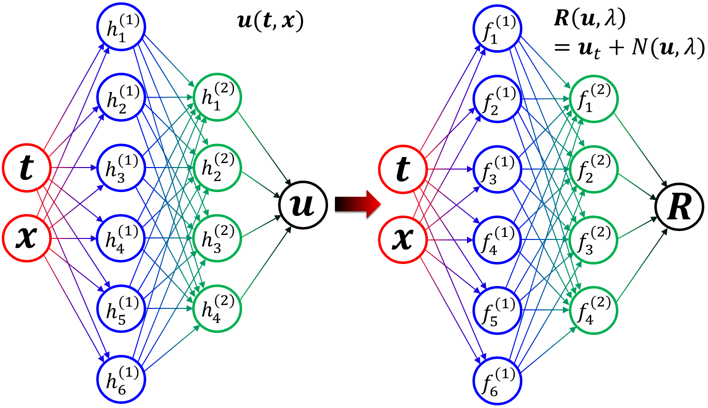