PNNL @ AAAI 2023
PNNL researchers bring their expertise to the 37th AAAI Conference on Artificial Intelligence
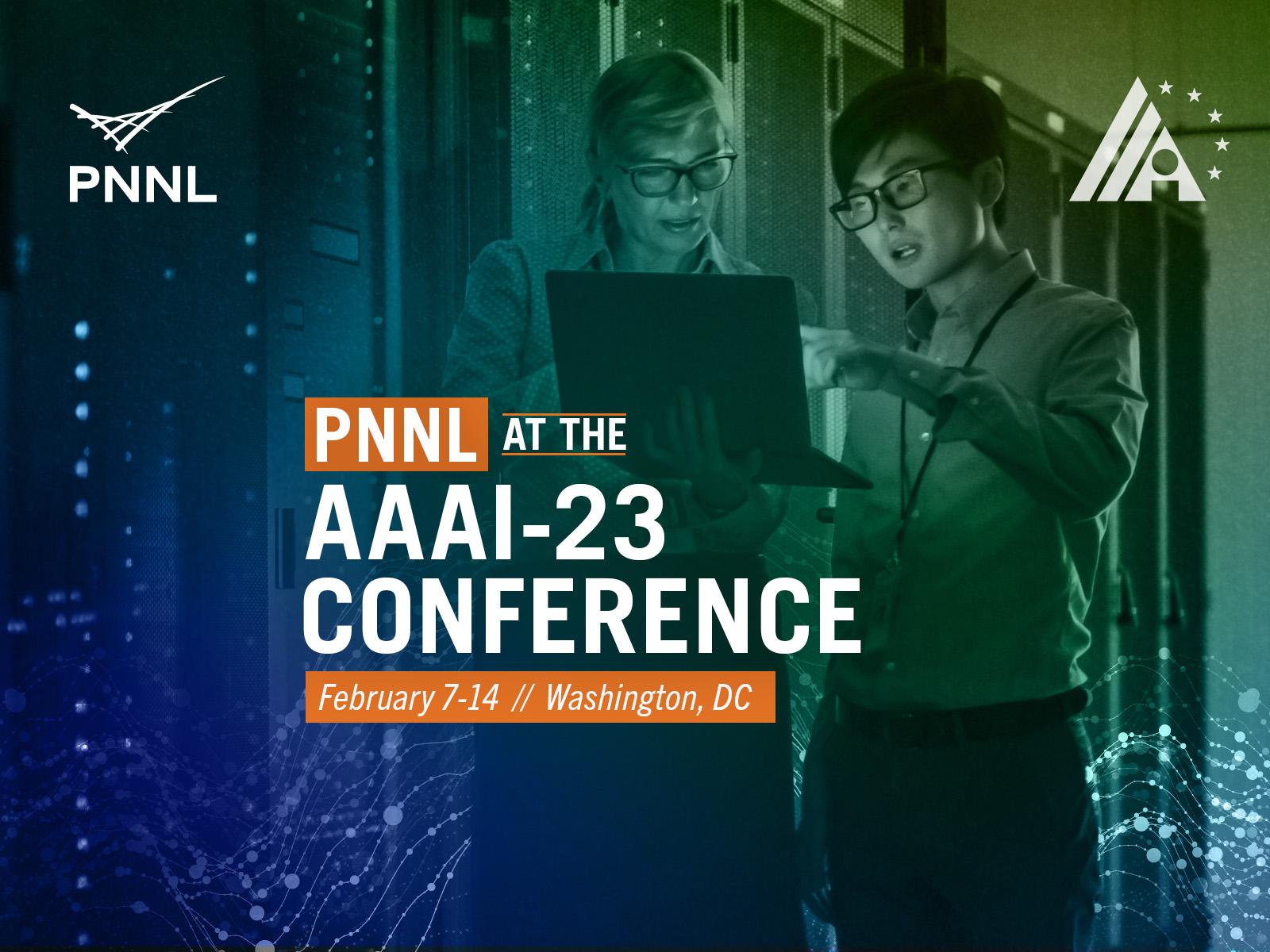
Image by Melanie Hess-Robinson
Washington, DC
PNNL will be at the 37th AAAI Conference on Artificial Intelligence on Feb. 7- 14 in Washington, DC.
Scientists and engineers at PNNL draw on signature capabilities in chemistry, Earth sciences, and data analytics to advance scientific discovery and create solutions to the nation's toughest challenges in energy resilience and national security. We’re looking for motivated candidates in the areas of data science, high-performance computing, cybersecurity, software engineering, and computational mathematics and statistics to join our teams.
PNNL Presentations, Papers, and Workshops
Experimental Observations of the Topology of Convolutional Neural Network Activations
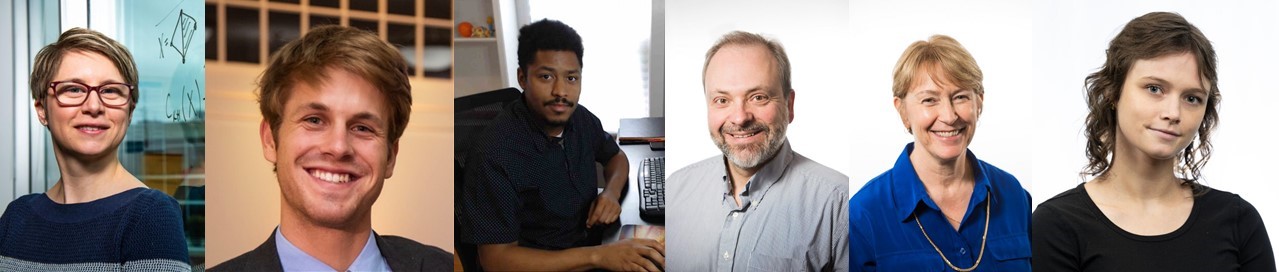
Accepted Paper
PNNL Authors: Emilie Purvine · Davis Brown · Brett Jefferson · Cliff Joslyn · Brenda Praggastis · Madelyn Shapiro
Abstract: Topological data analysis (TDA) is a branch of computational mathematics, bridging algebraic topology and data science, that provides compact, noise-robust representations of complex structures. Deep neural networks (DNNs) learn millions of parameters associated with a series of transformations defined by the model architecture resulting in high-dimensional, difficult to interpret internal representations of input data. As DNNs become more ubiquitous across multiple sectors of our society, there is increasing recognition that mathematical methods are needed to aid analysts, researchers, and practitioners in understanding and interpreting how these models' internal representations relate to the final classification.
In this paper we apply cutting edge techniques from TDA with the goal of gaining insight towards interpretability of convolutional neural networks used for image classification. We use two common TDA approaches to explore several methods for modeling hidden layer activations as high-dimensional point clouds, and provide experimental evidence that these point clouds capture valuable structural information about the model's process. First, we demonstrate that a distance metric based on persistent homology can be used to quantify meaningful differences between layers and discuss these distances in the broader context of existing representational similarity metrics for neural network interpretability. Second, we show that a mapper graph can provide semantic insight as to how these models organize hierarchical class knowledge at each layer. These observations demonstrate that TDA is a useful tool to help deep learning practitioners unlock the hidden structures of their models.
AutoNF: Automated Architecture Optimization of Normalizing Flows with Unconstrained Continuous Relaxation Admitting Optimal Discrete Solution
Accepted Paper

PNNL Author: Ján Drgoňa
Abstract: Normalizing flows (NF) are built upon invertible neural networks and have wide applications in probabilistic modeling. Currently, building a powerful yet computationally efficient flow model relies on empirical fine-tuning over a large design space. While introducing neural architecture search (NAS) to NF is desirable, the invertibility constraint of NF brings new challenges to existing NAS methods whose application is limited to unstructured neural networks. Developing efficient NAS methods specifically for NF remains an open problem. We present AutoNF, the first automated NF architectural optimization framework. First, we present a new mixture distribution formulation that allows efficient differentiable architecture search of flow models without violating the invertibility constraint. Second, under the new formulation, we convert the original NP-hard combinatorial NF architectural optimization problem to an unconstrained continuous relaxation admitting the discrete optimal architectural solution, circumventing the loss of optimality due to binarization in architectural optimization. We evaluated AutoNF with various density estimation datasets and show its superior performance-cost trade-offs over a set of existing hand-crafted baselines.
Deep Reinforcement Learning for Cyber System Defense under Dynamic Adversarial Uncertainties
Accepted Paper
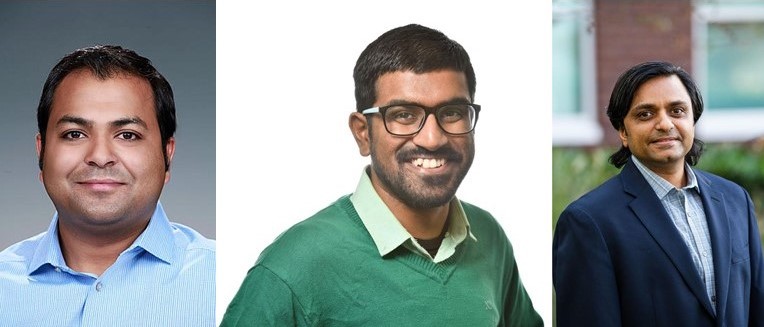
PNNL Authors: Samrat Chatterjee · Arnab Bhattacharya · Mahantesh Halappanavar
Abstract: Development of autonomous cyber system defense strategies and action recommendations in the real-world is challenging, and includes characterizing system state uncertainties and attack-defense dynamics. We propose a data-driven deep reinforcement learning (DRL) framework to learn proactive, context-aware, defense countermeasures that dynamically adapt to evolving adversarial behaviors while minimizing loss of cyber system operations. A dynamic defense optimization problem is formulated with multiple protective postures against different types of adversaries with varying levels of skill and persistence. A custom simulation environment was developed and experiments were devised to systematically evaluate the performance of four model-free DRL algorithms against realistic, multi-stage attack sequences. Our results suggest the efficacy of DRL algorithms for proactive cyber defense under multi-stage attack profiles and system uncertainties.
Training Machine Learning Models to Characterize Temporal Evolution of Disadvantaged Communities
Poster Presentation

PNNL Authors: Milan Jain · Narmadha Mohankumar · Heng Wan · Sumitrra Ganguli · Kyle Wilson · David Anderson
Abstract: Disadvantaged communities (DAC), as defined by the Justice40 initiative of the Department of Energy (DOE) identifies census tracts across the United States to determine where benefits of climate and energy investments are currently being accrued or not. The DAC status not only helps in determining the eligibility for future Justice40-related investments but is also critical for exploring ways to achieve equitable distribution of resources. However, designing inclusive and equitable strategies not only require a good understanding of current demographics, but also a deeper analysis of the transformations that happened in those demographics over the years. In this study, machine learning (ML) models were trained on publicly available census data from recent years to classify the DAC status at the census tracts level and use the trained model to classify DAC status for the historical years. A detailed analysis of the feature and model selection along with the evolution of disadvantaged communities between 2013 and 2018 is presented in this study.
Workshop (W4): AI for Energy Innovation
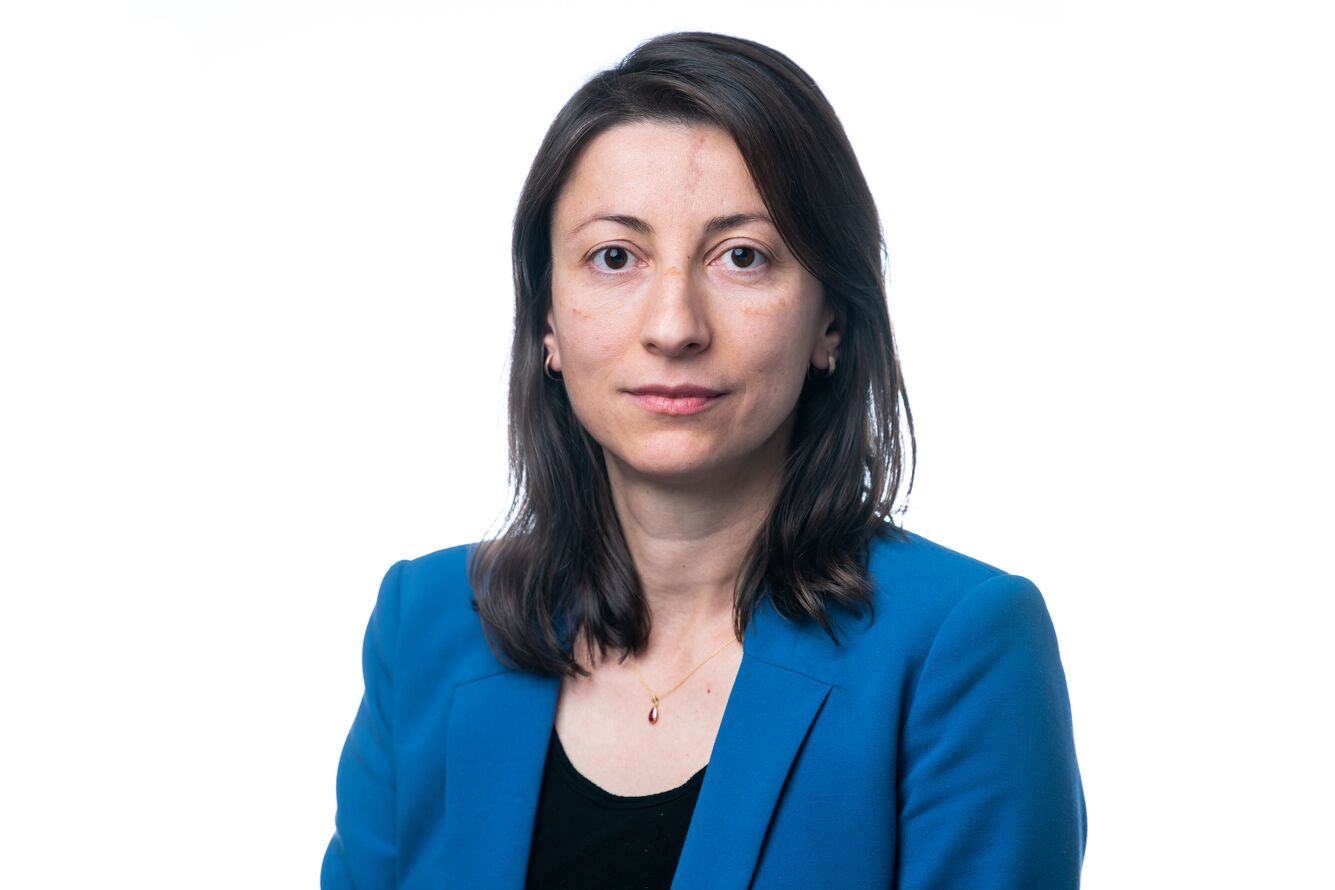
PNNL Organizer: Draguna Vrabie
Workshop overview: The Association for the Advancement of Artificial Intelligence (AAAI)-23 workshop AI for Energy Innovation invites attendees, researchers, practitioners, sponsors, and vendors from academia, government agencies, and the industry to present diverse views and engage in meaningful conversations on how innovation in all aspects of artificial intelligence (AI) may support and propel energy innovation. We strongly encourage dialogue-provoking contributions that summarize broader ongoing themes and efforts as well as upcoming and/or future opportunities that may stimulate a productive exchange and forge partnerships among participants. At the end of their talk, participants will be encouraged to propose a new energy-related benchmark problem that they would like the AI community to adopt, recognizing that well-known general datasets and problems may be suitable for general AI/ML education and research, but possibly not ideal nor focused-enough vehicles to propel AI-equipped, energy-focused innovations.
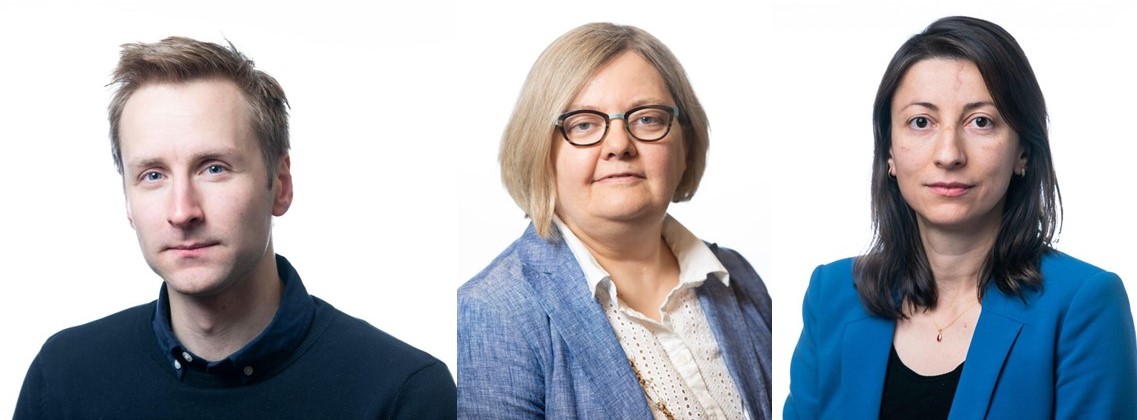
PNNL speakers: Ján Drgoňa (Differentiable Programming for Modeling and Control of Energy Systems), Sonja Glavaski (The Intersection of AI and Control: The Key to Addressing Energy Systems Challenges), Draguna Vrabie (AI Activities and Opportunities at Pacific Northwest National Laboratory)