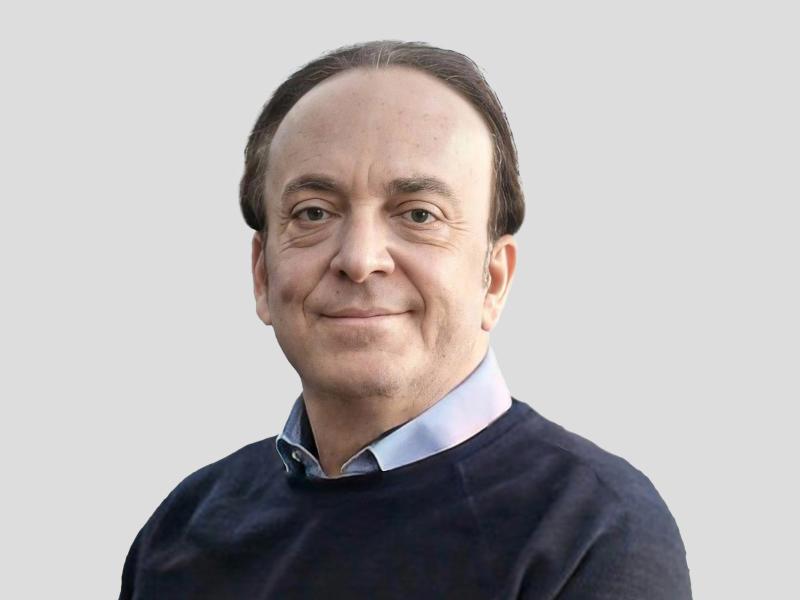
Data Science &
Computing
Data Science &
Computing
Extracting value from
a torrent of data
Extracting value from
a torrent of data
Pacific Northwest National Laboratory is addressing computationally focused problems in fundamental science, energy, environment, and national security. Computational scientist Kasia Swirydowicz and her colleagues study how to apply auto learning and reasoning to enhance scientific discovery.
Andrea Starr | Pacific Northwest National Laboratory
Data are everywhere in today’s world. From the phones in our hands, to the networks that run our homes and communities, to the scientific instrumentation that helps us discover the mysteries of the world—the deluge of data is staggering. This size, velocity, and complexity often make it difficult to gain clarity and extract value.
Pacific Northwest National Laboratory’s (PNNL's) computing research—from basic to applied—encompasses data and computational engineering, high-performance computing, applied mathematics, and semantic and human language technologies. Our scientists and engineers are developing and applying advanced theories, methods, and models to domains in machine learning, data and computing architectures, systems integration, and software and application development.
By combining engineering and operations domain expertise and creative integration of advanced hardware and software, we deliver advanced computational solutions that address complex data and analytic challenges. Working in multidisciplinary teams, we connect research to engineering to operations, providing the tools necessary to innovate quickly and field results faster. Our strengths are integrated across the data analytics lifecycle—from data acquisition and management, to analysis, to decision support.
With a strong focus on collaboration, PNNL is a partner in the Exascale Computing Project, a collaborative effort of the U.S. Department of Energy’s (DOE’s) Office of Science and the National Nuclear Security Administration designed to meet DOE’s national security and scientific needs. We are also part of the Physics-Informed Learning Machines for Multiscale and Multiphysics Problems Center—or PhILMs. Through this collaboration, scientists are developing physics-informed learning machines by encoding physics knowledge into deep learning networks.
Our computing research approaches are rooted in a scientific perspective founded on basic and applied research and analysis. We partner with government agencies and industry, performing research and development to deliver solutions to protect the nation’s critical strategic assets and address complex scientific challenges affecting energy, biological sciences, the environment, and national security.