Machine Reasoning for More Intelligent Microscopy
PNNL researchers design an artificial-intelligence-guided electron microscope
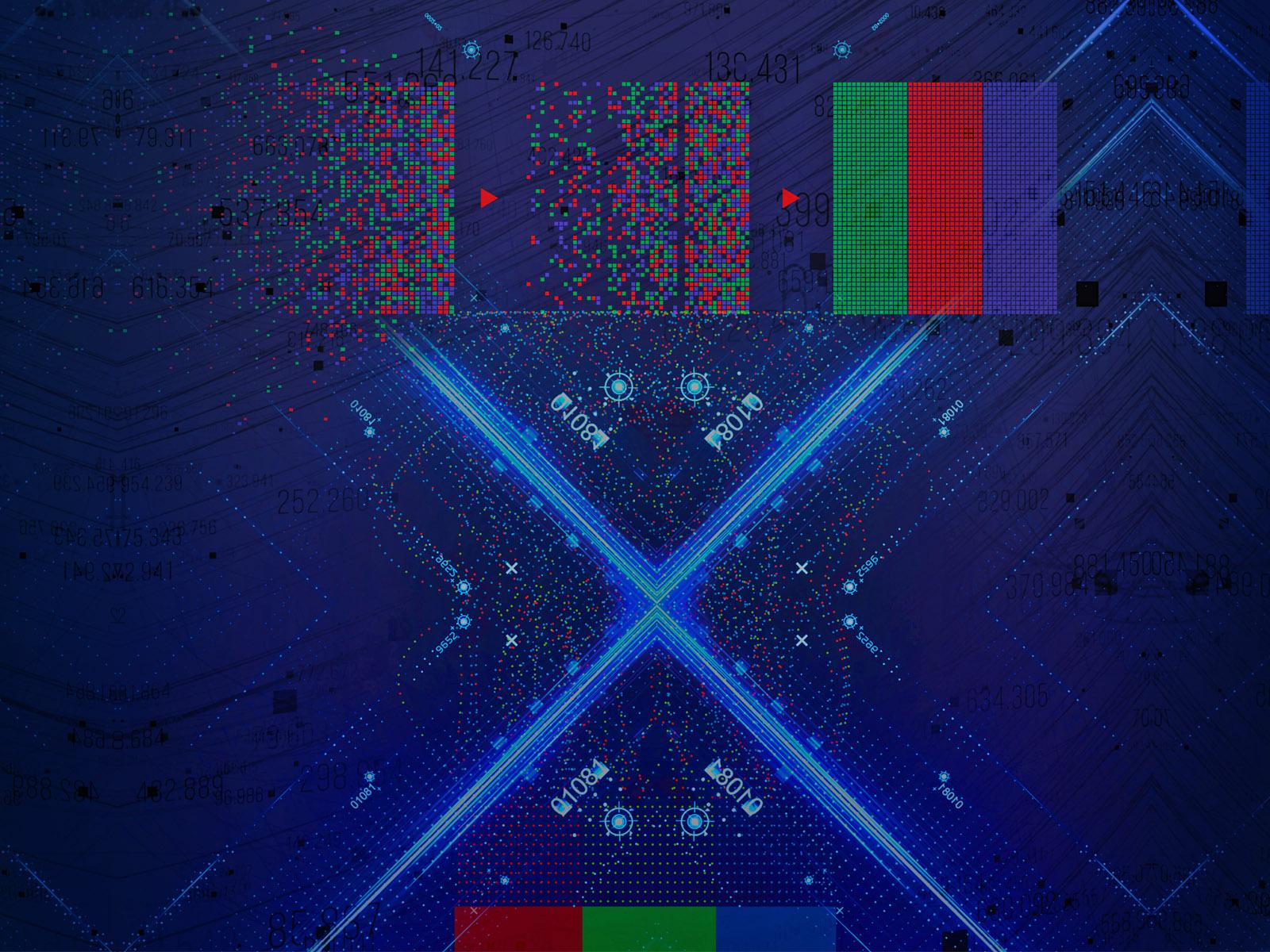
Researchers leveraged machine learning algorithms to automate data collection and analysis in microscopy.
(Composite image by Timothy Holland | Pacific Northwest National Laboratory)
The Science
Machine learning (ML) and automation have begun to transform data collection and analysis across many scientific disciplines. However, these techniques have not been widely used in imaging and spectroscopy due to proprietary instrumentation and lack of generalizable approaches to machine reasoning. Now, researchers from Pacific Northwest National Laboratory (PNNL) and Washington State University Tri-Cities have developed a way to automate electron microscopy experimentation in a variety of scenarios using emerging sparse data analytics. Using just a few examples, their ML algorithm can quickly detect patterns that denote important chemical and materials processes. The instrument can then automatically respond to and target features in an automated manner, providing unprecedented insight into materials for emerging technologies.
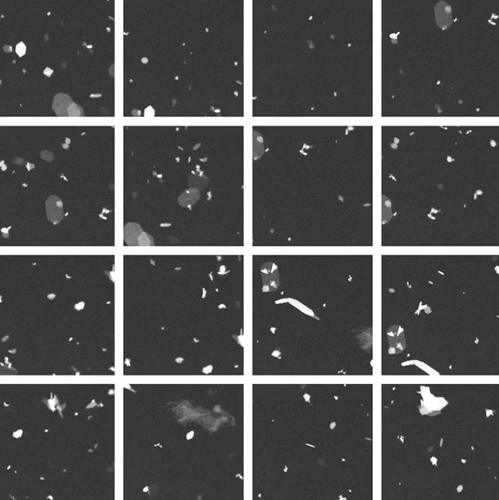
The Impact
Scientists use a variety of imaging and spectroscopic instruments to examine chemical and materials systems. However, these techniques produce massive amounts of data that scientists struggle to analyze and act on manually. The system developed by PNNL researchers will help expedite scientific discovery by automating data collection and analysis. Automated approaches will help standardize microscopy experiments, increase repeatability, and improve throughput to uncover key drivers of important energy and quantum information technologies. This approach also represents a new way to train a machine using expert knowledge and will open the door to a future of artificial-intelligence-guided experimentation.
Summary
The ML algorithm devised by the PNNL team can identify important features in microscope images based on a minimal amount of training. In brief, a user provides examples of what they care about, and a neural network is trained to find those features in the data. This approach works well for many different types of image data, ranging from the atomic to the micron scale, all without specialized tuning. This approach opens the door to a new kind of machine-guided analysis and automation of scientific experiments, which will transform the way materials and chemical systems are designed for energy storage and computing technologies.
The team has implemented their machine reasoning using a completely new instrument controller and intuitive graphical user interface. As corresponding author and PNNL materials scientist Steven Spurgeon explains, “This platform represents a unique approach to truly programmable experimentation in the electron microscope. Machine reasoning, decision-making, and low-level control are all conducted in real-time, equipping scientists with the ability to richly probe complex phenomena like never before.” Moving forward, the team is exploring commercialization of these technologies together with Japan Electron Optics Laboratory/Integrated Dynamic Electron Solutions (JEOL/IDES), a world leader in electron microscopy.
The system was constructed in the Radiological Microscopy Suite (RMS), located in the Radiochemical Processing Laboratory (RPL) at PNNL. Some sample preparation was performed at the Environmental Molecular Sciences Laboratory (EMSL), a national scientific user facility sponsored by the Department of Energy’s Biological and Environmental Research program and located at PNNL.
Contact
Steven R. Spurgeon
Pacific Northwest National Laboratory
steven.spurgeon@pnnl.gov, (509) 371-7709
Funding
This research was supported by the I3T Commercialization Laboratory Directed Research and Development (LDRD) program at PNNL. The few-shot ML code development was also supported by the Chemical Dynamics Initiative (CDi) LDRD program, with some initial code development performed under the Nuclear Process Science Initiative (NPSI) LDRD program.
Related links
https://www.pnnl.gov/news-media/enabling-data-driven-future-microscopy
https://www.pnnl.gov/news-media/electron-microscopy-age-automation
Published: June 28, 2022
Matthew Olszta, et al. An Automated Scanning Transmission Electron Microscope Guided by Sparse Data Analytics. Microscopy and Microanalysis (2022), 1–11. doi:10.1017/S1431927622012065