PNNL, PassiveLogic Join Forces to Improve Buildings
Two research organizations apply capabilities to shape new technology
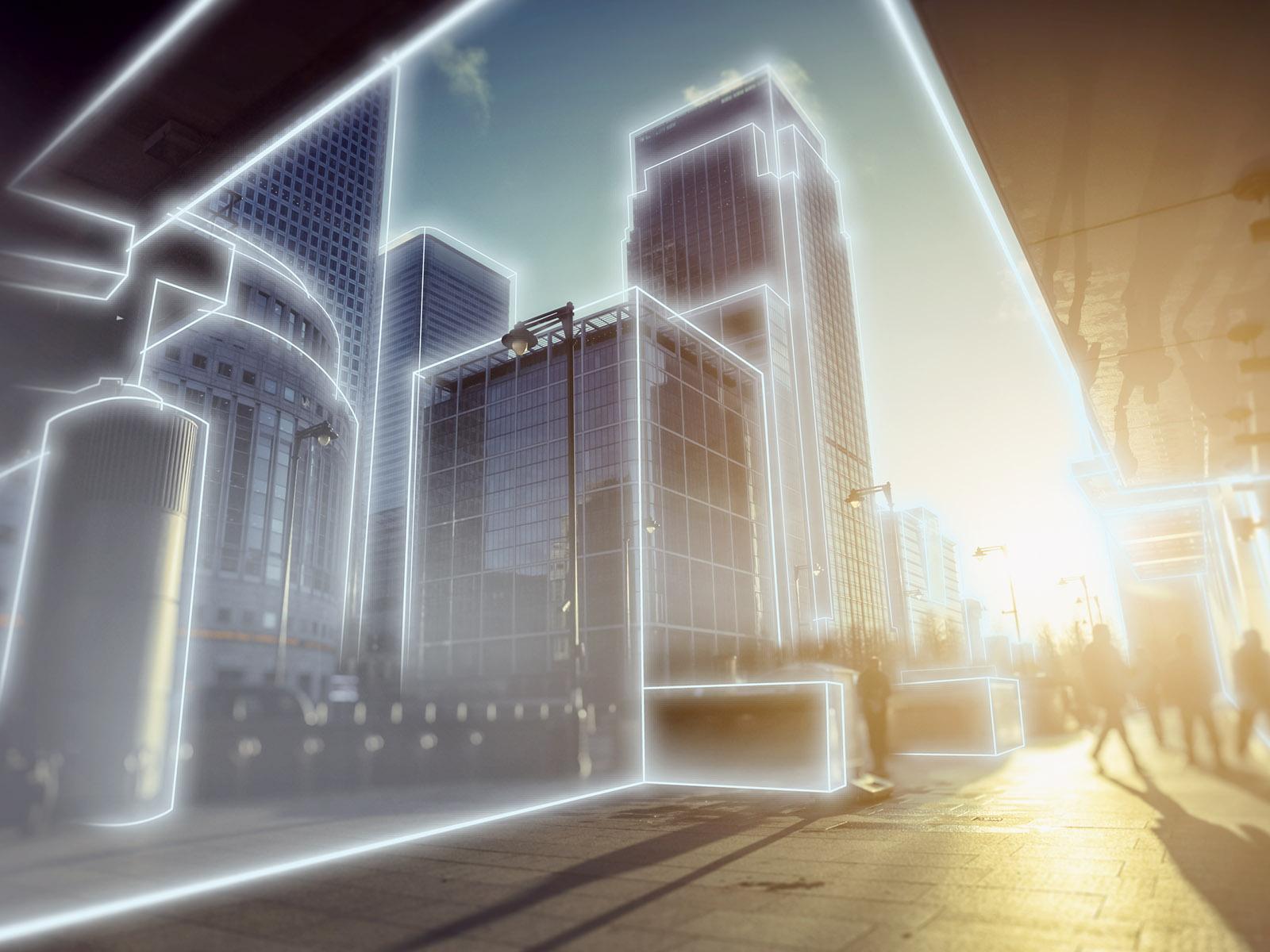
Collaboration between PNNL and PassiveLogic is expected to lead to a technology solution that will benefit buildings and the environment.
(Image: PassiveLogic)
Pacific Northwest National Laboratory (PNNL) and PassiveLogic, a Utah firm, are combining their research and development capabilities to help commercial and other buildings operate more effectively and deliver a range of benefits, including energy savings and reduced carbon emissions.
The two organizations this year signed a Cooperative Research and Development Agreement (CRADA) supported by funding from the Department of Energy’s (DOE's) Building Technologies Office (BTO). CRADAs have long been a DOE tool for encouraging public-private collaborations and advancing technologies to the marketplace.
The two-year agreement will enable PNNL and PassiveLogic to jointly develop and apply their capabilities. They’ll foster a technology that will help building energy systems, such as heating and cooling units and lighting, become fully autonomous, capable of identifying and addressing their own operational issues, and able to self-optimize their operation.
“When we met the PassiveLogic team more than a year ago, we learned that they share a similar vision and we were eager to work with them. Then, an opportunity to write a CRADA proposal emerged,” said Draguna Vrabie, a data scientist who leads PNNL’s Autonomous Learning and Reasoning Group. “BTO selected the CRADA proposal for funding, and we’re now very excited to work with PassiveLogic to collaborate and share our respective ideas and combine our capabilities.”
Maximizing benefits in buildings
Some of today’s larger commercial buildings contain a building automation system (BAS) designed to manage and coordinate the operation of building functions, such as heating and cooling, water heating, and lights. BASs are becoming even more prominent tools as building owners and managers, utilities, states, and communities look for ways to increase energy efficiency, use clean energy in buildings, improve occupant comfort, and reduce carbon emissions and costs.
PassiveLogic has been pursuing an advanced integrated hardware and software platform. The platform incorporates deep learning methods to create a new and innovative evolution of the traditional BAS, capable of supporting the entire building life cycle—from design to control and maintenance—and operable from user software tools the company has also developed.
Concurrently and independently, PNNL has developed a novel framework that leverages differentiable programming to achieve cost-effective predictive control. This method provides a practical means to optimize building operations without the computational overhead of traditional predictive approaches.
The CRADA will help improve and integrate the work of both organizations, accelerating release of a market-ready product designed for use in a broad range of buildings, including those small and medium commercial buildings that currently do not have BASs.
Advancing PNNL’s framework
While the details on the CRADA were being worked out, PNNL continued to develop its framework, which has become more mature. PassiveLogic also continued development and commercialization of its technology.
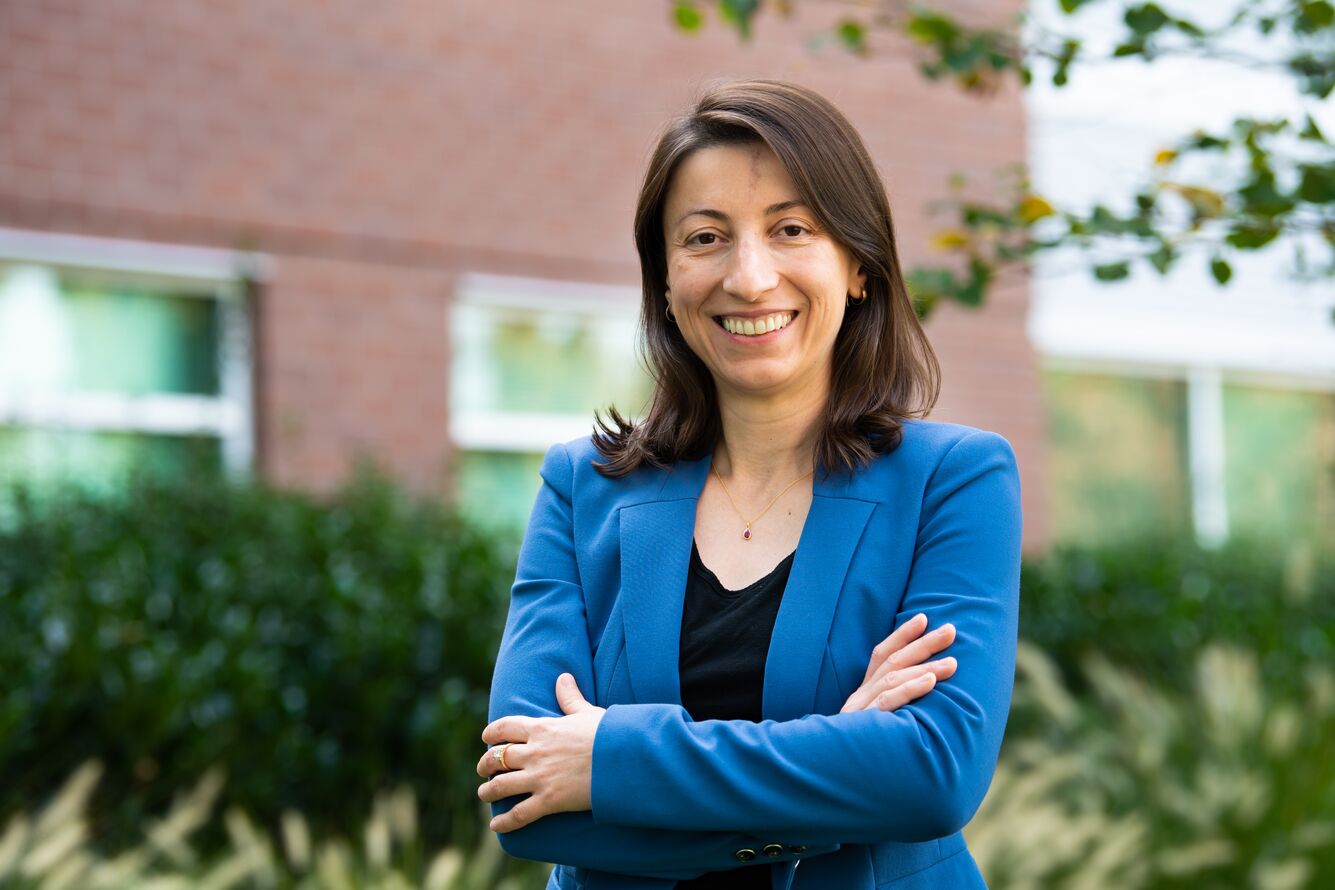
“We’re keen to start this collaboration with PassiveLogic and effectively integrate our advanced methods into industry practice,” Vrabie explained. “PassiveLogic offers outstanding laboratory capabilities, along with systems in real, occupied buildings that will serve as testbeds to verify the energy performance.”
Vrabie noted that one of the exciting and novel aspects of PNNL’s framework is that it’s not specific to buildings. “With technical leadership and a strong partnership of a team of computer scientists, data scientists, and domain experts led by Jan Drgona and Aaron Tuor, PNNL’s differentiable programming framework is broadly applicable to prediction and optimal decision-making in domains ranging from basic energy sciences to complex engineered energy systems,” she said. “We’re very optimistic about the future of this capability and its applications to the whole range of engineering and scientific research.”