Co-Designing the Future of Computational Chemistry
New research informs the development of foundational models through co-design
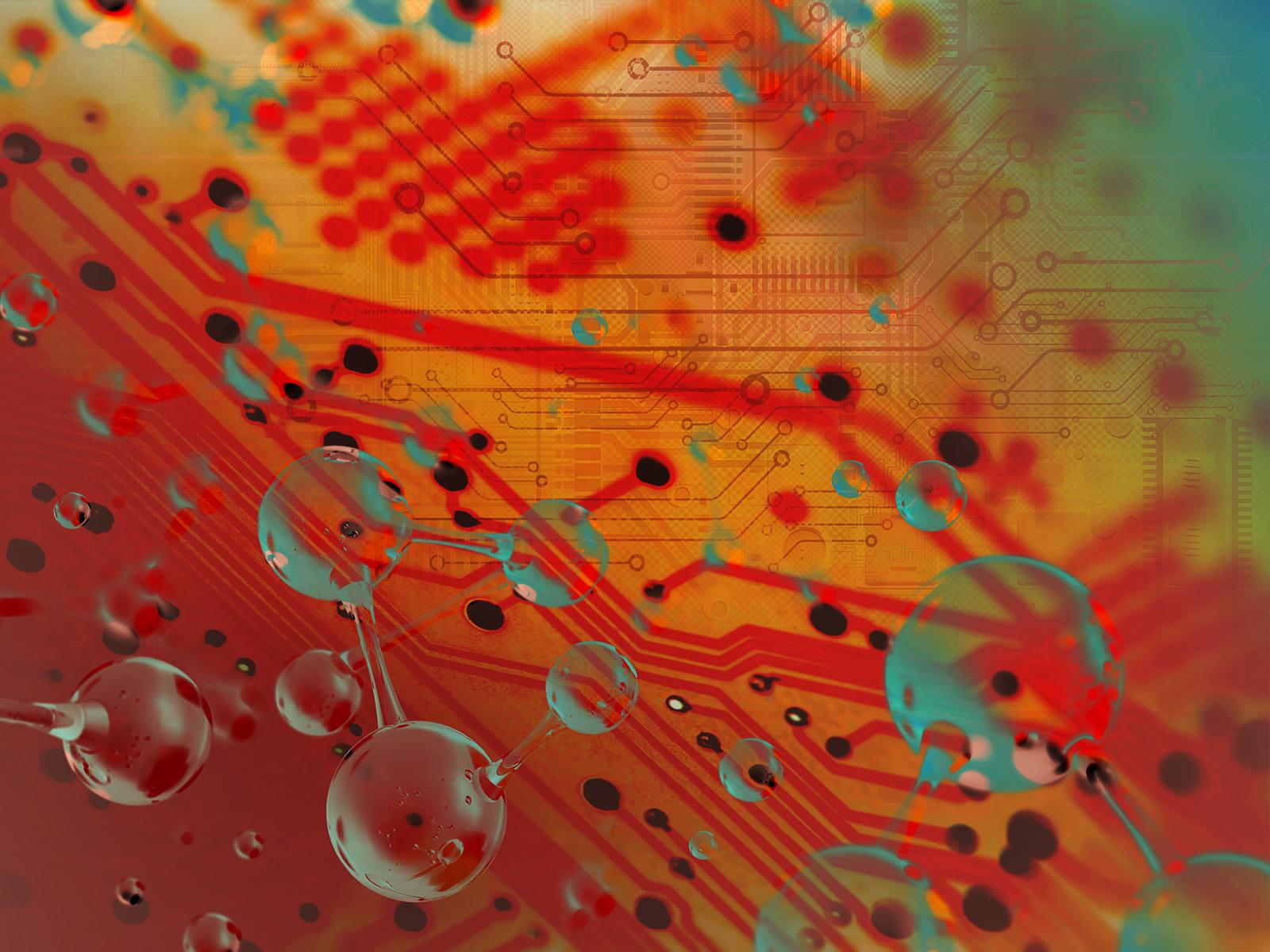
Researchers developed a set of optimization techniques for graph neural networks on intelligence processing units.
(Composite image by Donald Jorgensen | Pacific Northwest National Laboratory)
In the field of computational chemistry, researchers use computers to calculate and predict the properties of chemicals and materials. Modeling these properties accurately can be expensive in terms of computational time and energy.
Machine learning techniques can help make these predictions more efficient. Researchers from Pacific Northwest National Laboratory (PNNL), the University of Washington (UW), Graphcore, and IBM Research developed a faster way to train machine learning models through hardware–software co-design. Their research was published in the Journal of Chemical Theory and Computation.
“In chemical calculations, the geometries of atoms are represented as graphs,” said Sutanay Choudhury, who is a Chief Scientist of data sciences at PNNL. “Thus, we focused on a type of machine learning called graph neural networks—GNNs—for chemical structure and property prediction and optimized their training.”
Using Graphcore’s Intelligence Processing Unit (IPU)—a processor designed for artificial intelligence-based workloads, the researchers developed a new hardware–software co-design approach to scale up the training of GNN-based models.
They first built a set of optimization techniques for execution of the GNNs. These techniques take advantage of the high-bandwidth local memory and fast interconnect of the Graphcore system architecture to improve GNN training time. The researchers also built these techniques in a way that allows the IPU-trained atomistic GNNs to support transfer learning of custom models to graphics processing units (GPUs), and central processing units (CPUs).
“After pre-training our GNNs on IPUs, we found that we could fine-tune the GNN on a single GPU with significantly less data than we could without pre-training,” said Choudhury. “This means a pre-trained model could allow for more research at a lower computational cost—setting the stage for potential atomistic foundational models in the future.”
Developing new molecular modeling capabilities such as these are one of the goals of the Computational and Theoretical Chemistry Institute (CTCI) at PNNL, where Choudhury serves as Deputy Director. CTCI Director Sotiris Xantheas co-authored the paper with Choudhury.
“The CTCI supports the advancement of computational chemistry through close collaborations between domain scientists, data scientists, and computer scientists,” said Xantheas. “From the development of new software for electronic structure calculations to the incorporation of physics-informed machine learning techniques, we formulate new solutions for scientific problems on a variety of leadership computing facility architectures.”
PNNL researchers Jesun Firoz, Jenna (Bilbrey) Pope, Henry Sprueill, and Ang Li also contributed to the research paper. In addition to his primary appointment at PNNL, Xantheas is also an affiliate professor at the University of Washington.
This work was partially supported by the ExaLearn Co-design Center within the Exascale Computing Project, a collaborative effort between the Department of Energy’s (DOE’s) Office of Science (SC) and the National Nuclear Security Administration. It was also supported from the Center for Scalable Predictive Methods for Excitations and Correlated Phenomena (SPEC), which is funded by the U.S. Department of Energy, Office of Science, Basic Energy Sciences, Chemical Sciences, Geosciences and Biosciences Division, as part of the Computational Chemical Sciences (CCS) program under FWP 70942 at Pacific Northwest National Laboratory (PNNL), a multiprogram national laboratory operated by Battelle for the U.S. Department of Energy. This research was partially supported by the DOE-SC, Advanced Scientific Computing Research program, under CENATE – the Center for Advanced Technology Evaluation. Additionally, PNNL is advancing work in AI technology through its Center for AI.
Published: April 25, 2024