Soil Microbiome Research
PNNL’s Soil Microbiome Metaphenome Science Focus Area aims to achieve a systems-level understanding of the soil microbiome’s phenotypic response to changing moisture. We perform multi-scale examinations of molecular and ecological interactions occurring within and between members of microbial consortia during soil organic matter decomposition, using chitin as a model compound.
Integrated experiments are designed to confront spatial challenges and inter-kingdom interactions among bacteria, fungi, viruses and plants that regulate community functions. In turn, these data are used to parametrize individual- and population-based models for predicting interspecies and inter-kingdom interactions.

Predictions are tested in lab and field experiments to reveal individual and community microbial phenotypes. In the lab, reduced complexity model microbial communities provide details underpinning microbial interspecies and interkingdom interactions in response to moisture and substrate stress.
At the field level, multi-omics provides metabolic maps that are predictions of carbon use efficiency (CUE). We define metabolic phenotypes at the community scale as the metaphenome: metagenome (MG) x environmental resources (E) = metaphenome (MP). Information gained about metabolic interactions from genomes and metagenomes enable predictions of MP and thus the metabolic capacity of the community. Expression data from multi-omics add information about activities that result in measured phenotypes.
Knowledge gained will provide fundamental understanding of how soil microbes interact to decompose organic substrates and enable prediction of how biochemical reaction networks shift in response to changing moisture regimes.
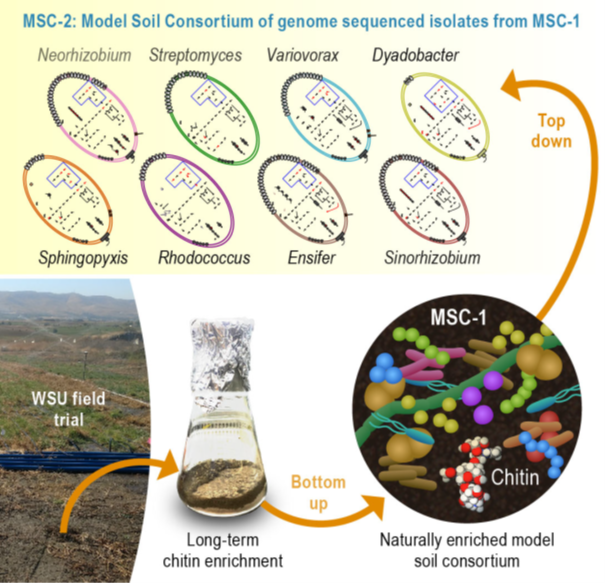
Thrust 1 – Tractable Metaphenomes
How do organismal phenotypes influence community interactions to generate metaphenomes?
We will investigate metabolic and physiological responses among bacteria, fungi, and viruses through empirical experiments and new predictive modeling approaches.
In this figure: Model soil consortia are a resource developed by the SFA to enable study of interspecies interactions between soil microorganisms during decomposition of chitin, a model soil substrate. Left panel shows the bottom-up strategy used to develop MSC-1, a naturally enriched and reduced complexity consortium with 35 microbial members and the top-down strategy used to develop MSC-2, a defined consortium of eight members that have been genome sequenced.
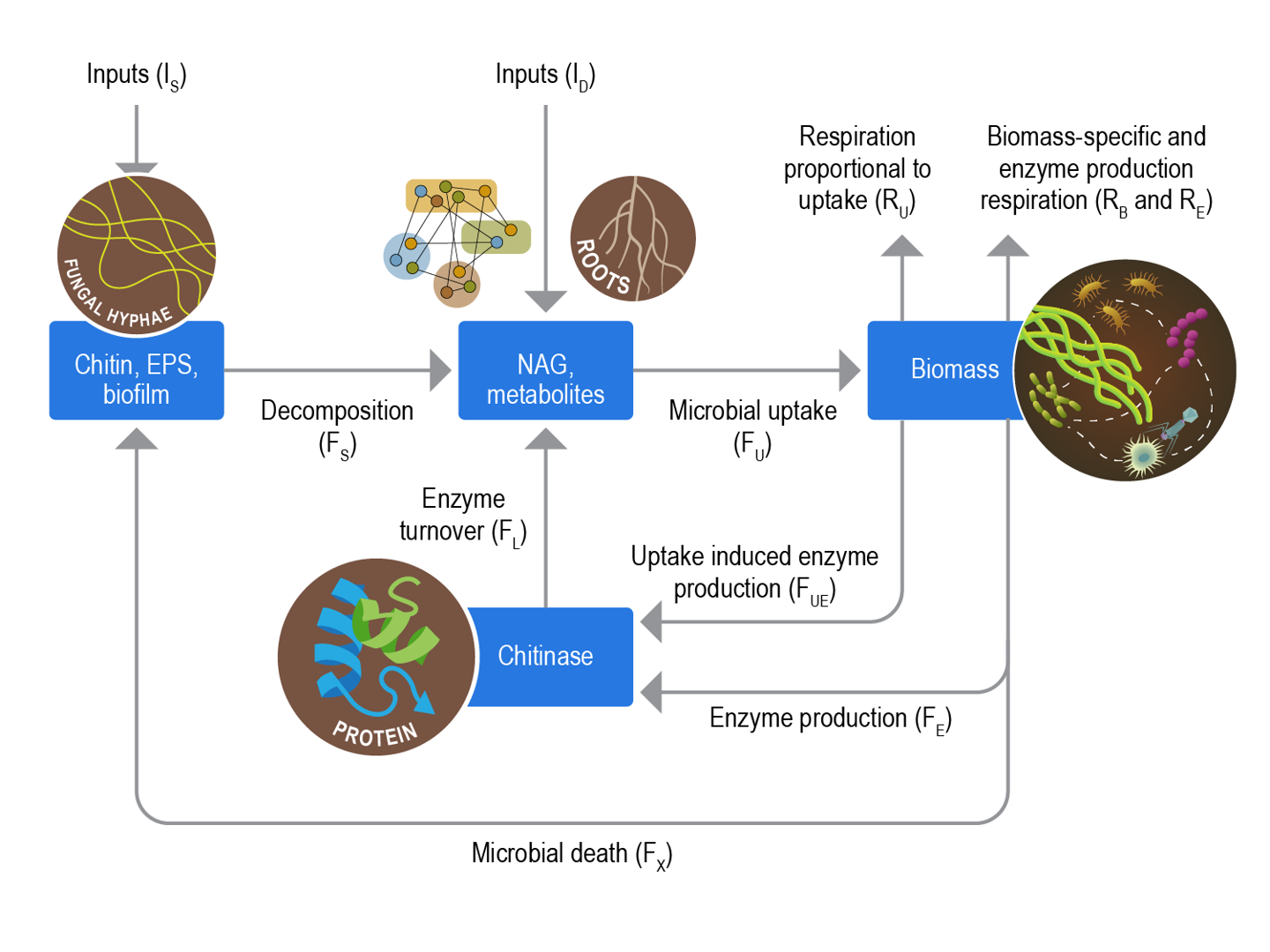
Thrust 2 – Intermediate Complexity Metaphenomes
How do metaphenomes change in response to environmental conditions?
We will test the effects of moisture and microbial connectivity on community interactions and resultant metaphenomes using a suite of model-informed laboratory experiments with increasing microbial and habitat complexity.
In this figure: Carbon use efficiency (CUE) is investigated from a biogeochemical and molecular perspective concurrently to better understand how carbon is allocated among pathways required for microbial survival. Here we use chitin decomposition as an example to examine both bulk and molecular pools and fluxes that may influence CUE for soil microbial communities.
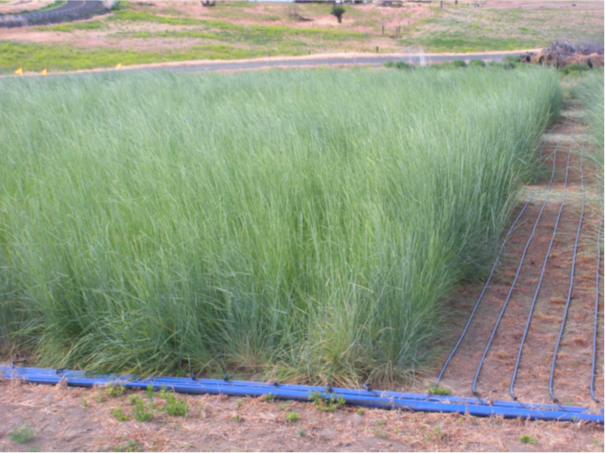
Thrust 3 – Field Metaphenomes
Do reduced complexity metaphenomes accurately identify the microbial mechanisms underlying metagenomic expression in the field?
Thrust 3 incorporates our vast amount of multi-omic data developed in the first triennial. We will test if key processes governing microbial CUE are shared between lab and field scales and if universal relationships are definable based on scale- independent dimensionless parameters.
In this figure: Irrigation field trials at the WSU field station in Prosser, WA (with blue driplines) included four levels of irrigation (25%, 50%, 75% and 100% of normal); 2 varieties of tall wheatgrass (Alkar and Jose); and an unplanted control. Bulk soil samples were taken monthly before and after planting, and rhizosphere samples were taken during peak growing season.
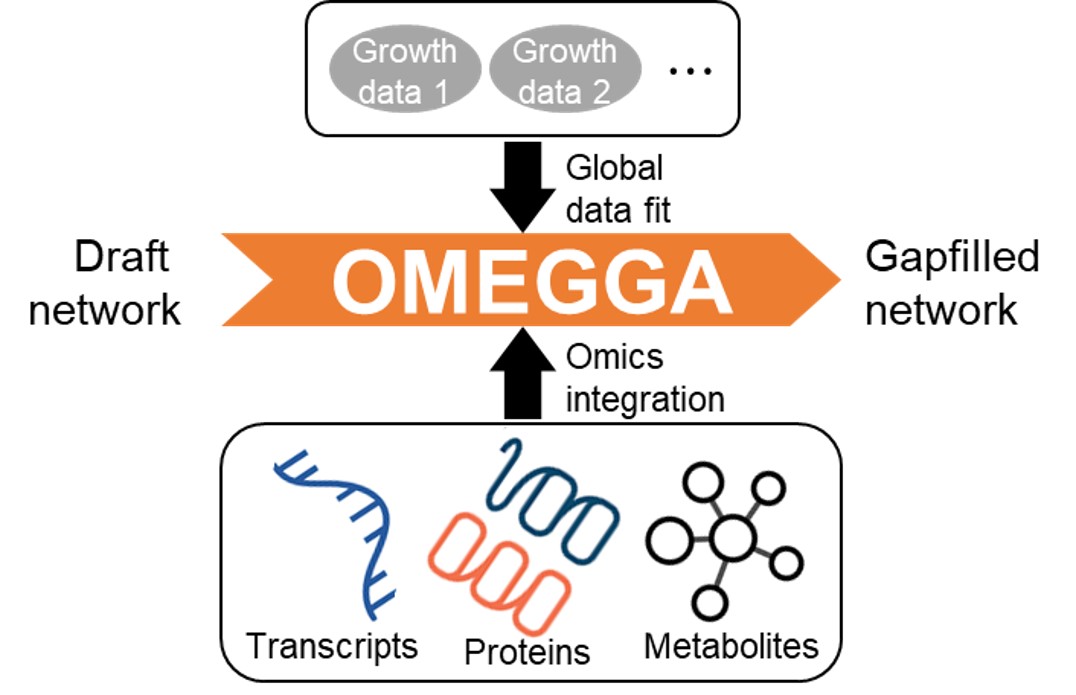
OMEGGA
PNNL and UNL are working with the KBase team to develop an advanced gapfilling optimization algorithm (termed OMics-Enabled Global GApfilling or OMEGGA) that uses multi-omics data to simultaneously fit a draft metabolic model to a given set of phenotype data. The novel integration of amplicon, transcript, protein, and metabolite data into model refinement will yield more precise and predictive metabolic models and increase the accuracy of identification of active reactions. False predictions are a greater problem in modeling communities, compared to isolates, due to the substantially larger pool of reactions available as options for gapfilling.
The effectiveness of OMEGGA is being tested and demonstrated using benchmark data for non-model organisms as well as commonly studied bacterial species. The valuable data for non-model organisms is sourced from the Soil Microbiome SFA Model Soil Consortia (MSC) and associated isolated organisms. Therefore, the goals of the Soil Microbiome SFA will directly benefit from improvements in the metabolic modeling refinement through OMEGGA. Implementation of OMEGGA into existing KBase apps along with testing and documentation is planned to benefit the larger scientific community as well.