ESMI Publications
ESMI Publications
PNNL’s ESMI project team generates a variety of project documents, research papers, articles, reports, presentations, and other resources to share with researchers, industry stakeholders, and other interested parties.
Publication Highlights
ESMI researchers use machine learning to predict solubility of molecules for better flow battery chemistries
ESMI’s researchers are using machine learning to predict how well certain molecules dissolve in water. By making these material property predictions quickly and more accurately, ESMI seeks to reduce time-consuming experimental testing and accelerate the design of chemistries for better performing redox flow batteries. To do that, an ESMI research team is addressing some of the challenges associated with developing solubility prediction models, such as the lack of large training datasets containing molecular property measurements and the insufficient solubility prediction accuracy of current deep learning methods.
To address the first challenge, they used natural language processing tools to extract molecular measurement data from scientific literature using material solubility as the target property. The researchers created training and evaluation datasets containing tags for solubility-related words using a combination of regular expressions and manual tagging, compared five entity recognition models, and explored a novel pretraining approach by generating large datasets without the need for intensive manual tagging. As a final step the team performed an analysis to identify the causes of classification errors. This work was published and presented at the 2021 Conference on Empirical Methods of Natural Language Processing hosted by the Association of Computational Linguistics.
Additionally, the team wanted to understand how well current deep learning methods can predict solubility and what types of information about the molecules are most helpful to support accurate predictions. To address these questions, they tested four different deep learning solubility prediction methods which each leveraged a different representation of the molecular structure. They found that that the best models are those that use molecular descriptors or graph-based representations of the molecular structure. As part of this work, they also studied how much data is needed to make accurate predictions and whether data on other molecular properties can be used to improve solubility prediction. In general, more work needs to be done to create better, more accurate models as current methods have a hard time predicting the impact of small structural differences in the molecules on the resulting solubility. This work was published in ACS Omega by the American Chemical Society in August of 2022.
Now that the team has looked at predicting properties from molecular structure, they want to do the inverse task, which is to use machine learning to automatically generate novel molecular structures given certain target properties as input, such as solubility or electrical conductivity.
The research team focused on machine learning includes Emily Saldanha, Gihan Panapitiya, Tim Yin, Peiyuan Gao, and Jessica Kong.
ESMI researchers introduce promising physics-informed machine learning models for predicting performance of Redox Flow Batteries
Fast and accurate models could prove key to unlocking the next generation of redox flow batteries (RFBs) for storing large amounts of energy for the grid. Researchers with the Energy Storage Materials Initiative (ESMI) are working towards creating new models using physics-informed machine learning (PIML) algorithms to better predict how a battery will perform under certain conditions. Better models could lead to improved battery chemistries and designs.
An ESMI research team, led by Amanda Howard, developed a promising Multifidelity Physics-Informed Cokriging (CoPhIK) machine learning model for predicting the charge–discharge curve of a VRFB. This model only requires a small number of high-fidelity datasets (current density, flow rate, and initial concentrations) to make accurate predictions, making it an improvement over predictions with existing zero-dimensional models alone.
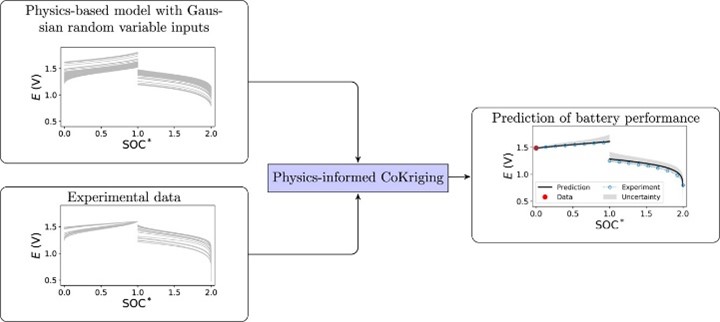
This new model was presented in a journal article published in the Journal of Power Sources, along with an article by ESMI researcher Yucheng Fu which explains how to use an enhanced PIML to identify parameters critical to the performance of a flow battery. Fu and his team introduce a physics-constrained deep neural network (ePCDNN) and demonstrate that it can accurately predict the cell voltage response of a battery throughout the charge-discharge cycle, even under extreme conditions. This can improve how well battery manufacturers and operators determine the optimal operation conditions to prevent hazards during battery operation.
Publications List
Published in 2024
- Tran, T.-N., X. Cao, Y. Xu, P. Gao, H. Zhou, F. Guo, K.S. Han, D. Liu, P.M.L. Le, J.M. Weller, M.H. Engelhard, C. Wang, M.S. Whittingham, W. Xu, J.-G. Zhang. 2024. “Enhancing Cycling Stability of Lithium Metal Batteries by a Bifunctional Fluorinated Ether,” Advanced Functional Materials. DOI: 10.1002/adfm.202407012.
- Howard, A.A., Y. Fu, P. Stinis. 2024. “A multifidelity approach to continual learning for physical systems.” Machine Learning: Science and Technology. 5(2): 25042. DOI: 10.1088/2632-2153/ad45b2.
- Fu Y., A.A. Howard, C. Zeng, Y. Chen, P. Gao, P. Stinis. 2024. "Physics-Guided Continual Learning for Predicting Emerging Aqueous Organic Redox Flow Battery Material Performance.” ACS Energy Letters. 9(6): 2767-2774. DOI: 10.1021/acsenergylett.4c00493.
- Noh, J., H.A. Doan, H. Job, L.A. Robertson, L. Zhang, R.S. Assary, K. Mueller, V. Murugesan, Y. Liang. 2024. “An Integrated High-throughput Robotic Platform and Active Learning Approach to Accelerated Discovery of Optimal Electrolyte Formulations.” Nature Communications. 15: 2757. DOI: 10.1038/s41467-024-47070-5.
- Lim, H.-S., C.V. Subban, D.T. Nguyen, T.S. Nasoetion, T. Liu, K. Han, B.M. Sivakumar, T. Lemmon, S. Kim, Z. Yu, A. Devaraj, M.J. Willey, W. Wang, V. Murugesan. 2024. “Room Temperature Electrorefining of Rare Earth Metals from End-of-Use Nd−Fe−B Magnets.” ACS Sustainable Resource Management. 1(2), 269-277. DOI: 10.1021/acssusresmgt.3c00062.
- Dean, W., M. Munoz, J. Noh, Y. Liang, W. Wang, B. Gurkan. 2023. Tuning and high throughput experimental screening of eutectic electrolytes with co-solvents for redox flow batteries. Electrochimica Acta. 474: 143517. DOI: 10.1016/j.electacta.2023.143517.
- Chen, W., Y. Chen, P. Stinis. 2023. “Physics-informed machine learning of redox flow battery based on a two-dimensional unit cell model.” Journal of Power Sources. 584, 233548. DOI: 10.1016/j.powsour.2023.233548..
- Yin, T., G.U. Panapitiya, E.D. Coda, E.G. Saldanha. 2023. “Evaluating uncertainty-based active learning for accelerating the generalization of molecular property prediction.” J. Cheminformatics. 15(1): 105. DOI: 10.1186/s13321-023-00753-5.
- Kumar, N., W. Rishko, K.R. Fiedler, A. Hollas, J. Chun, S.I. Johnson. 2023. “Correlations between Molecular Structure, Solvation Topology, and Transport Properties of Aqueous Organic Flow Battery Electrolyte Solutions.” ACS Mat. Lett. 5(11): 3050-3057. DOI: 10.1021/acsmaterialslett.3c00838.
- Fiedler, K.R., M.J. Olszta, K.H. Yano, C.M. Doty, D.F. Hopkins, S.M. Akers, S.R. Spurgeon. 2023. “Evaluating Stage Motion for Automated Electron Microscope.” Microscopy and Microanalysis. 29(6): 1931-1939. DOI: 10.1093/micmic/ozad108.
- Liang, Y., H.M. Job, R. Feng, F. Parks, A.M. Hollas, X. Zhang, M.E. Bowden, J. Noh, V. Murugesan, W. Wang. 2023. “High throughput solubility determination for data-driven materials design and discovery in redox flow battery research.” Cell Reports Physical Science. 54(10): 101633. DOI: 10.1016/j.xcrp.2023.101633.
Published in 2023
- Cho, J., K.-S. Kim, S. Kim, Y. Shao, Y.-T. Kim, S. Park. 2023. “Substrate-Driven Catalyst Reducibility for Oxygen Evolution and Its Effect on the Operation of Proton Exchange membrane Water Electrolyzers.” Small Structures. 5(1): 2300276. DOI: 10.1002/sstr.202300276.
- Liu, Y., R. Huang, W. Du, A. Singhal, Z. Huang. 2023. "Highly-Scalable Transmission and Distribution Co-simulation with 10,000+ Grid-Following and Grid-Forming Inverters,” IEEE Transactions on Power Delivery. 39: 578-590. DOI: 10.1109/TPWRD.2023.3302303.
- Liu, J., Z. Zhang, X. Li, M. Zong, Y. Wang, S. Wang, P. Chen, Z. Wan, L. Liu, Y. Liang, W. Wang, S. Wang, X. Guo, E.G. Saldanha, K.M. Rosso, X. Zhang. 2023. “Machine learning assisted phase and size-controlled synthesis of iron oxide particles.” Chem. Eng. J. 473: 145216. DOI: 10.1016/j.cej.2023.145216.
- Feng, R., Y. Chen, X. Zhang, B. Rousseau, P. Gao, P. Chen, S.T. Mergelsberg, L. Zhong, A. M. Hollas, Y. Liang, V. Murugesan, Q. Huang, S. Hammes-Schiffer, Y. Shao, W. Wang. 2023. “Proton regulated alcohol oxidation for high-capacity ketone-based flow-battery anolyte,” Joule. 7(7): 1609-1622. DOI: 10.1016/j.joule.2023.06.013.
- Belongia, S., X. Wang, X. Zhang. 2023. “Progresses and Perspectives of All-Iron Aqueous Redox Flow Batteries,” Advanced Functional Materials. 34( 5): 2302077. DOI: 10.1002/adfm.202302077.
- Chen, Y., J. Bao, Z. Xu, P. Gao, L. Yan, S. Kim, W. Wang. 2023. “A hybrid analytical and numerical model for the cross-over and performance decay in a unit cell vanadium redox flow battery," Journal of Power Sources. 578: 233210. DOI: 10.1016/j.jpowsour.2023.233210.
- Zeng C., S. Kim, Y. Chen, Y. Fu, J. Bao, Z. Xu, W. Wang. 2023. "In-situ Characterization of Kinetics, Mass Transfer, and Active Electrode Surface Area for Vanadium Redox Flow Batteries," Journal of The Electrochemical Society, 170(3): 030507. DOI: 10.1149/1945-7111/acbf7f.
- Fu Y., R.K. Singh, S. Feng, J. Liu, J. Xiao, J. Bao, Z. Xu, D. Lu. 2023. "Understanding of Low-Porosity Sulfur Electrode for High-Energy Lithium-Sulfur Batteries,” Advanced Energy Materials, 13(13): 2203386. DOI: 10.1002/aenm.202203386.
- Kim, S., L. Yan, W. Wang. 2023. “Asymmetric Vanadium-based Aqueous Flow Batteries.” Flow Batteries: From Fundamentals to Applications, Vol. 2, Ch 31. DOI: 10.100289783527832767.ch31.
- Zeng C., S. Kim, Y. Chen, Y. Fu, J. Bao, Z. Xu, W. Wang. 2022. "Characterization of electrochemical behavior for aqueous organic redox flow batteries," Journal of Electrochemical Society, 169(12): 120527. DOI: 10.1149/1945-7111/acadad.
- Shin S.H.R., H.-S. Lim, K.S. Han, A.J. Robinson, A. Hollas, B. M. Sivakumar, S.I. Johnson, J. Chun, W. Wang, V. Murugesan, P.K. Thallapally. 2022. "Porous Liquids as Electrolyte: A Case Study of Li+ and Mg2+Ion Transport in Crown Ether Based-Type II Porous Liquids," ACS Materials Letters, 5: 330-335. DOI: 10.1021/acsmaterialslett.2c00956.
- Wu, W., A. P. Wang, J. Luo, T. L. Liu. 2022. "A Highly Stable, Capacity Dense Carboxylate Viologen Anolyte towards Long- Duration Energy Storage," Angewandte Chemie International Edition, 62(7):e202216662. DOI: 10.1002/anie.202216662.
- Gao, P., A. Andersen, J. Sepulveda, G.U. Panapitiya, A. Hollas, E.G. Saldanha, V. Murugesan, W. Wang. 2022. “SOMAS: a platform for data-driven material discovery in redox flow battery development,” Scientific Data, 9: 740. DOI: 10.1038/d41597-022-01814-4.
- Fu, Y., J. Bao, C. Zeng, Y. Chen, Z. Xu, S. Kim, W. Wang. 2022. "A Three-Dimensional Pore-scale Model for Redox Flow Battery Electrode Design Analysis," Journal of Power Sources, 556: 232329. DOI: 10.1016/j.powsour.2022.232329.
- Liu, Y., R. Huang, W. Du, A. Singhal, Z. Huang. 2022. "High-Performance Transmission and Distribution Co-simulation with 10,000+ Inverter-Based Resources," IEEE Industry Applications Society Annual Meeting (IAS 2022). Pgs. 1-5. DOI: 10.1109/IAS54023.2022.9940149.
- Kalinin S.V., M. Ziatdinov, S.R. Spurgeon, C. Ophus, E.A. Stach, T. Susi, J. Agar, J. Randall. 2022. "Deep Learning for Electron and Scanning Probe Microscopy: From Materials Design to Atomic Fabrication," MRS Bulletin, 47: 931-939. DOI: 10.1557/s43577-022-00413-3.
Published in 2022
- Zeng C., S. Kim, Y. Chen, Y. Fu, J. Bao, Z. Xu, and W. Wang. 2022. "Characterization of electrochemical behavior for aqueous organic redox flow batteries." Journal of Electrochemical Society, 169(12): 120527. DOI: 10.1149/1945-7111/acadad.
- Gao, P. A. Andersen, J.P. Sepulveda, G.U. Panapitiya, A.M. Hollas, E.G. Saldanha, V. Murugesan. 2022. “SOMAS: a platform for data-driven material discovery in redox flow battery development.” Scientific Data. Accepted Oct. 31, 2022. PNNL-SA-161978.
- Fu, Y., J. Bao, C. Zeng, Y. Chen, Z. Xu, S. Kim, W. Wang. 2022. "A Three-Dimensional Pore-scale Model for Redox Flow Battery Electrode Design Analysis." Journal of Power Sources. Accepted Oct. 2022. PNNL-SA-175492.
- Kalinin S.V., Randall, T. Susi, J. Agar, M. Ziatdinov, S.R. Spurgeon, C. Ophus, E.A. Stach. 2022. "Deep Learning for Electron and Scanning Probe Microscopy: From Materials Design to Atomic Fabrication." MRS Bulletin. DOI: 10.1557/s43577-022-00413-3. PNNL-SA-171109. Front Cover Art.
- Fan, Y., H. You, X. Tian, X. Yang, X. Li, N. Prakash, Y. Yu. 2022. “A Meshfree Peridynamic Model for Brittle Fracture in Randomly Heterogeneous Materials,” Science Direct, 399: 115340. DOI: 10.1016/j.cma.2022.115340. PNNL-SA-179560. 09/01/2022. Stinis (Lehigh).
- Yan L., Y. Shao, W. Wang. 2022. "A hydrogen iron flow battery with high current density and long cyclability enabled through circular water management." Energy and Environmental Materials. DOI: 10.1111/eem2.12478. PNNL-SA-175158. 07/22/2022.
- He, Q., Y. Fu, P. Stinis, A.M. Tartakovsky. 2022. “Enhanced physics-constrained deep neural networks for modelling vanadium redox flow battery,” Journal of Power Sources, 542: 231807. DOI: 10.1016/j.jpowsour.2022.231807. PNNL-SA-170353. 06/25/2022.
- Zhang, L., R. Feng, W. Wang, G. Yu. 2022. “Emerging chemistries and molecular designs for flow batteries,” Nature Reviews Chemistry, 6: 524-543. DOI: 10.1038/s41570-022-00394-6. PNNL-SA-168763. 06/17/2022.
- Fan, Y., X. Tian, X. Yang, X. Li, C. Webster, Y. Yu. 2022. “An asymptotically compatible probabilistic collocation method for randomly heterogeneous nonlocal problems,” Journal of Computational Physics, 465:111376. DOI: 10.1016/j.jcp.2022.111376. PNNL-SA-179561. 06/07/2022.
- Howard, A.A., T. Yu, W. Wang, A.M. Tartakovsky. 2022. “Physics-informed CoKriging model of a redox flow battery,” Journal of Power Sources, 542: 231668. DOI: 10.1016/j.jpowsour.2022.231668. PNNL-SA-162807. 05/22/2022.
- Zhou, J., P. Chen, W. Wang, X. Zhang. 2022. “Li7P3S11 electrolyte for all-solid-state lithium-ion batteries: structure, synthesis, and applications.” Chemical Engineering Journal, 446: 137041. DOI: 10.1016/j.cej.2022.137041. PNNL-SA-168712. 05/14/2022.
- Yan, L., J. Bao, Y. Shao, W. Wang. 2022. “An electrochemical hydrogen looping process for CO2 capture from seawater,” ACS Energy Letters, 7(6): 1947-1952. DOI: 10.1021/acsenergylett.2c00396. PNNL-SA-168725.
- Panapitiya, G.U., M.K. Girard, A.M. Hollas, J. Sepulveda, V. Murugesan, W. Wang, E. Saldanha. 2022. “Evaluation of Deep Learning Architectures for Aqueous Solubility Prediction,” ACS Omega. DOI: 10.1021/acsomega.2c00642. PNNL-SA-161618.
- Xie, X., L. Du, L. Yan, S. Park, Y. Qiu, J. Sokolowski, W. Wang, Y. Shao. 2022. “Oxygen Evolution Reaction in Alkaline Environment: Material Challenges and Solutions,” Advanced Functional Materials, 32(21): 2110036. DOI: 10.1002/adfm.202110036. PNNL-SA-166448.
- He, Q., P. Stinis, A.M. Tartakovsky. 2022. “Physics-constrained deep neural network method for estimating parameters in a redox flow battery,” Journal of Power Sources, 528: 231147. DOI: 10.1016/j.jpowsour.2022.231147. PNNL-SA-163269.
Published in 2021
- Hu, B., M. Hu, Jian Luo, T. Liu, A. Hollas. 2021. “A Stable, Low Permeable TEMPO Catholyte for Aqueous Total Organic Redox Flow Batteries.” Advanced Energy Materials. 12(8): 2102577. DOI: 10.1002/aenm.202102577. PNNL-SA-169500.
- Gao, P., X. Yang, Y.-H. Tang, M. Zheng, A. Andersen, V. Murugesan, A.M. Hollas, W. Wang. 2021. “Graphical Gaussian process regression model for aqueous solvation free energy prediction of organic molecules in redox flow batteries,” Physical Chemistry Chemical Physics, 23 (43): 24892-24904. DOI: 10.1039/D1CP04475C. PNNL-SA-161057. 10/28/21.
- Panapitiya, G., F. Parks, J. Sepulveda, E. Saldanha. 2021. “Extracting Material Property Measurement Data from Scientific Articles,” 2021 Conference on Empirical Methods in Natural Language Processing. Pgs. 5393-5402. DOI: 10.18653/v1/2021.emnlp-main.438. PNNL-SA-162563.
- Hu, B., M. Hu, Jian Luo, T. Leo Liu. 2021. “A Stable, Low Permeable TEMPO Catholyte for Aqueous Total Organic Redox Flow Batteries.” Advanced Energy Materials. DOI: 10.1002/aenm.202102577.
- Gao, P., X. Yang, Y. Tang, M. Zheng, A. Andersen, V. Murugesan, A.M. Hollas, W. Wang. 2021. “Machine Learning for the Physicochemical Properties of Organic Molecules for Redox Flow Battery: Aqueous Solvation Free Energy Prediction.” Submitted to Physical Chemistry Chemical Physics, 23 (43): 24892-24904. DOI: 10.1039/D1CP04475C.
- Zhou, J., Y. Chen, Z .Yu, M. Bowden, Q.R.S. Miller, P. Chen, H.T. Schaef, K.T. Mueller, D. Lu, J. Xiao, J. Liu, W. Wang, X. Zhang. “Wet-chemical synthesis of Li7P3S11 with tailored particle size for solid state electrolytes.” Chemical Engineering, 429: 132334. DOI: 10.1016/j/cej/2021.132334.
- Li, X., P. Gao, Y.-Y. Lai, J.D. Bazak, A.M. Hollas, H.-Y. Lin, V. Murugesan, S. Zhang, C.- F. Cheng, W.-Y. Tung, Y.-T. Lai, R. Feng, J. Wang, C.-L. Wang, W. Wang, Y. Zhu. “Symmetry-breaking design of an organic iron complex catholyte for a long cyclability aqueous organic redox flow battery,” Nature Energy, 6: 873-881. DOI: 10.1038/s41560-021-00879-6.
- Chen, Y., J. Bao, Z. Xu, P. Gao, L. Yan, S. Kim, W. Wang. 2021. “A two-dimensional analytical unit cell model for redox flow battery evaluation and optimization.” Journal of Power Sources, 506: 230192. DOI: 10.1016/j.jpowsour.2021.230192.
- Wang, W. 2021. “A Membrane with Repelling Power,” Nature Energy, 6: 452-453. DOI: 10.1038/s41560-021-00811-y.
- Feng, R., X. Zhang, V. Murugesan, A.M. Hollas, Y. Chen, Y. Shao, E.D. Walter, N.P.N. Wellala, L. Yan, K.M. Rosso, W. Wang. 2021. “Reversible ketone hydrogenation for aqueous organic redox flow batteries,” Science, 372 (6544): 836-840. DOI: 10.1126/science.abd9795.
Published in 2020
- Chen, Y., Z. Xu, C. Wang, J. Bao, B. Koeppel, W. Wang. 2020. “Analytical modeling for redox flow battery design,” Journal of Power Sources, 482: 228817. DOI: 10.1016/j.jpowsour.2020.228817.
- Zhang L., Y. Qian, R. Feng, Y. Ding, X. Zu, C. Zhang, X. Guo, W. Wang, G. Yu. 2020. "Reversible redox chemistry in azobenzene-based organic molecules for high-capacity and long-life nonaqueous redox flow batteries." Nature Communications, 11 (1): 3843. DOI: 10.1038/s41467-020-17662-y.
- Bao, J., V. Murugesan, C.J. Kamp, Y. Shao, L. Yan, W. Wang. 2020. “Machine learning coupled multi-scale modeling for redox flow batteries,” Advanced Theory and Simulations, 3 (2): 1900167. DOI: 10.1002/adts.201900167.