ESMI Capabilities
The ESMI project at PNNL is pioneering new R&D approaches and developing new technologies to transform the field of materials science and accelerate development of a new generation of battery materials and chemistries for long-duration energy storage.
Automated Robotics for Energy Storage (ARES) Lab
Combining artificial intelligence and lab automation for high-throughput experimentation
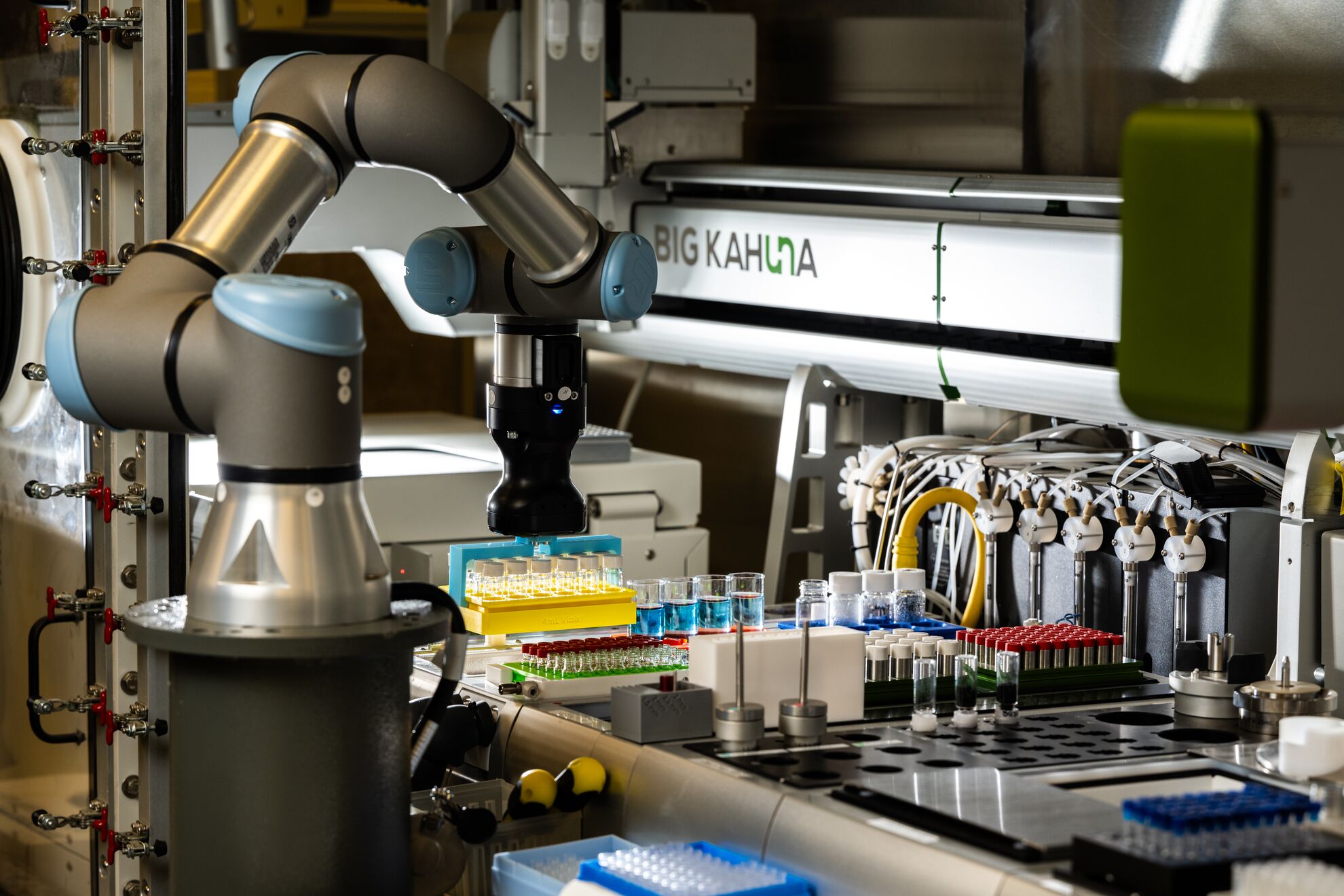
The Energy Storage researchers at PNNL have turbocharged their materials discovery research with the addition of the Automated Robotics for Energy Storage (ARES) lab. Anchored by two AI-guided High-Throughput Experimentation (HTE) platforms, capable of handling both aqueous and non-aqueous electrolyte studies, the ARES lab offers automated materials synthesis, screening, formulation, characterization, and optimization capabilities for large scale data generation.
Part robot, part workstation, part intelligent database, PNNL’s ARES lab functions autonomously using integrated machine learning algorithms and advanced software control, enabling researchers to accomplish tasks within a day that used to take weeks or even months. ESMI’s researchers can now perform hundreds of experiments per day all while maintaining a bird’s-eye view of results by using physics-informed data sets to improve experimental design and eliminate errors.
Intelligent Database for Materials Discovery
Physics-informed machine learning for better data analysis
The ARES Lab’s intelligent database makes it easier to organize and understand complex data related to the chemical properties of materials. The database uses physics-informed machine learning to characterize and predict material structure, solubility, electrical conductivity, and even cell performance for thousands of experimental organic molecules and liquid electrolytes for redox flow batteries, lithium-ion batteries, Sodium-ion batteries, and many other battery chemistries for energy storage.
This intelligent database enables ESMI’s researchers to significantly accelerate material discovery and predict material design through advanced machine learning models. Additionally, ESMI’s researchers are using a combination of multi-scale modeling and physics-informed machine learning to create models for prototype cells and stacks of cells for redox flow batteries.
By working faster, smarter, and more efficiently, ESMI’s researchers can get closer to developing new and advanced materials for energy storage from the molecules to devices and systems—bringing the nation closer to a decarbonized energy future.