Technical Approach
The different algorithms developed as part of the project and their technical approaches can be summarized as:
- Online attack identification using streaming data: An online attack identification algorithm has been developed that performs a spatio-temporal nonlinear modal analysis on streaming sensors data, based on Koopman operator theory. The algorithm detects as well as localizes malicious activities. Strategic attacks initiated during naturally-caused transients due to abrupt changes in load and generation can fool the conventional model-based detection methods, relying on thresholds, comparison to trigger an anomaly. The developed model-agnostic attack identification algorithm uses Koopman modes to learn the spatio-temporal signatures from streaming measurements data and successfully detects and localizes stealthy sensor attacks.
- Deep learning-based surrogate models for predicting transients: Deep learning-based surrogate models, based on the Koopman operator theory of nonlinear systems, have been developed for predicting the power systems state trajectories at transient timescales. Performances of these models have been compared using data generated on GridSTAGE. In particular, a deep learning implementation of Koopman operator, based on the deep dynamic mode decomposition (DeepDMD), is demonstrated to deliver successful predictive performance on IEEE 68-bus system. Detailed performance analysis of the DeepDMD model is carried out, using metrics such as the root mean square error (RMSE) and the mean absolute percentage error (MAPE), and its comparison with other deep learning predictive model, spatiotemporal graph convolution network (STGCN), is reported. Robustness of the prediction performance is demonstrated both spatially (at multiple buses across the power system network) and temporally (at varying lengths of the prediction horizon). The surrogate models are then used for training the deep RL policies.
- Koopman-Based Distributed Learning of Multiagent Systems: In the above-mentioned prediction algorithm solution has been obtained by solving a centralized minimization problem. However, scaling up is a challenge when working with large systems as we find in the real world. Therefore, a distributed approach for computing the Koopman operator for large scale networks has been developed. The dynamical system is treated to be a multi-agent system and an algorithm has been developed to compute the finite-dimensional approximation of the Koopman operator in a distributed manner using the knowledge of the topology of the underlying multi-agent system. The proposed distributed approach is shown to be equivalent to the centralized learning problem and results in a sparse Koopman whose block structure mimics the Laplacian of the multiagent system.
- Deep RL-based defense strategies: A deep Q-learning based model has been developed for recommending defense actions (e.g. generator setpoints) for the cyber-physical power network in order to maximize system-level reliability and resilience, and mitigate any adversarial impacts. Our RL-approach is distinguished by incorporation of the underlying topology through graph representation learning methods and accounting for the dynamical system behavior through the incorporation of Koopman encoding. In particular, using the variance of frequency measurements as the reward function, we train a multilayer perceptron as the policy network to synthesize the generator control setpoints. Largescale simulations of attackdefense scenarios have been performed to generate training data for RLbased algorithm. Simulations are instrumented to collect topological metrics describing system configurations, as well as various performance metrics useful to construct the rewards functions for RL policies.
We have also developed an open-source simulation framework and published datasets for use by the large power systems community. GridSTAGE (Grid: Spatio Temporal Adversarial Scenario GEneration framework) is a simulation framework to synthesize strategic adversarial attack scenarios in power systems, along with different faults and contingencies, to generate time-series of sensor measurements mimicking different operational settings (e.g. with automatic generation control in-the-loop). GridSTAGE is utilized for performing time-domain nonlinear simulations on IEEE bus systems and multivariate spatiotemporal datasets are generated. Different event scenarios can be created by enabling or disabling any of the following faults, AGC control, PSS control, exciter control, and different types of cyberattacks. Sensors in the system model include both PMU and SCADA, and simulation data are generated for both of these sensors. This framework currently supports ramp, step, random, trapezoidal, time-delay, packet-loss, freezing, replay and multiplicative attacks on sensors. The repository is available here: https://github.com/pnnl/GridSTAGE .
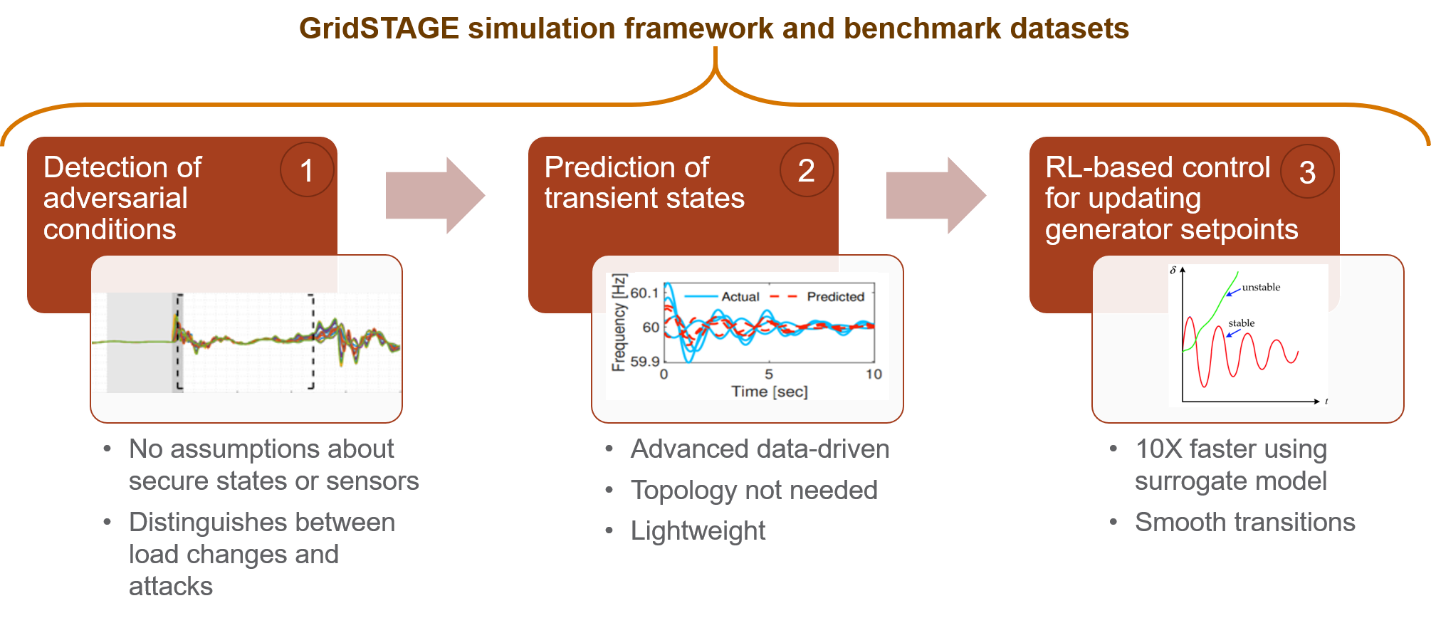