PowerDrone: Adaptive Steering of Power Systems for Resilient Operation under Adversarial Conditions
A data-driven approach towards detection and mitigation of adversarial conditions in the power grid to adaptively steer the system back to secure operational mode.
POC: Sutanay Choudhury
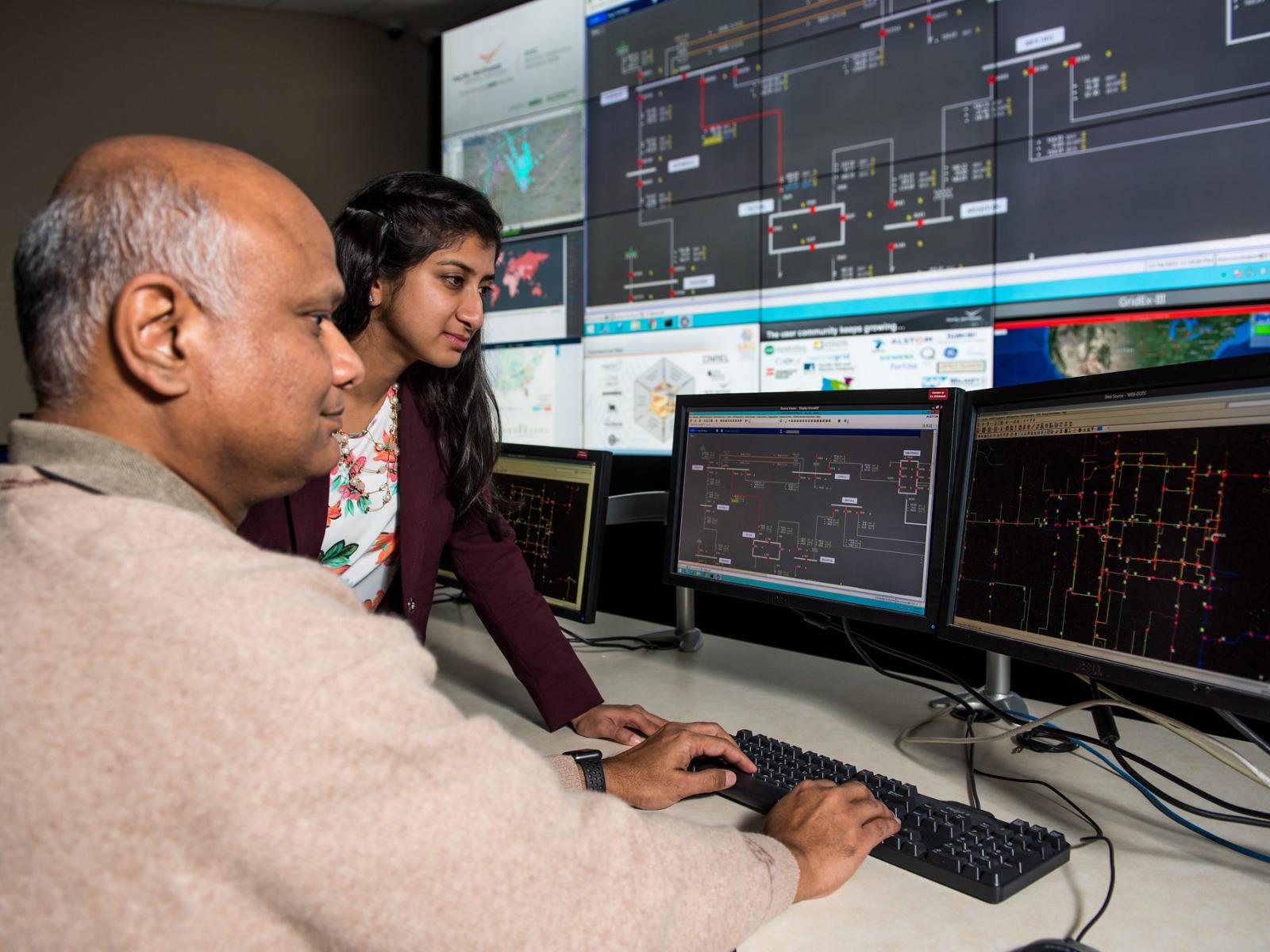