Stinis to Lead Two New Scientific Machine Learning Projects
Stinis will serve as a principal investigator on two of the four projects selected for funding
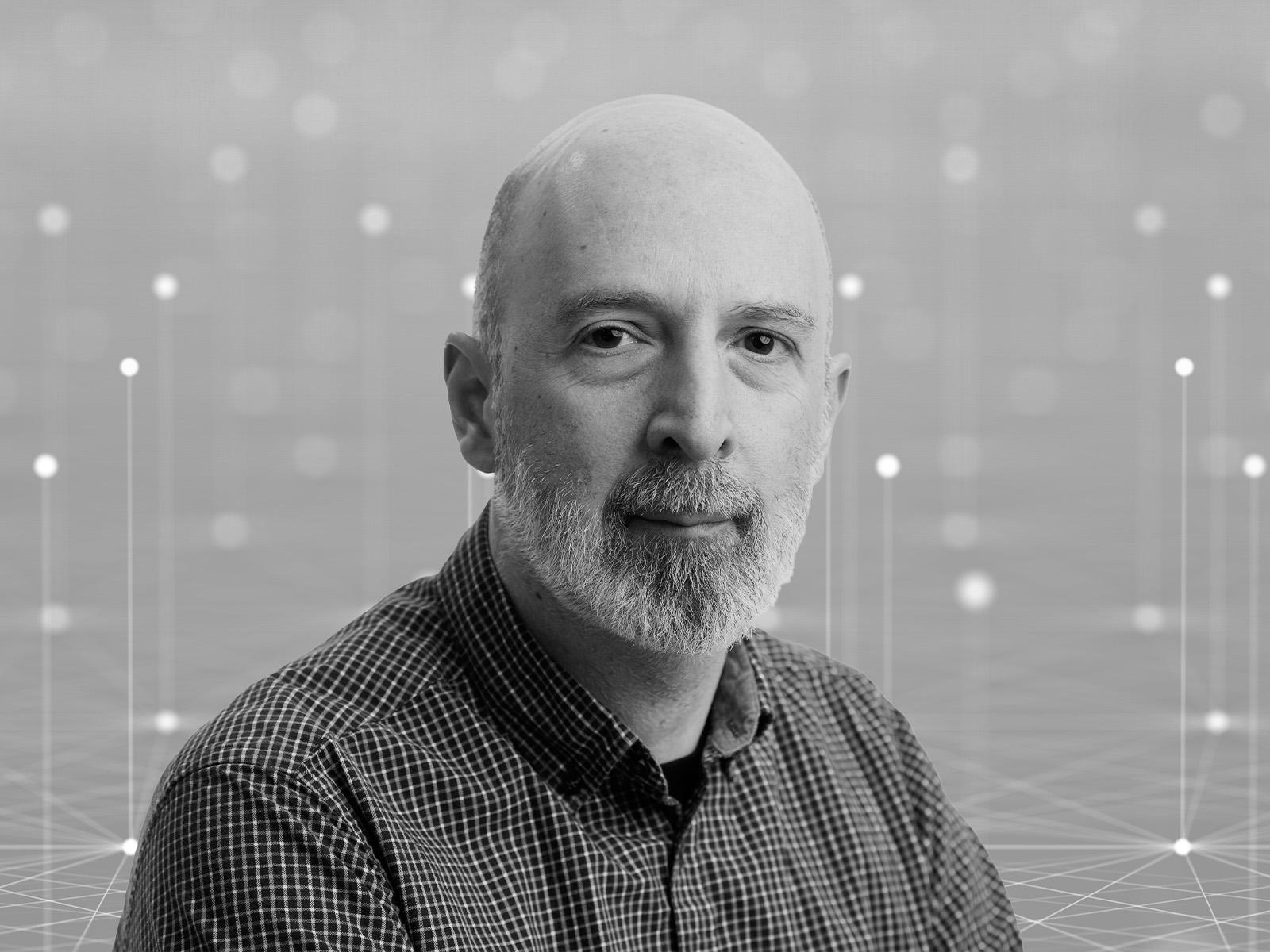
Panos Stinis will lead two new scientific machine learning projects: one in collaboration with Spelman College, and another with the University of Pennsylvania and Sandia National Laboratories.
(Composite image by Kelly Machart | Pacific Northwest National Laboratory)
The Department of Energy (DOE) Advanced Scientific Computing Research (ASCR) program recently announced funding for four projects in scientific machine learning for complex systems. Pacific Northwest National Laboratory (PNNL) Mathematician Panos Stinis will serve as a principal investigator on two of those four projects.
“Basic research for scientific computing and machine learning continues to have a wide impact across a range of applications,” said Ceren Susut, the DOE acting associate director of science for ASCR. “These projects are important for advancing our modeling, simulation, and data analysis capabilities for scientific discovery and innovation.”
In the (MOLUcQ) Uncertainty Quantification for Multifidelity Operator Learning project Stinis will partner with Erdi Kara from Spelman College to improve predictions in atmospheric simulations and measurements.
Stinis will also co-lead the Resolution-Invariant Deep Learning for Accelerated Propagation of Epistemic and Aleatory Uncertainty in Multiscale Energy Storage Systems, and Beyond project with Paris Perdikaris from the University of Pennsylvania and Nathaniel Trask from Sandia National Laboratories.
These projects build upon discoveries made through the Scalable, Efficient and Accelerated Causal Reasoning Operators, Graphs and Spikes for Earth and Embedded Systems (SEA-CROGS) and Physics-Informed Learning Machines for Multiscale and Multiphysics Problems (PhILMs) efforts.
“The two projects will advance machine learning techniques for combining multiple sources of knowledge about real-world multiscale systems and studying the propagation of uncertainty across the scales,” said Stinis. “The developed techniques will improve the predictive capability for problems of relevance to the DOE, like atmospheric simulations and batteries.”
Stinis earned his PhD in applied mathematics in 2003, from Columbia University in New York. He subsequently worked at Lawrence Berkeley National Laboratory, the Stanford Center for Turbulence Research, and the University of Minnesota before arriving at PNNL. He currently leads the Computational Mathematics group in the Advanced Computing, Mathematics, and Data Division at PNNL. He also serves as the co-director of the PhILMs collaboration and leads the Digital Twin Component Development thrust of the Energy Storing Materials Initiative.
Published: September 15, 2023