PNNL Papers on Machine Learning Accepted to AAAI-21
Data scientists Henry Kvinge and Ted Fujimoto presented their teams’ research at top artificial intelligence conference workshops
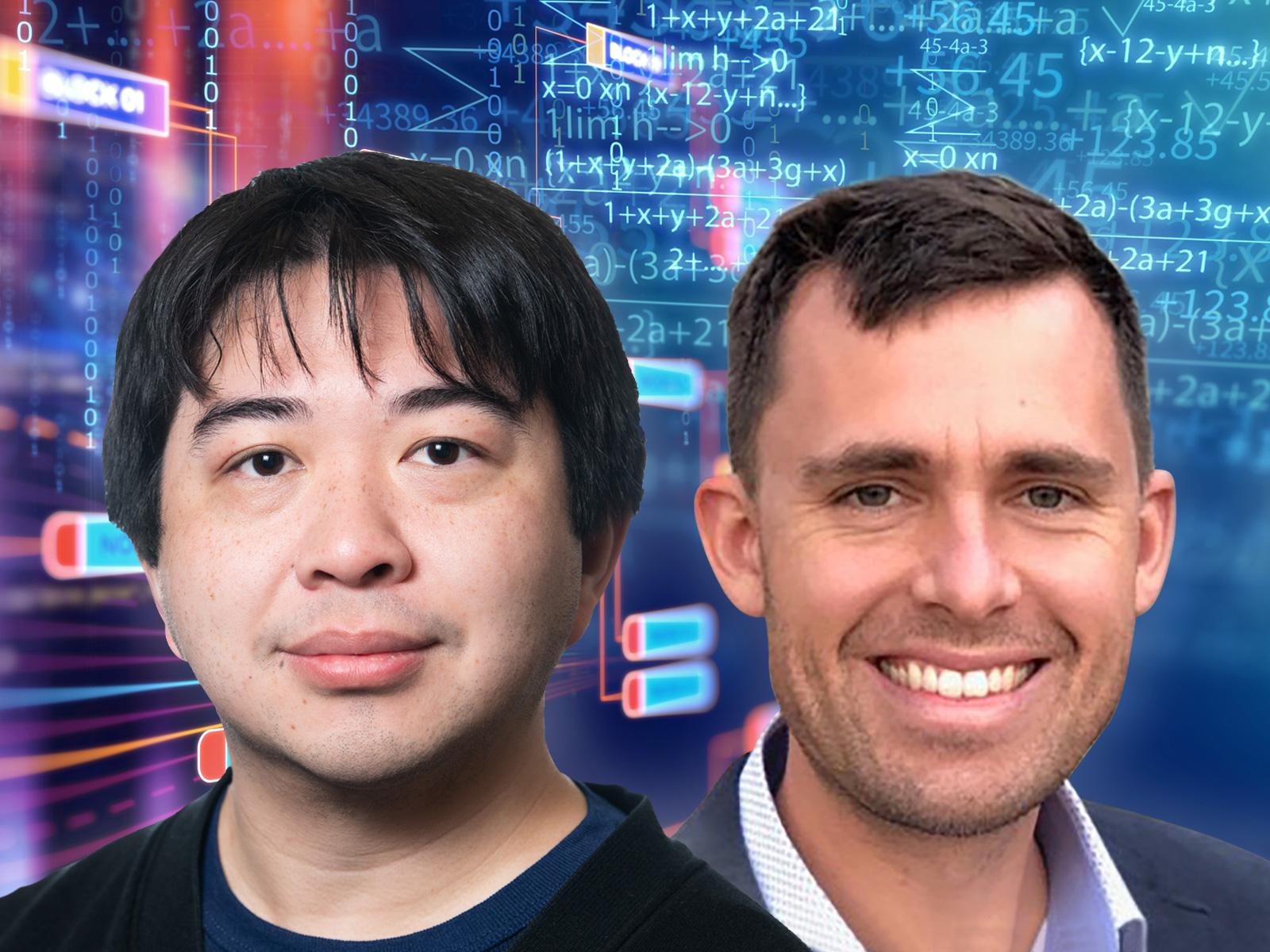
PNNL data scientists Ted Fujimoto (left) and Henry Kvinge presented their research on machine learning at the 2021 AAAI Conference on Artificial Intelligence.
(Composite image by Jeffrey London | Pacific Northwest National Laboratory)
Two Pacific Northwest National Laboratory (PNNL) papers on machine learning were featured at workshops during the 2021 AAAI Conference on Artificial Intelligence, Feb. 2–9. The conference, also known as AAAI-21, was sponsored by the Association for the Advancement of Artificial Intelligence, a top international scientific society focused on the research and use of artificial intelligence (AI).
Building a new topology-inspired few-shot learning model
Data scientist Henry Kvinge presented his team’s work to develop a new few-shot learning model at the AAAI-21 Workshop on MetaLearn Challenges. The model uses ideas from topology to build a low-dimensional representation of a class of objects from a limited number of examples.
Titled, “Fuzzy Simplicial Networks: A Topology-Inspired Model to Improve Task Generalization in Few-Shot Learning,” the paper is part of PNNL scientists’ work to create machine learning tools that can learn from very small amounts of data. More adaptive than traditional few-shot learning models, the new topology-based model could help improve task generalization in machine learning.
“This work is connected to the larger goal of creating models that can adapt to a broad range of tasks on the fly, rather than being limited to the specific task they were trained on,” Kvinge said.
Kvinge was the paper’s lead author. Co-authors include PNNL staff members Zachary New, Nicolas Courts, Jung Lee, Lauren Phillips, Courtney Corley, Aaron Tuor, Andrew Avila, and Nathan Hodas.
Understanding antagonistic behavior in reinforcement learning
Ted Fujimoto, a postgraduate research associate in PNNL’s Computing and Analytics Division, presented at the AAAI-21 Workshop on Reinforcement Learning in Games about his team’s paper on adversarial behavior in machine learning. The study explored how a purely antagonist agent performs compared to a well-trained victim that learns directly from a reward system.
Fujimoto said the paper, “The Effect of Antagonistic Behavior in Reinforcement Learning,” aims to “capture the intuition behind antagonistic behavior, to formalize it, and to see how such behavior emerges from intelligent agents.” The research is part of PNNL’s work to build stronger autonomous systems by understanding and mitigating failures caused by malicious activities of adversaries.
“This framework can be used as a starting point toward applying reinforcement learning models to develop more secure, safe, and robust AI systems,” Fujimoto said. “For example, as a long-term goal, we could protect against how a human architect might develop an antagonist agent to disrupt automated systems, such as autonomous vehicles or delivery drones.”
In addition to Fujimoto, contributing authors to the paper include PNNL scientists Timothy Doster, Adam Attarian, Jill Brandenberger, and Nathan Hodas.
Published: February 25, 2021