How PNNL Is Using Machine Learning To Detect Nuclear Threats Quicker and Easier

PNNL research combines machine learning, data analytics and artificial reasoning with nonproliferation and safeguards expertise to keep our nation safe against nuclear threats.
(Composite image by Shannon Colson | Pacific Northwest National Laboratory)
Machine learning made news in 1962 when an IBM computer beat a human in checkers.
This event embodied the very definition of machine learning, as the computer was able to learn without explicitly being programmed.
Today, machine learning underpins many everyday encounters—from personalized shopping recommendations to voice-driven assistants like Siri and Alexa.
At the Department of Energy’s Pacific Northwest National Laboratory, researchers are developing new machine learning techniques that allow humans and computers to team in innovative ways to accelerate discovery and understanding.
They are creating secure, trustworthy, science-based systems that use machine learning to address challenges in science, energy, health and security.
In national security, for example, our experts are combining expertise in nuclear nonproliferation and artificial reasoning to detect and mitigate nuclear threats.
Their aim is to use data analytics and machine learning to monitor nuclear materials that could be used to produce nuclear weapons.
For instance, nuclear reprocessing facilities in non-nuclear weapon states are monitored by the International Atomic Energy Agency to be sure that plutonium separated from spent nuclear fuel is not diverted for nuclear weapons production.
In addition to regular inspections, the IAEA uses sample analysis and process monitoring to help implement safeguards for nuclear materials.
Analyzing the process data from reprocessing facilities is time- and labor-intensive. Working with collaborators from Sandia National Laboratories, nonproliferation analysts at PNNL sought to design a system that could automatically detect suspicious activity.
Their system relied on a machine learning technique known as a “transformer-based model” that can assign different weights to each part of the input data based on significance.
These models are often used in applications where the sequence or position of the data is important, like language processing.
Our researchers determined that these models could also capture important temporal patterns in data from reprocessing plants.
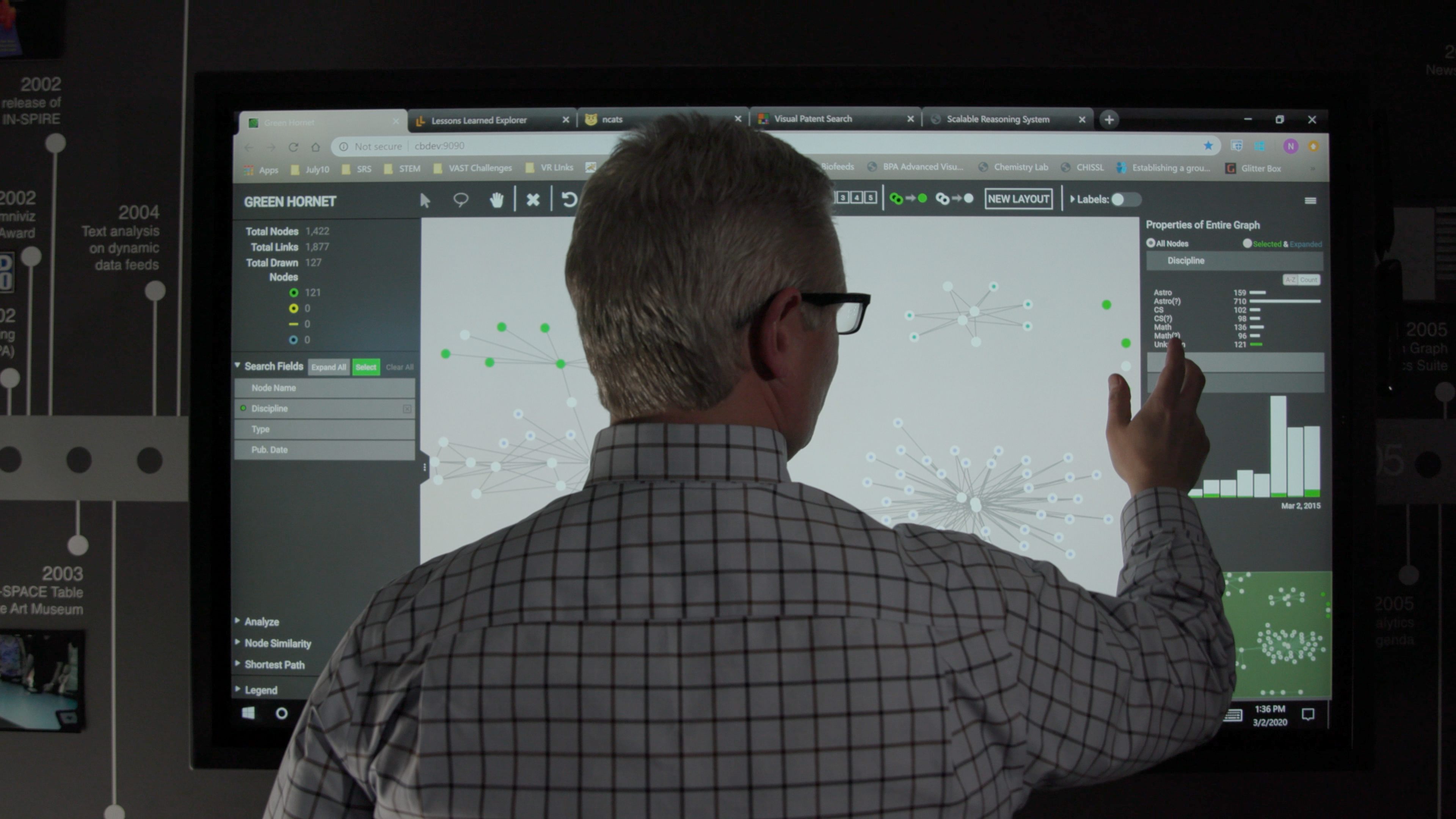
The researchers started by building a virtual replica of a reprocessing facility. They used the data generated by this virtual facility to train their transformer-based model.
Once the model could capture patterns in the data and make predictions about what would be expected for several locations within the facility, they designed a system to detect differences between those predictions and observed data from each location.
If something abnormal was detected—indicating a potential diversion—inspectors could be alerted to investigate. In addition, this system could identify which area in the process appears most anomalous.
Although this technique is not ready for deployment, early results were promising.
In another effort, PNNL and collaborators applied machine learning to help law enforcement agencies speed investigations when they find nuclear materials in the field.
Their approach to forensic analysis uses a model called an autoencoder, which learns to compress and decompress images into small descriptions that are useful for computational analysis. In this case, the model was looking at images of microscopic radioactive particles.
A radioactive material will have a unique microstructure based on the environmental conditions or purity of the source materials at its production facility.
By comparing the microstructures of field samples with a library of electron microscope images developed by university and national laboratory collaborators, investigators could quickly identify the material’s “fingerprint.”
These clues could help analysts determine the material’s origin, guide investigations and aid in deterring proliferation.
Machine learning algorithms and computers will not replace humans in detecting nuclear threats any time soon. But they may make it possible for people to discover important information and identify risks more quickly and easily.
As a computer scientist, I am particularly interested in the advancements being made by our researchers in this field today and how they will help to make the world a safer place for us all.
Steven Ashby, director of Pacific Northwest National Laboratory, writes this column monthly. To read previous Director's Columns, please visit our Director's Column Archive.
Published: March 27, 2023