Accomplishments
Estimation:
Tests with a 16-generator system demonstrated good tracking performance of an EnKF-based dynamic state estimation (Figure 2). The test system consisted of 16 generators, 86 transmission lines, and 68 buses. A 0.05-second disturbance from a three-phase-to-ground fault at a bus at 1.1 second was simulated with 3% noise added to all simulated voltages. The estimated rotor angle and rotor speed of one generator is shown in Figure 2. As can be seen, initial errors in the state variables affect only the state variable tracking for the initial time period. After a short time, e.g., about 1 second, there is no significant error for the state tracking. The disturbance does cause some deviation in the tracking. The Kalman filter can correct itself with continuous data and track the states again. Overall performance indicates that once the EnKF is locked into tracking, the accuracy of tracking is consistently good for continuous tracking.
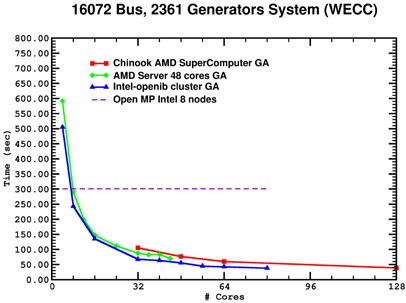
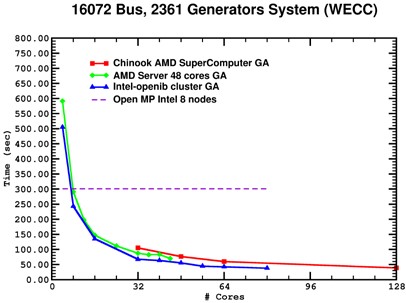
Figure 3. Computational performance of EnKF-based dynamic state estimation with a 16072-bus system: (a) execution time; (b) speedup
Besides tracking accuracy, estimating dynamic states fast enough is another important aspect for real-time application. The Kalman filter has high computation demands and requires a large number of FLOPS (floating point operations per second), especially for large power grids. HPC computers with thousands to hundreds of thousands of processor cores are needed to speed up the computation. Implementing and parallelizing codes on such HPC computers is a fundamental challenge. An initial attempt (Figure 3) has shown a promising path forward to achieve the required computational performance. Figure 3(a) is the time used to compute one time step of the EnKF dynamic state estimation for a Western Electricity Coordinating Coundil (WECC)-size system. The parallel codes are based on the Global Array programming model. Although the best time, at 128 cores, is still about 1000 times longer than 0.03 second (the phasor measurement cycle), the execution time decreases consistently as more processor cores are applied. This is confirmed by the speedup curve in Figure 3(b). Dynamic state estimation is very attainable, especially for regional power system.
Calibration
We developed an innovative tool suite and a complete procedure for power plant model validation and calibration. The main flowchart of the developed integrated tool suite is shown in Figure 1.
The model validation function is realized in the Power Plant Model Validation (PPMV) tool, with the concept shown in Figure 4. A subsystem model needs to be created first by using an infinite generator to represent the rest of the power network. The PMU measurements collected at the equivalent generator bus, including voltage magnitude and frequency (or phase angle), are injected into the network; while running a dynamic simulation. The model outputs, including active and reactive power from the power plant, will be compared against the corresponding PMU measurements. If the difference between simulated response and measured response is sufficiently small, the model accuracy is good, and parameter calibration is not needed. The PPMV tool was created to automate this process for a number of different events and/or dynamic parameter files; it can read PMU data, SCADA data (for providing generator status), power-flow files, and dynamic parameter files, and then run the validation process to check model performance. Currently, the interface is developed for the commercial software GE PSLF and SIEMENS PTI PSSE.
We developed an advanced EnKF based algorithm to automatically calibrate model parameters; it is able to effectively estimate the states of a dynamic system corresponding to feedback from measurements. Unlike the extended Kalman filter (EKF), which relies on a linear assumption, the EnKF algorithm can estimate states of a nonlinear system. The EnKF algorithm uses Monte Carlo samples (called ensembles) to represent and propagate the distribution of states and therefore circumvent the laborious and problematic linearization procedure used by the EKF. The EnKF based tool is implemented and integrated in commercial software GE PSLF in collaboration with GE Energy Consulting. Integrating the EnKF algorithm in commercial software package and utilizing its industry standard and well-maintained stability model libraries greatly enhanced the adaptability and validity of the proposed parameter calibration algorithm for different power plants being used in the power industry.
The developed model validation and calibration tools were tested on a realistic hydro power plant for several events. Figure 5 shows the model validation results under three different events with original parameters (green curves) and calibrated parameters (red curves), where the blue curves represent actual PMU measurements. It is clearly shown in Figure 5 that the original parameters of the model is not consistent with the real physical model, as the real and reactive power curves with original parameters (green curves) have a large discrepancy with the actual PMU measurements (blue curves). The effectiveness of the proposed EnKF algorithm is verified also from Figure 5, since the real and reactive power curves with calibrated parameters match the PMU measurements much better than the curves with original parameters.