Wildfire Typing Improves Wildfire Predictions in the Western United States
Clustering wildfires into four types based on their land-atmosphere drivers can potentially improve regional wildfire predictions
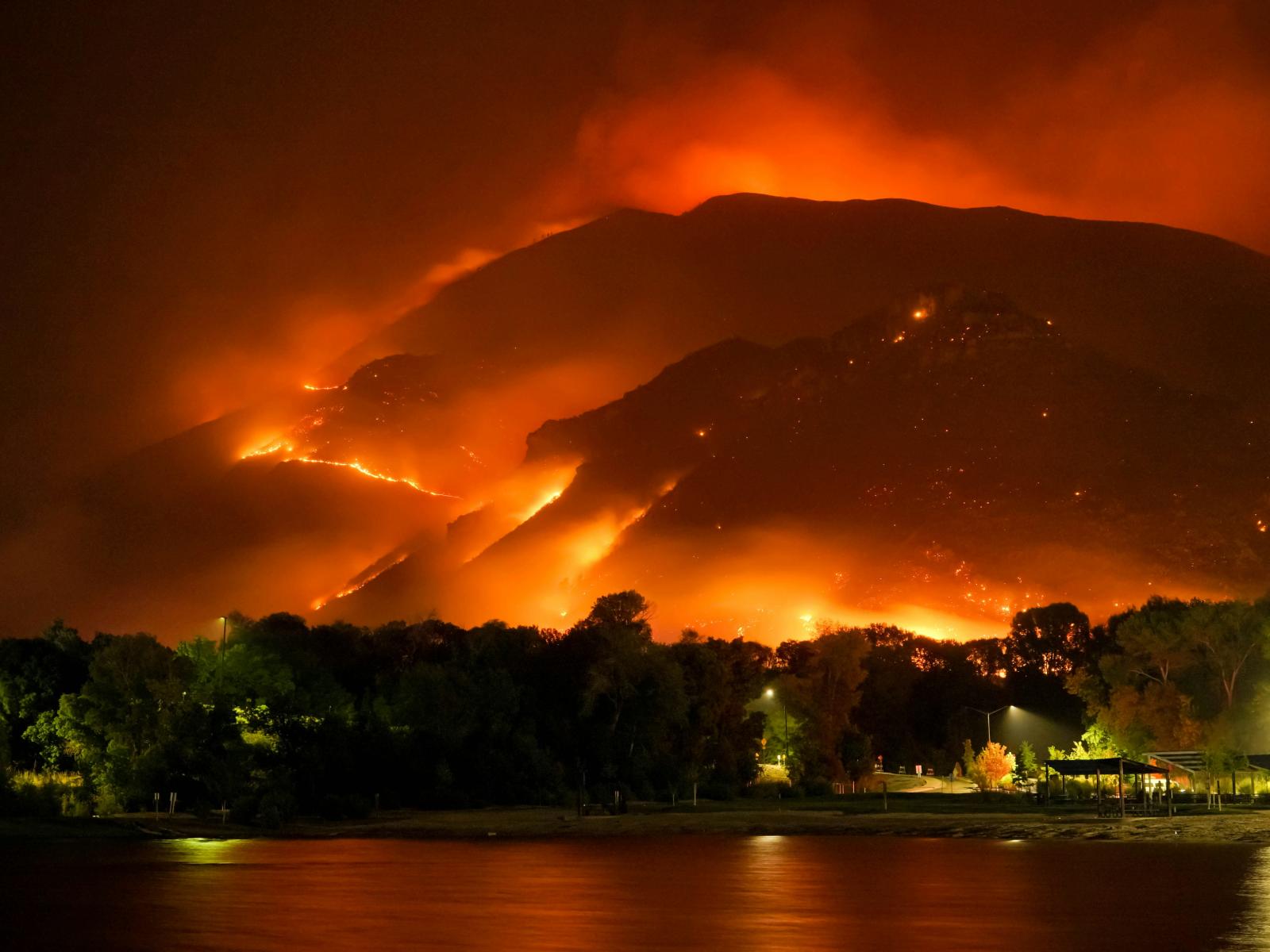
The Eagle Creek Fire burns in 2017 in the Columbia River Gorge in Oregon. Wildfires in the Western United States are usually triggered by hot and dry weather conditions, but one recent study identified four unique types of land-atmosphere conditions before fire ignitions that are conducive to wildfires and clustered fires into these four categories. Utilizing this clustering scheme can lead to better regional wildfire predictions.
(Photo by Mike Newbry | Unsplash)
The Science
Wildfires in the Western United States (U.S.) have become more frequent and hazardous under warming, motivating a better understanding of the environmental conditions before fire ignition to improve wildfire predictions and disaster management. Using historical wildfire records and a high-resolution climate simulation over the Western U.S., scientists identified four types of wildfires, each showing a distinct combination of energy and moisture conditions before fire ignition. They also revealed diverse, sometimes opposite, relationships between the occurrences of these wildfire types and the environmental conditions, which are often stronger than similar relationships based on all wildfires as a single population. Wildfire prediction models based on this wildfire clustering can improve the prediction accuracy of regional wildfire occurrence by ~10%.
The Impact
Wildfires are a vital component of the regional water cycle and ecological system. They have significant impacts on vegetation, wildlife, and soil composition. They can cause substantial human and economic losses. While many fire forecasting/prediction schemes exist, there are opportunities to provide better predictions using improved methodologies. This study used a machine learning clustering method to identify four types of wildfires in the Western U.S. The findings suggest that wildfire predictions can be improved by incorporating this wildfire clustering scheme into current fire prediction systems. This study also demonstrates the importance of considering the integrated hydroclimate conditions in improving understanding of the environmental drivers of wildfires.
Summary
Researchers analyzed wildfire observations and a regional storm-resolving hydroclimate simulation over the Western U.S. during the warm season (April–September) of 1984–2018. All 5,175 wildfire events feature a consistently drier and warmer atmosphere, with some common indices such as vapor pressure deficit showing good predictive power. However, wildfires tend to occur under diverse surface pressure, atmospheric radiation, and soil moisture conditions before fire ignition, suggesting these conditions may better explain the variability of fire occurrence. Using the K-means clustering method, researchers identified these distinct combinations of these environmental conditions—high versus low surface pressure, intense longwave radiation, and wetter soil. They also clustered regional wildfires into these four corresponding categories.
Researchers revealed stronger statistical correlations between individual wildfire categories and regional atmospheric and land conditions compared to all wildfires as a whole. Based on a simple predictive model, seasonal meteorological and land conditions exhibit better predictive skills for the seasonal occurrence of each category of wildfire—thus, all wildfires. Two sources of such improved predictability are identified: (1) most lightning-ignited wildfires fall into the longwave radiation type, which captures most of the randomness in fire ignition and makes the other three categories more predictable, and (2) wet soil wildfires often show opposite environmental correlations compared to the other three categories. Thus, separating wet soil wildfires can improve predictions because the correlations and the predictors would otherwise be very low for predicting wildfires as a whole. Incorporating this wildfire clustering scheme also shows the potential of better operational fire forecast systems, which may improve water and natural disaster management practices.
Contact
L. Ruby Leung, Pacific Northwest National Laboratory, ruby.leung@pnnl.gov
Funding
This research is supported by the Department of Energy, Office of Science, Biological and Environmental Research program as part of the Regional and Global Modeling and Analysis and MultiSector Dynamics program areas.
Published: February 15, 2024
Chen, X., Leung, L. R. & L. Dong. “Antecedent Hydrometeorological Conditions of Wildfire Occurrence in the Western U.S. in a Changing Climate”. Journal of Geophysical Research: Atmospheres, 128, e2023JD039136 (2023).