NeuroMANCER Shoots for the Stars
This deep learning framework is PNNL’s most popular repository on GitHub
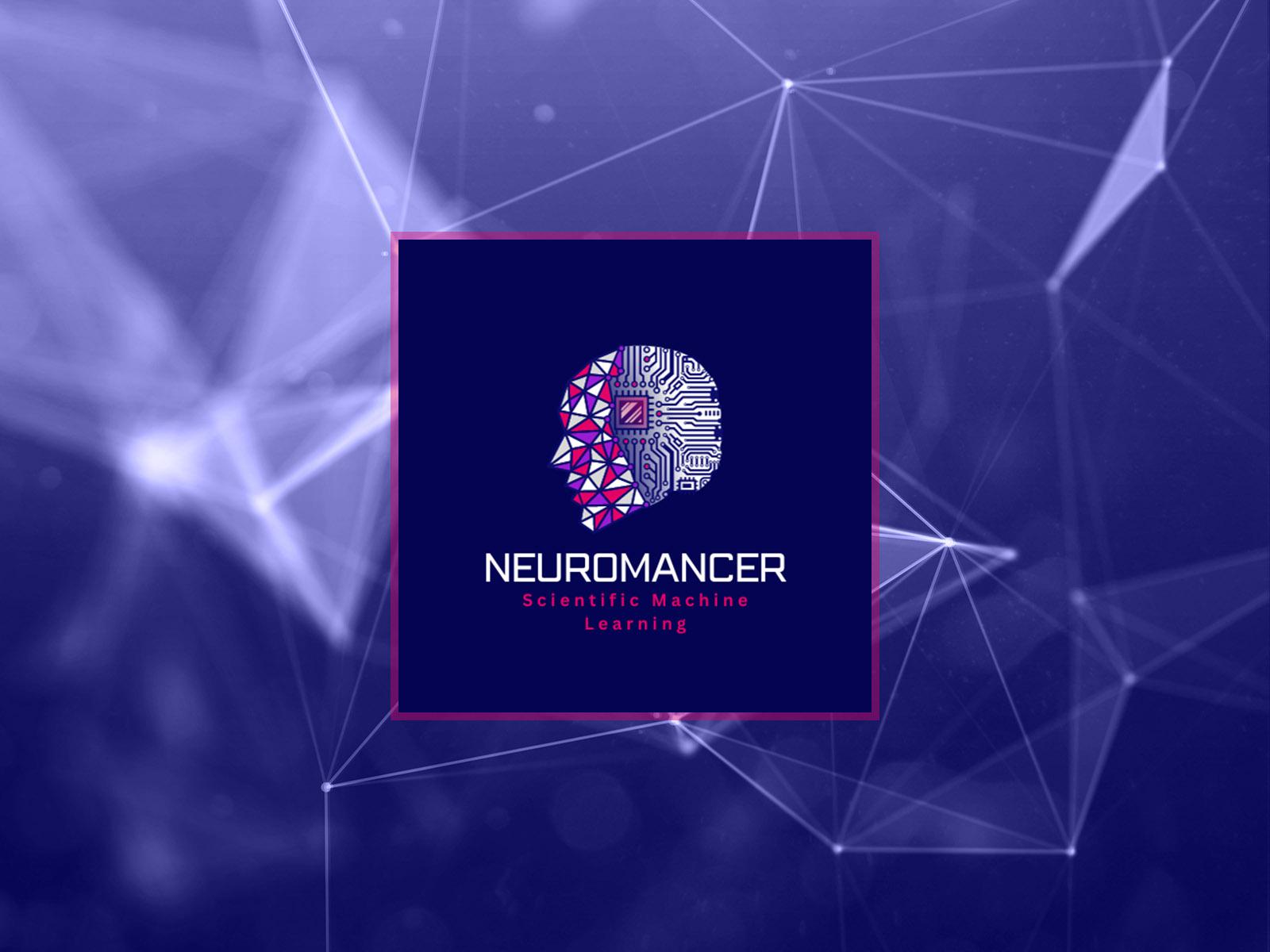
NeuroMANCER makes scientific machine learning accessible to domain scientists.
(Image courtesy of Jan Drgona | Pacific Northwest National Laboratory)
From power grid load forecasting to efficient control of building energy systems, simulations allow scientists to make decisions that would be impossible without computational help. Despite their importance, some simulations have proven to be too costly to run in certain systems, such as in building control optimization. Scientific machine learning (SciML) connects physics with artificial intelligence (AI) to find data-driven solutions to these challenges.
Differentiable programming offers a unifying approach for data-driven modeling and optimization of complex systems by leveraging the infrastructure of modern deep learning. However, in 2019, no easy-to-use, open-source tool existed for science applications needed by the Department of Energy (DOE). A team of researchers at Pacific Northwest National Laboratory (PNNL) developed NeuroMANCER—Neural Modules with Adaptive Nonlinear Constraints and Efficient Regularizations—to address DOE science needs. This SciML library became so popular with researchers it is now PNNL’s most used open-source tool based on GitHub stars.
Jan Drgona, who is a data scientist at PNNL, developed NeuroMANCER alongside colleagues Aaron Tuor, Madelyn Shapiro, Rahul Birmiwal, James Koch, and Draguna Vrabie.
“When we created NeuroMANCER, we initially wanted to create a library for our team to use,” said Drgona. “We quickly realized how useful it was for the broader community, so we focused on making it as open and easy to use as possible.”
Drgona produced a series of informational YouTube videos to teach others about NeuroMANCER and how to use it. “The videos are intended to help anyone in the scientific community to get started with NeuroMANCER,” he said.
With its open-source code and multiple educational resources, NeuroMANCER democratizes SciML and empowers smaller businesses and independent engineers in deploying advanced optimization and control algorithms.
Drgona and Vrabie will present NeuroMANCER in a hands-on coding tutorial at the LEANOPT-24 workshop as part of the Thirty-Eighth AAAI Conference on Artificial Intelligence.
The development of NeuroMANCER was partially supported by the Mathematics for Artificial Reasoning in Science and Data Model Convergence initiatives via the Laboratory Directed Research and Development investments at PNNL, by the DOE Office of Advanced Scientific Computing Research’s “Data-Driven Decision Control for Complex Systems” project, and through the Office of Energy Efficiency and Renewable Energy, Building Technologies Office under the “Dynamic Decarbonization through Autonomous Physics-Centric Deep Learning and Optimization of Building Operations” and the “Advancing Market-Ready Building Energy Management by Cost-Effective Differentiable Predictive Control” projects. NeuroMANCER is being used to support decision making and control in autonomous materials synthesis as part of the Adaptive Tunability for Synthesis and Control via Autonomous Learning on Edge initiative at PNNL.
NeuroMANCER is one of many AI-related projects at PNNL. The Laboratory recently launched the Center for AI @PNNL to coordinate PNNL’s AI capability development and application of AI.
Published: February 20, 2024