PNNL @ AAAI 2024
PNNL researchers showcase their work at the 38thAAAI Conference on Artificial Intelligence
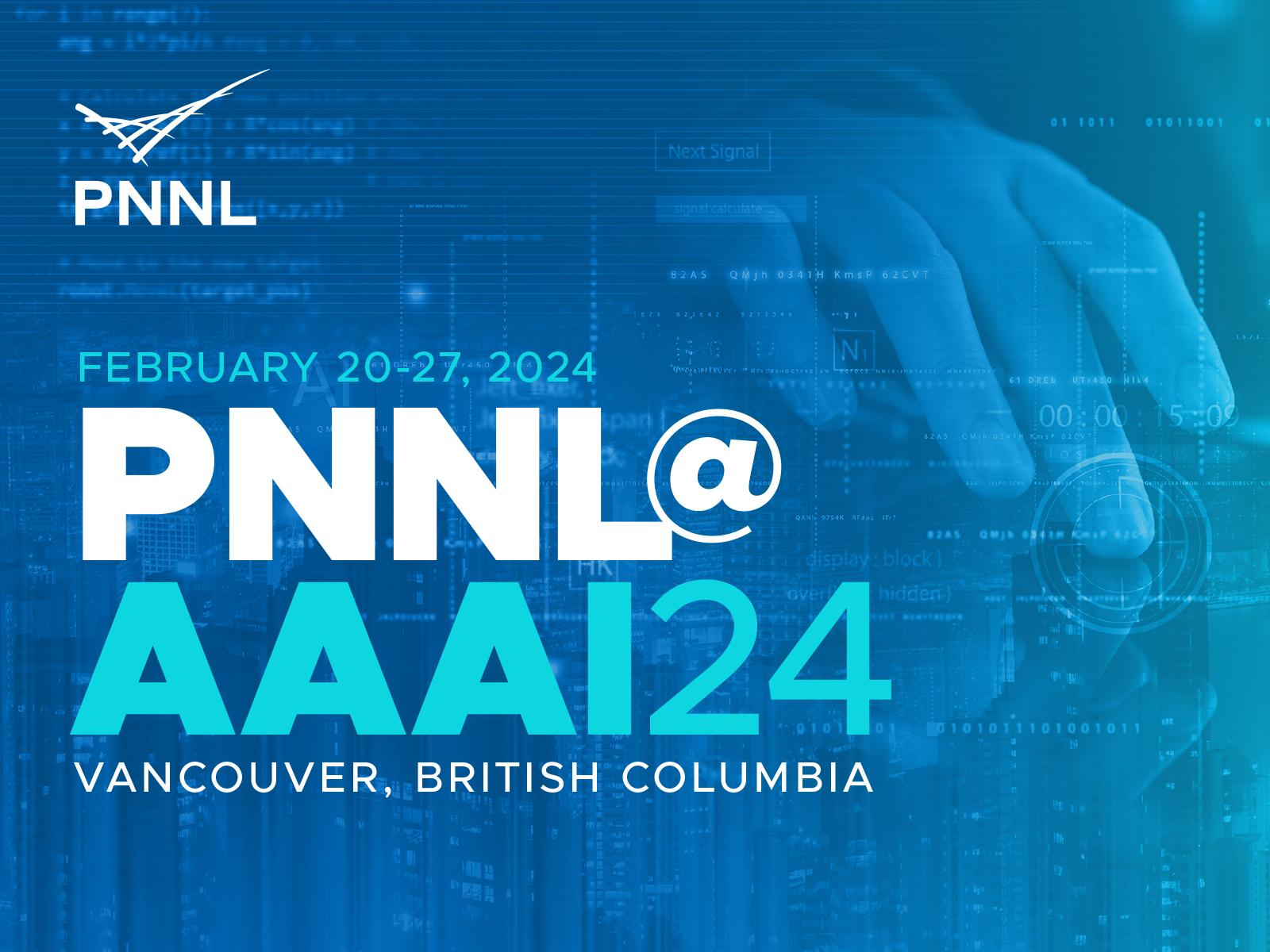
Vancouver, British Columbia
Pacific Northwest National Laboratory (PNNL) will be participating at the Thirty-Eighth AAAI Conference on Artificial Intelligence on February 20–27 in Vancouver, British Columbia.
The AAAI conference promotes research in artificial intelligence and facilitates scientific exchange across AI. AAAI-24 will include technical paper presentations, special tracks, invited speakers, workshops, tutorials, poster sessions, competitions, and more.
At PNNL, our team of scientists and engineers utilize their expertise in chemistry, Earth sciences, and data analytics to push the boundaries of scientific exploration and develop innovative solutions to address the most pressing challenges in energy resilience and national security. More recently, PNNL launched the Center for AI, a research agenda that explores the foundations of AI and its future.
PNNL Presentations, Papers, and Workshops
Workshop on Learnable Optimization (LEARNOPT)
PNNL Organizers: Jan Drgona and Draguna Vrabie
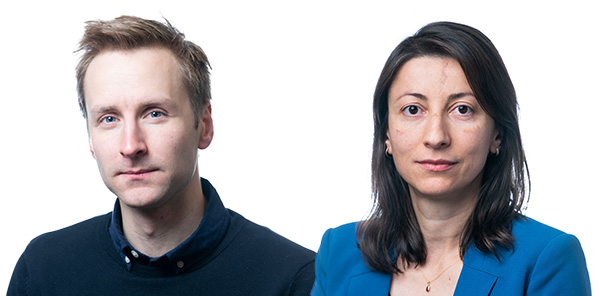
Overview: The AAAI Workshop on Learnable Optimization (LEANOPT) builds on the momentum that has been directed over the past 6 years, in both the operations research (OR) and machine learning (ML) communities, towards establishing modern ML methods as a “first-class citizen” at all levels of the OR toolkit. While much progress has been made, many challenges remain due in part to data uncertainty, the hard constraints inherent to OR problems, and the high stakes involved. LEANOPT will serve as an interdisciplinary forum for researchers in OR and ML to discuss technical issues at this interface and present new ML approaches and software tools that accelerate classical optimization algorithms (e.g., for continuous, combinatorial, mixed-integer, stochastic optimization) as well as novel applications.
Semi-Supervised Learning of Dynamical Systems with Neural Ordinary Differential Equations: A Teacher-Student Model Approach
Accepted Paper
PNNL Author: Mahantesh Halappanavar, Jan Drgona
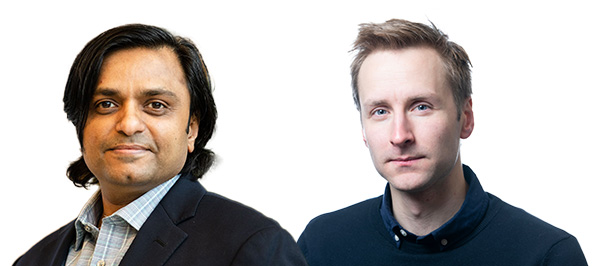
Abstract: Modeling dynamical systems is crucial for a wide range of tasks, but it remains challenging due to complex nonlinear dynamics, limited observations, or lack of prior knowledge. Recently, data-driven approaches, such as Neural Ordinary Differential Equations (NODE), have shown promising results by leveraging the expressive power of neural networks to model unknown dynamics. However, these approaches often suffer from limited labeled training data, leading to poor generalization and suboptimal predictions. On the other hand, semi-supervised algorithms can utilize abundant unlabeled data and have demonstrated good performance in classification and regression tasks. We propose TS-NODE, the first semi-supervised approach to modeling dynamical systems with NODE. TS-NODE explores cheaply generated synthetic pseudo rollouts to broaden exploration in the state space and to tackle the challenges brought by lack of ground-truth system data under a teacher-student model. TS-NODE employs a unified optimization framework that corrects the teacher model based on the student's feedback while mitigating the potential false system dynamics present in pseudo rollouts. TS-NODE demonstrates significant performance improvements over a baseline neural ODE model on multiple dynamical system modeling tasks.
Fortify Your Defenses: Strategic Budget Allocation to Enhance Power Grid Cybersecurity
AI for Cybersecurity (AICS) Workshop
PNNL Organizers: Rounak Meyur, Sumit Purohit, and Braden K. Webb
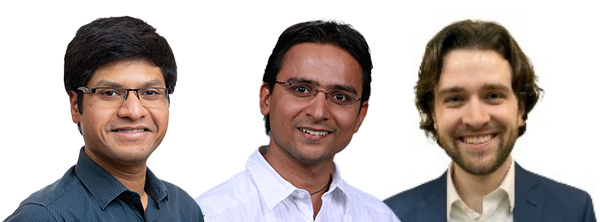
Overview: The abundance of cyber-physical components in the modern-day power grid, with its diverse hardware and software vulnerabilities, has made it difficult to protect it from advanced persistent threats. An attack graph depicting the propagation of potential cyber-attack sequences from the initial access point to the end objective is vital to identify critical weaknesses of any cyber-physical system. A cybersecurity personnel can accordingly plan preventive mitigation measures for the identified weaknesses addressing the cyber-attack sequences. However, limitations on available cybersecurity budget restrict the choice of mitigation measures. We address this aspect through our framework, which solves the following problem: given potential cyber-attack sequences for a cyber-physical component in the power grid, find the optimal manner to allocate an available budget to implement necessary preventive mitigation measures. We formulate the problem as a mixed integer linear program to identify the optimal budget partition and set of mitigation measures which minimize the vulnerability of cyber-physical components to potential attack sequences. We assume that the allocation of budget affects the efficacy of the mitigation measures. We show how altering the budget allocation for tasks, such as asset management, cybersecurity infrastructure improvement, incident response planning, and employee training affects the choice of the optimal set of preventive mitigation measures and modifies the associated cybersecurity risk. The proposed framework can be used by cyber policymakers and system owners to allocate optimal budgets for various tasks required to improve the overall security of a cyber-physical system.
ATLANTIC: Structure-Aware Retrieval-Augmented Language Model for Interdisciplinary Science
Accepted Paper
PNNL Authors: Sai Munikoti, Anurag Acharya, Sridevi Wagle, and Sameera Horawalavithana
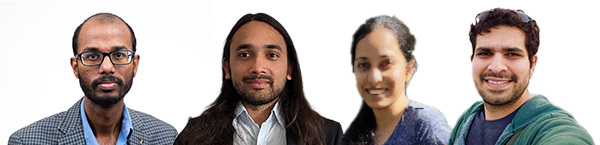
Abstract: Large language models (LLM) record impressive performance on many natural language processing tasks. However, their knowledge capacity is limited to the pretraining corpus. Retrieval augmentation offers an effective solution by retrieving context from external knowledge sources to complement the language model. However, existing retrieval augmentation techniques ignore the structural relationships between these documents. Furthermore, retrieval models are not explored much in scientific tasks, especially in regard to the faithfulness of retrieved documents. In this paper, we propose a novel structure-aware retrieval augmented language model that accommodates document structure during retrieval augmentation. We create a heterogeneous document graph capturing multiple types of relationships (e.g., citation, co-authorship, etc.) that connect documents from more than 15 scientific disciplines (e.g., physics, medicine, chemistry, etc.). We train a graph neural network on the curated document graph to act as a structural encoder for the corresponding passages retrieved during the model pretraining. Particularly, along with text embeddings of the retrieved passages, we obtain structural embeddings of the documents (passages) and fuse them together before feeding them to the language model. We evaluate our model extensively on various scientific benchmarks that include science question-answering and scientific document classification tasks. Experimental results demonstrate that structure-aware retrieval improves retrieving more coherent, faithful, and contextually relevant passages, while showing a comparable performance in the overall accuracy.
Empirical Evaluation of Uncertainty Quantification in Retrieval-Augmented Language Models for Science
Accepted Paper
PNNL Authors: Sridevi Wagle, Sai Munikoti, Anurag Acharya, Sara Smith, and Sameera Horawalavithana
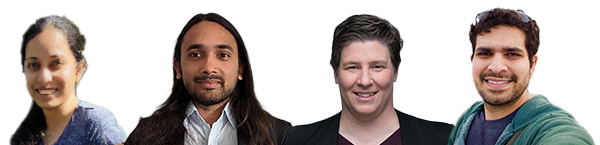
Abstract: LLMs have shown remarkable achievements in natural language processing tasks, producing high-quality outputs. However, LLMs still exhibit limitations, including the generation of factually incorrect information. In safety-critical applications, it is important to assess the confidence of LLM-generated content to make informed decisions. Retrieval augmented language models (RALMs) is relatively a new area of research in natural language processing. RALMs offer potential benefits for scientific natural language processing tasks, as retrieved documents can serve as evidence to support model-generated content. This inclusion of evidence enhances trustworthiness, as users can verify and explore the retrieved documents to validate model outputs. Quantifying uncertainty in RALM generations further improves trustworthiness, with retrieved text and confidence scores contributing to a comprehensive and reliable model for scientific applications. However, there is limited to no research on UQ for RALMs, particularly in scientific contexts. This study aims to address this gap by conducting a comprehensive evaluation of UQ in RALMs, focusing on scientific tasks. This research investigates how uncertainty scores vary when scientific knowledge is incorporated as pretraining and retrieval data and explores the relationship between uncertainty scores and the accuracy of model-generated outputs. We observe that an existing RALM fine-tuned with scientific knowledge as the retrieval data tends to be more confident in generating predictions compared to the model pretrained only with scientific knowledge. We also found that RALMs are overconfident in their predictions, making inaccurate predictions more confidently than accurate ones. Scientific knowledge provided either as pretraining or retrieval corpus does not help alleviate this issue.
Workshop on AAAI: Artificial Intelligence for Cyber Security (AICS)
PNNL Program Committee and Reviewer: Luke Richards
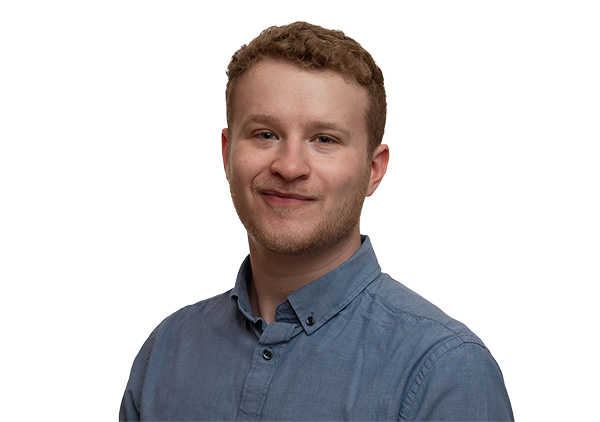
Workshop overview: The workshop will focus on the application of AI to problems in cybersecurity. While AI and ML have shown astounding ability to automatically analyze and classify large amounts of data in complex scenarios, the techniques are not still widely adopted in real-world security settings, especially in cyber systems. The workshop will address technologies and their applications in security, such as ML, game theory, natural language processing, knowledge representation, automated and assistive reasoning, and human machine interactions. This year the workshop emphasis will be on applications of generative AI, including LLMs, to cybersecurity problems as well as adversarial attacks on such models.
GLaM: Fine-Tuning Large Language Models for Domain Knowledge Graph Alignment via Neighborhood Partitioning and Generative Subgraph Encoding
Accepted Paper
PNNL Authors: Khushbu Agarwal, Stefan Dernbach, Alejandro Michel Zuniga, Michael Henry, and Sutanay Choudhury
Abstract: Integrating large language models (LLMs) with knowledge graphs derived from domain-specific data represents an important advancement towards more powerful and factual reasoning. As these models grow more capable, it is crucial to enable them to perform multi-step inferences over real-world knowledge graphs while minimizing hallucination. While large language models excel at conversation and text generation, their ability to reason over domain-specialized graphs of interconnected entities remains limited. For example, can we query a LLM to identify the optimal contact in a professional network for a specific goal, based on relationships and attributes in a private database? The answer is no – such capabilities lie beyond current methods. However, this question underscores a critical technical gap that must be addressed. Many high-value applications in areas such as science, security, and e-commerce rely on proprietary knowledge graphs encoding unique structures, relationships, and logical constraints. We introduce a fine-tuning framework for developing \textbf{G}raph-aligned \textbf{LA}nguage \textbf{M}odels ($\ourSystem$) that transforms a knowledge graph into an alternate text representation with labeled question-answer pairs. We demonstrate that grounding the models in specific graph-based knowledge expands the models’ capacity for structure-based reasoning. Our methodology leverages the large-language model's generative capabilities to create the dataset and proposes an efficient alternate to retrieval-augmented generation styled methods.