Machine Learning Assesses Climate Variability, Reconciles Arctic Amplification Discrepancy
Accounting for natural climate variability using machine learning reconciles the model-versus-observation discrepancy in Arctic amplification from 1980-2022
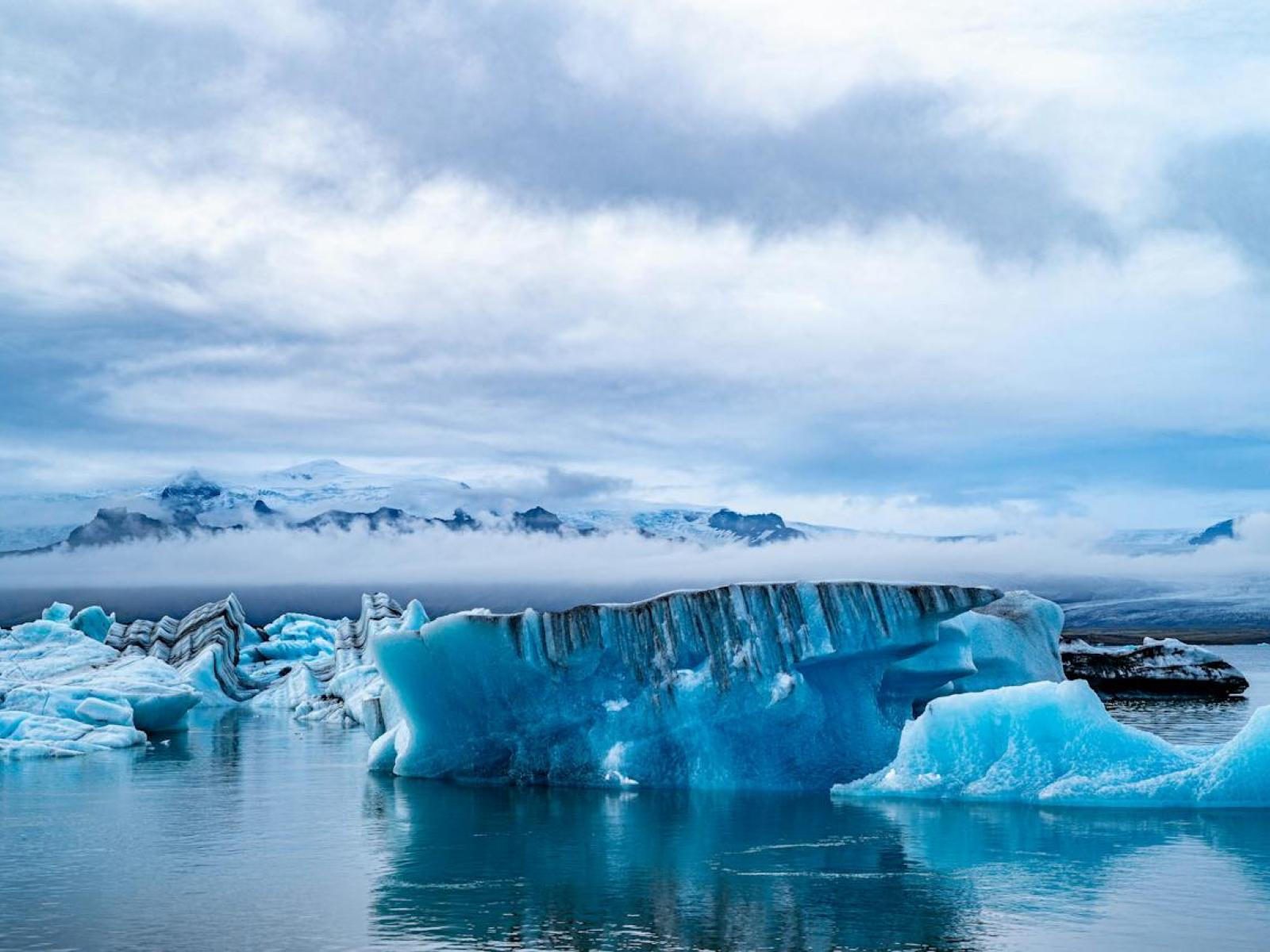
A machine learning technique allowed researchers to disentangle the contributions of external forcing and internal variability on global and Arctic warming.
(Image by David Stanfield | Pexels)
The Science
The Arctic has warmed faster than any region on Earth. Identifying the causes of this warming is critical for understanding climate change. Scientists applied a machine learning approach to quantify the influence of internal climate variability on Arctic amplification (AA) – the ratio of Arctic to global surface warming. The researchers found that natural fluctuations in the climate system that arise from internal variability have reduced global warming and accelerated Arctic warming, inflating AA, which causes disagreements between climate models and observations.
The Impact
The results of this work can help researchers understand this remarkable AA observed over 1980-2022, which exceeds AA in most climate model simulations. Separating out internal variability with a machine learning method brings simulated and observed AA into good agreement.
Summary
Observations show the Arctic warming four times as quickly as the global mean during the satellite era (1980 to present). AA is a robust feature in state-of-the-art climate models, but model simulations rarely reproduce the observed levels of AA for 1980-2022. Observations of AA yield a ratio of Arctic to global surface warming of 3.9 to 4.6, which exceeds the magnitude of AA in over 90% of model ensemble members (ranging from 2.1 to 3.6). This discrepancy between models and observations raises a concern about the climate models’ capability to capture the response of the Arctic and/or global climate to external forcings. AA is a result of external forcing (e.g., from human-induced greenhouse gas emissions), but can vary widely because of multidecadal internal variability. Researchers applied a machine learning technique to disentangle the contributions of external forcing and internal variability on global and Arctic warming. After demonstrating that the method can accurately estimate the magnitude of externally forced warming and internal variability in climate models, scientists applied the approach to observational data and determined that internal variability simultaneously reduced global warming while accelerating warming in the Arctic. This manifestation of internal variability effectively increased AA. When the effect of natural, multidecadal variability is removed from the data, observations and model simulations show excellent agreement on the AA attributable to external forcing impacts.
PNNL Contact
Hailong Wang
Pacific Northwest National Laboratory
hailong.wang@pnnl.gov
Funding
This research was supported by the U.S. Department of Energy (DOE), Office of Science, Office of Biological and Environmental Research, Regional and Global Model Analysis (RGMA) program area, as part of the HiLAT-RASM project. This research was also supported by the NASA FINESST Grant, NSF Grant, and the Calvin Professorship in Atmospheric Sciences. S.P.-C was supported through the PCMDI Project, which is funded by the RGMA program area of the Office of Science at DOE. M. Wang is funded with support of the Arctic Research Program of the NOAA Global Ocean Monitoring and Observing (GOMO) office through the Cooperative Institute for Climate, Ocean, and Ecosystem Studies (CICOES). High-performance computing calculations utilized Cheyenne (https://doi.org/10.5065/D6RX99HX) provided by NCAR’s Computational and Information Systems Laboratory, which is sponsored by the National Science Foundation.
Published: January 29, 2024
Sweeney, A. J., Fu, Q., Po-Chedley, S., Wang, H., & Wang, M. “Internal variability increased Arctic amplification during 1980–2022.” Geophysical Research Letters, 50, e2023GL106060 (2023). [DOI: 10.1029/2023GL10]