Embedding a Physics Informed Deep Learning Model in a Chemical Transport Model
A deep learning model overcomes persistent challenges in emulating long-term simulations of secondary organic aerosols
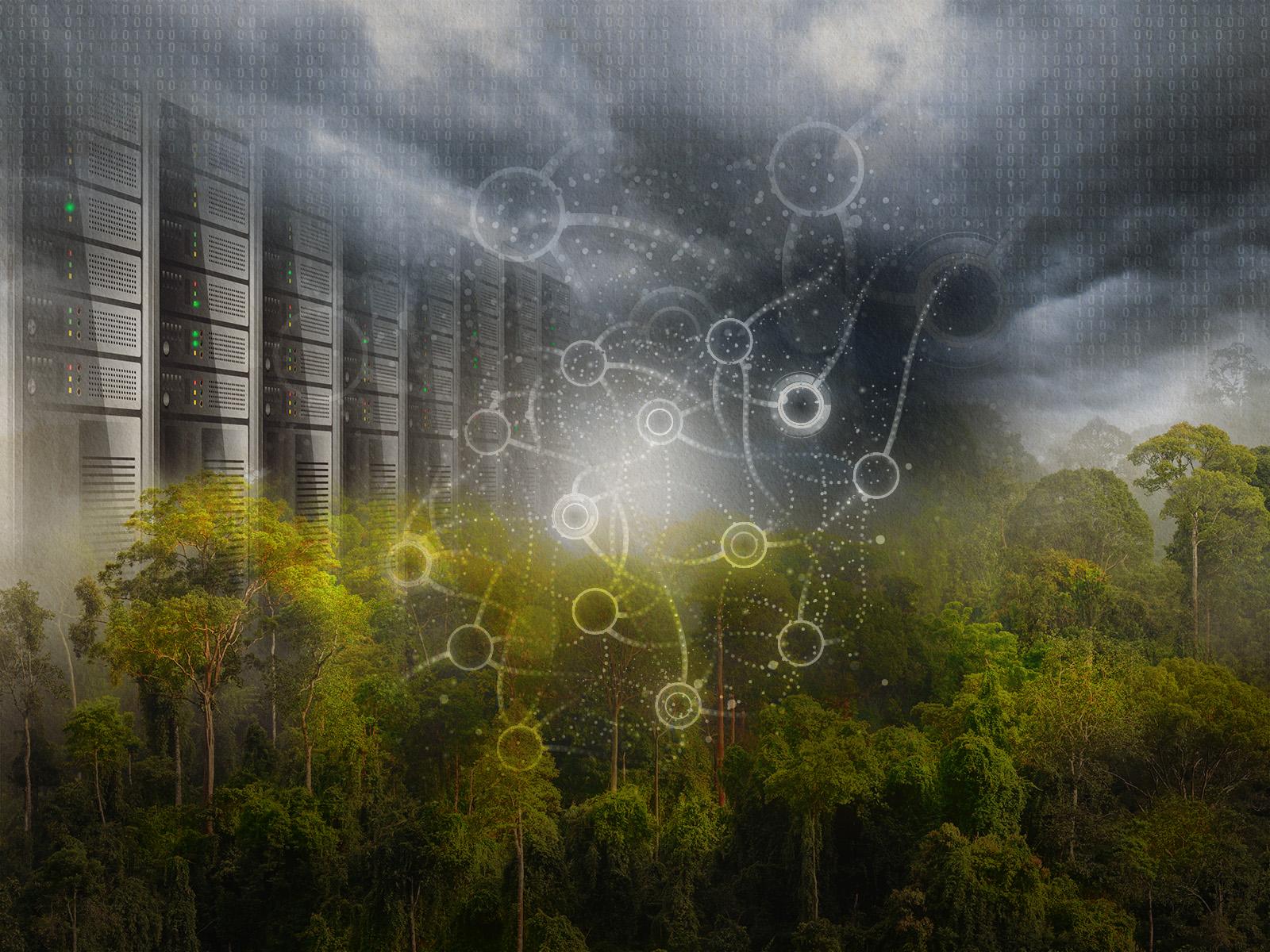
Researchers trained a deep learning neural network using a dataset of just seven hours of size-dependent isoprene epoxydiol secondary organic aerosol fluxes over the Amazon. The trained module can reproduce simulations over an extended testing time without accumulating errors.
(Image by Melanie Hess-Robinson | Pacific Northwest National Laboratory)
The Science
Secondary organic aerosols (SOA) are fine particles in the atmosphere that interact with clouds and radiation and affect Earth’s energy budget. SOA formation involves chemistry in gases, aqueous aerosols, and clouds. Due to the widely varying chemistry timescales and multidimensional nature of SOA systems, simulating these aerosol processes is computationally expensive. Deep neural networks (DNNs) could be used to represent these complex processes; however, multiple challenges have limited their use in atmospheric chemistry. New work overcomes many of these challenges using a physics-informed DNN that employs SOA physics and chemistry knowledge in feature selection. Research demonstrates the DNN’s applicability for emulating the multiphase chemistry of isoprene epoxydiol SOA (IEPOX-SOA) in a regional model over the Amazon rainforest.
The Impact
Researchers showed that a physics-informed DNN embedded in a detailed regional model successfully emulates the complex multiphase chemistry of IEPOX-SOA for the first time. The model generalizes well over multiple days of simulations, satisfies mass balance, and predicts IEPOX-SOA in 20 aerosol size sections. Previous models had only been applied to much simpler aerosol distributions (less than eight modes or size sections). This work provides a clear proof of concept for successfully implementing machine learning algorithms to speed up complex physics and chemistry calculations in three-dimensional chemical transport and climate models.
Summary
Using knowledge of the multiphase chemistry of IEPOX-SOA, researchers developed and trained a physics-informed DNN to emulate the entire size distribution of IEPOX-SOA over the Amazon. The DNN can successfully simulate the complex functional dependence of IEPOX-SOA formation on the composition of the inorganic aerosol (sulfate, ammonium, nitrate), acidity, particle water, and diffusion limitations in the organic shell. These parameters are varied spatially and temporally as a function of meteorology (temperature, relative humidity) and chemistry. By training the DNN to predict fluxes of IEPOX-SOA converting to particles rather than concentrations, the model could address the challenge of maintaining mass balance. Researchers overcame another obstacle related to the skewed and sparse input and output distributions in the training data for the DNN. They applied an inverse hyperbolic sine transform to data distributions. Trained with just seven hours of Weather Research and Forecasting Model coupled to chemistry (WRF-Chem) simulations, the DNN predictions generalize well over several days of simulations in both the dry and wet seasons of the Amazon. The embedded DNN reduces the computational expense of WRF-Chem by a factor of two. The approach shows substantial promise for application to computationally expensive chemistry solvers in climate models.
PNNL Contact
Manish Shrivastava, Pacific Northwest National Laboratory, manishkumar.shrivastava@pnnl.gov
Funding
This research was supported by the Department of Energy (DOE), Office of Science, Biological and Environmental Research (BER) program through the Early Career Research Program. Computational resources for the simulations were provided by PNNL Institutional Computing and EMSL, the Environmental Molecular Sciences Laboratory (a DOE Office of Science User Facility sponsored by the Office of Biological and Environmental Research located at PNNL). This research also used resources of the Argonne Leadership Computing Facility, which is a DOE Office of Science User Facility.
Published: May 1, 2023
Sharma H., Shrivastava M., and Singh B. 2023. “Physics informed deep neural network as aerosol emulator embedded in a regional chemical transport model,” npj Climate and Atmospheric Sciences, 6, 28. [DOI: 10.1038/s41612-023-00353-y]