Scientists Use Machine Learning to Analyze and Explore How Biological Cells Operate
New research demonstrates physical principles can predict the control of metabolism
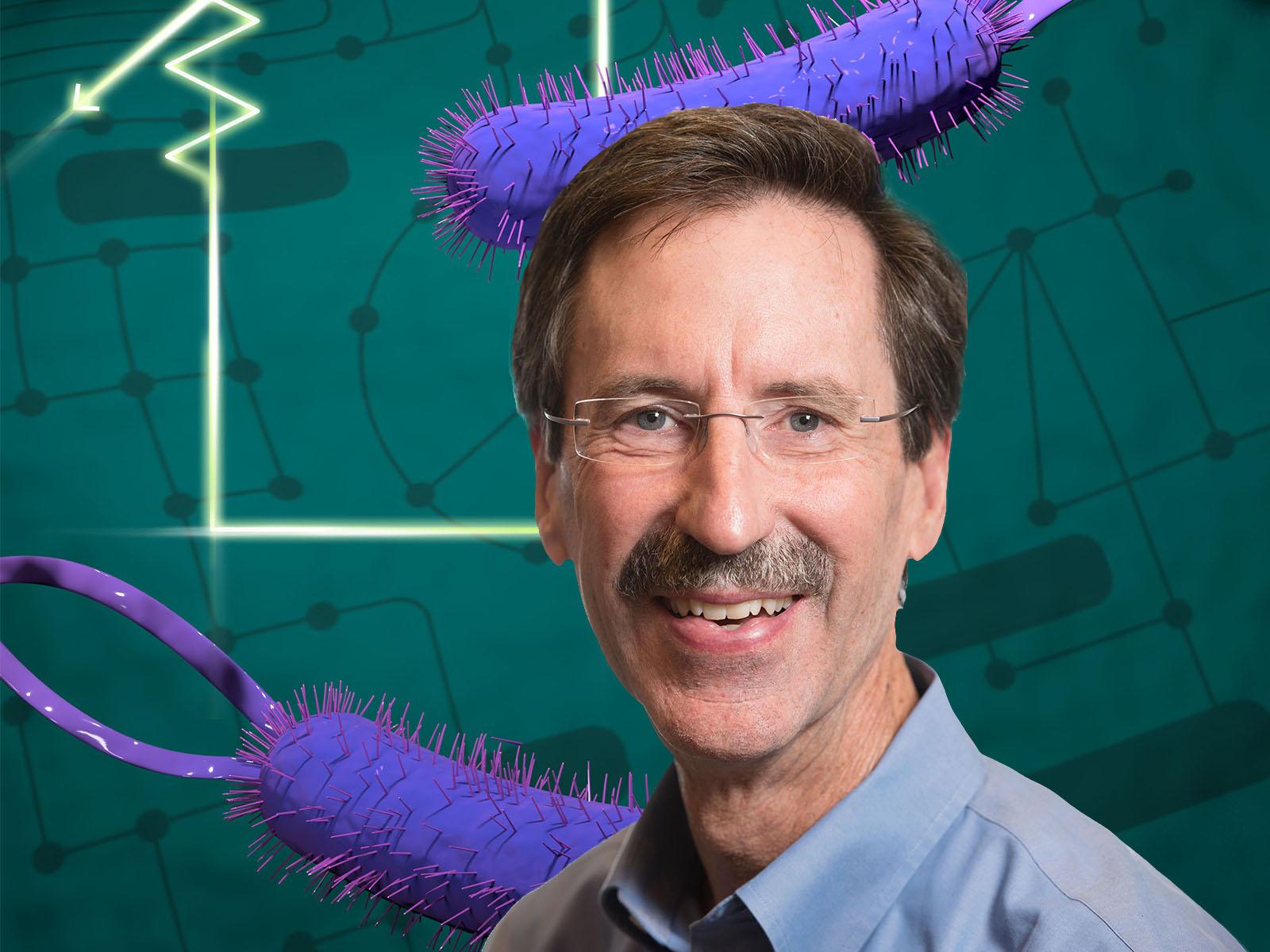
Pacific Northwest National Laboratory researcher William Cannon's new research demonstrates that the control of metabolism can be predicted from physical principles, providing new insights into biological processes.
(Image composite by Shannon Colson | Pacific Northwest National Laboratory)
Cells are the building blocks of life, but scientists still do not know exactly how they work. Pacific Northwest National Laboratory researcher William Cannon and University of California, Riverside’s Mark Alber and Samuel Britton are studying the chemical reactions involved in cellular metabolism, the process that regulates life in living things.
“We understand tornadoes and how the warm air mixes with the cool air to create winds,” said Cannon. “Inside cells, there are molecules that are essentially spinning like tornadoes. The issue is that the cell, like a tornado, can only exist under certain conditions and the cell needs to be able to control those conditions. We want to understand how the cells regulate the enzymes that are responsible for the reactions and cause metabolism to work.”
The research team investigated two hypotheses from the late 1960s that postulated that regulation in metabolism has two purposes: to control the metabolites, and to maintain energy flow inside the cells. Until now, the first hypothesis that cells regulate the enzymes to control common biochemical pathways had not been proven with experiments or computer simulations.
The scientists used a combination of statistical thermodynamics, control theory, and computational mathematics to predict which enzymes needed to be controlled to match physiological levels of metabolite concentrations. Cannon, who holds a joint appointment at UC Riverside and has a background in chemistry, biophysics, and physics, said, “Computational mathematics is the most exciting work. In experimental work, you can only study what the instrument can measure—which often isn’t a direct measure of the phenomena you want to understand. You can build models from first principles to really understand how things work.”
Reinforcement learning is a trial-and-error approach
Cannon’s research team used two methods to control the concentrations by regulating the reactions. The deterministic method relies on statistical thermodynamics and control theory. This approach assumes that each time a reaction occurs, it will yield the same results. The second method relies on statistical thermodynamics and control theory but also uses reinforcement learning, a type of machine learning (ML). While it takes longer to run and reach a solution, reinforcement learning can get around bumps and barriers.
Using the mathematical and computational models, the scientists found that the cells were able to control the conditions “so that the interior did not become thick and gooey like molasses,” said Cannon. They found that the cells regulate by controlling the metabolite concentrations, and their ML approach was highly accurate.
No other approach has been shown to rapidly identify points of metabolic regulation
In a study published in the Journal of the Royal Society Interface, Cannon and his team found that the first part of the original hypotheses was true. “The regulation actually causes the reactions to be much further from equilibrium. The nonequilibrium nature of a regulated reaction is due to the regulation; the regulation is not caused by the nonequilibrium nature,” Cannon said.
For the second phase of the research, the team is trying the same ML approach on a system with close to a thirtyfold increase in reactions. They expect the second method will perform even better.
This study was part of a three-year, $2.1 million grant from the U.S. Department of Energy, Office of Science Graduate Student Research award and the Biological and Environmental Research program; the National Institute of Biomedical Imaging and Bioengineering; and the National Science Foundation. Read more about this research here.
Learning how cells regulate and control themselves is essential to advance in important research areas, such as medicine, fundamental science, and synthetic biology, “We can predict how to engineer cells to do things we want them to do. We can predict what will happen if we change the environment that they are in. We can model them, and those models will help us guide experimental engineering efforts, like converting plants into biofuel,” said Cannon.
Published: May 7, 2021