PNNL Partners with Oak Ridge on AI Project
Mahantesh Halappanavar, along with six colleagues, to lead PNNL's contribution, part of a $16 million U.S. Department of Energy program
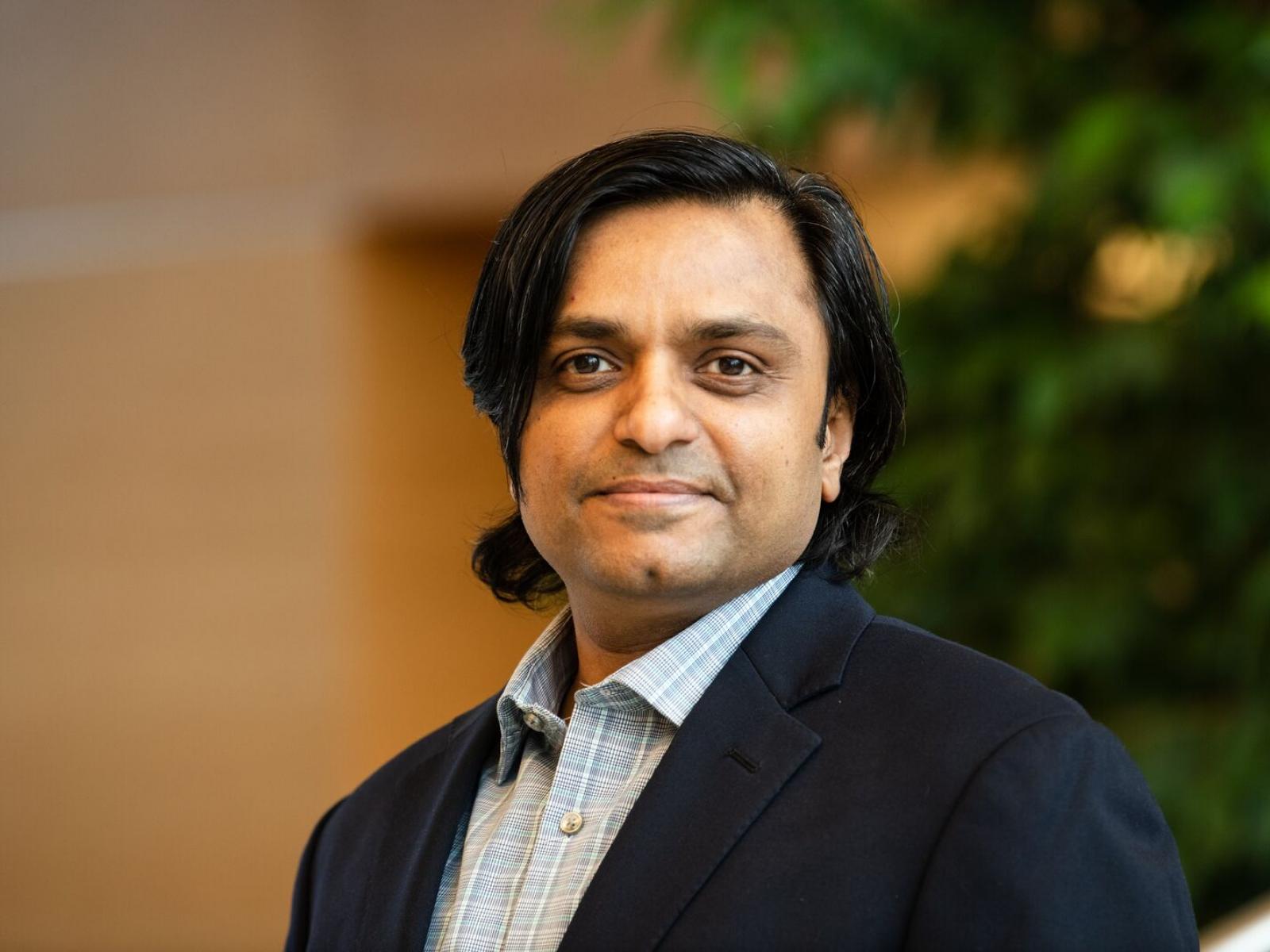
Mahantesh Halappanavar, the acting group leader for the PNNL Data Sciences & Machine Intelligence group.
(Photo by Andrea Starr | Pacific Northwest National Laboratory)
Pacific Northwest National Laboratory (PNNL) is partnering with Oak Ridge National Laboratory (ORNL) to apply artificial intelligence (AI) to improve complex systems, including improving heating and cooling in buildings or reconfiguring electrical grid efficiencies.
“It was an extremely competitive process for the participating labs, starting with an initial screening process, selections of finalists, and another screening process,” said PNNL computer scientist Mahantesh Halappanavar, who is leading PNNL’s partnership with ORNL. “I would say it was recognized that we have a very good team at PNNL.” The PNNL team also includes Vikas Chandan, Sam Chatterjee, Jan Drgona, Sayak Mukherjee, Malachi Schram, and Ramakrishna Tipireddy.
The partnership is part of a U.S. Department of Energy (DOE) Office of Science $4.2 million, three-year grant for the project “DnC2S: Decision and Control of Complex Systems: A Data‐Driven Framework.” Arizona State University and the University of California, Santa Barbara are also participating in the project.
Research within the project is divided among four interdependent focus areas—model construction, uncertainty quantification, decision and control, and continual learning—that each have particular desired outcomes. Yet, taken together, they assure the success of the overall effort. A fifth cross-cutting area known as application demonstration will validate results from the individual efforts and apply these findings, via proof-of-concept demonstrations, to a range of areas including building energy efficiency, reactor power control, and science user facility control.
The award represents a portion of the $16 million that DOE announced last year to provide for advanced research in machine learning (ML) and AI for both scientific investigation and the management of complex systems.
The project will strive to develop a unified theoretical foundation based on probabilistic graphical models, or PGMs. Explaining PNNL’s role, Halappanavar, the acting group leader for the PNNL Data Sciences & Machine Intelligence group, said, “These data systems that we are exploring are very complex. Even though we have good mathematical models, we cannot understand everything just with models. So, we are going to depend quite a bit on the data—creating data sets crafted through ML that we manage to build new mathematics. That's the overarching theme to develop new mathematical methods.”
PNNL researchers will work with Frank Liu, ORNL’s principal investigator and a distinguished scientist.
“The goal of this project is to develop a data-driven approach to model construction for complex systems, with the final objective of applying AI and ML techniques for more advanced and robust decision and control of these complex systems,” said Liu. “The project will build on the previous work at both ORNL and PNNL, and develop new foundational work and solutions for complex systems in both science and engineering.”
Schram, a senior physicist and team leader in the Data Sciences & Machine Intelligence group, said the research has the potential to benefit real-world applications, such as energy usage in buildings, electrical grid management, and experimental high-energy physics facilities.
“We would want to collect as much data as we can from a building,” Schram said. “We determine how much energy is it consuming at a given point of the day and, eventually, determine patterns through the years—across multiple years. With this huge amount of data, we could then go back, and question how does it correlate with weather? How does it correlate with the occupancy? How does it correlate with a host of external factors, such as between different building types?”
Ultimately, building usage data can provide feedback that contributes to more efficient electrical grid management, Schram said.
Added Drgona, a PNNL computer scientist, “We expect to be able to design control systems that are far more accurate when they have been designed with deep learning techniques. And these systems would be consistently evolving because it’s never complete.”
Chatterjee, a senior data and operations research scientist and team leader in the Data Sciences & Machine Intelligence group, said, “Our data-trained models, using deep learning techniques, will always have uncertainties associated with them. These uncertainties, in fact, would be part of our AI model.”
Halappanavar noted the wide-ranging expertise of team members.
“By bringing together a diverse team of mathematicians, computer scientists, and subject matter experts,” said Halappanavar, “the project aims to not only contribute to theoretical foundations of AI driving the control of complex systems, but also on specific application use cases considered in the project.”
Published: January 22, 2021