Using Explainable AI to Understand Key Wildfire Controls
Researchers seek a deeper understanding of when, where, and why large wildfires occur using explainable artificial intelligence
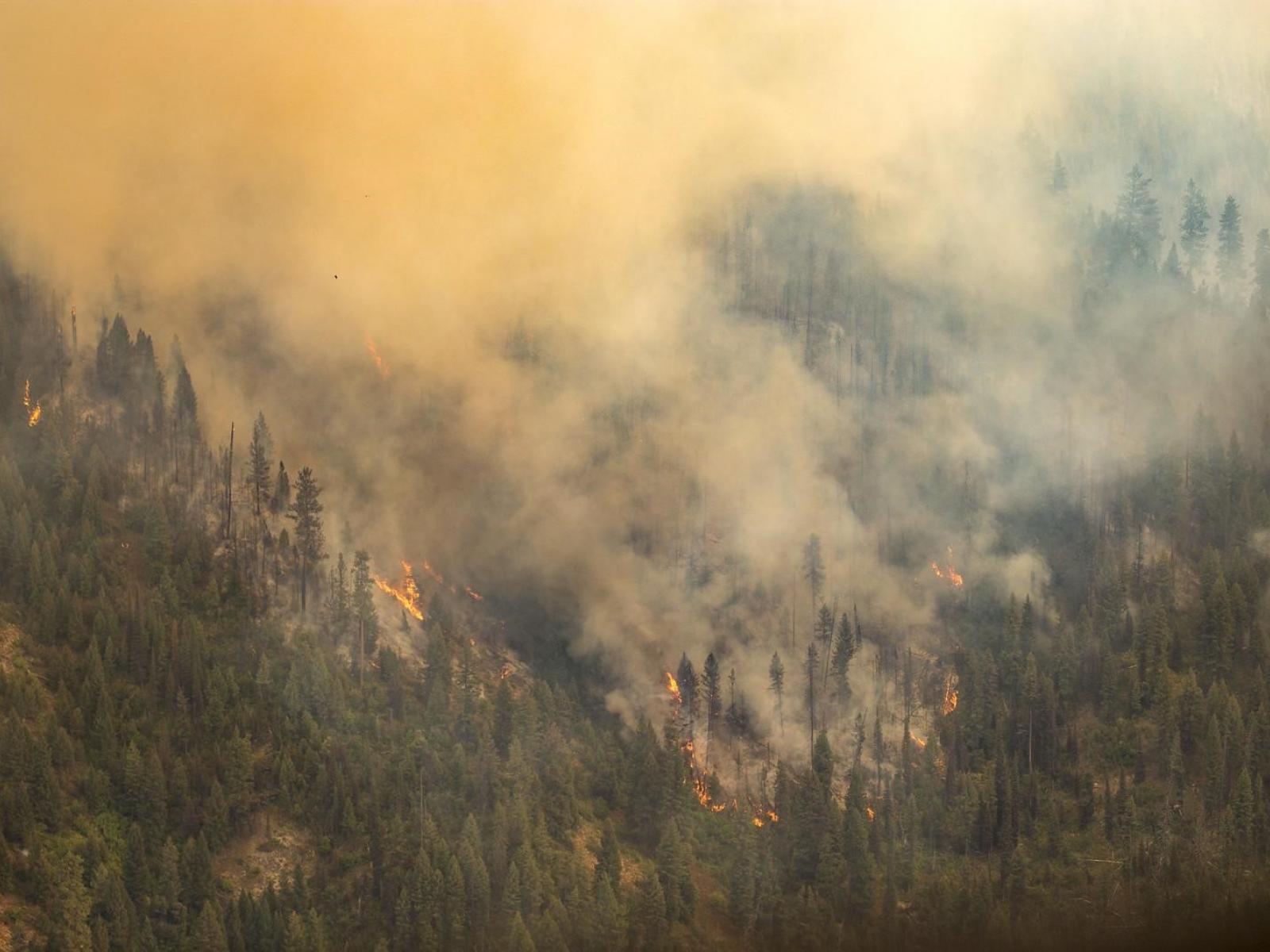
Explainable AI allows researchers to predict wildfires and explain the most important factors that drive the size of the overall burned area.
(Photo: Forest Service, USDA | Flickr.com)
The Science
Artificial intelligence (AI) refers to a computer simulating human intelligence, solving complex problems and predicting unknowns. Although AI is powerful, it has a reputation as a “black box” with inscrutable connections between the inputs and outputs. This study used explainable AI, a set of tools or framework that helps researchers understand and interpret predictions made by machine learning models, to predict wildfires across the U.S. and identify key wildfire predictors in different regions. Scientists found that fuel dryness is important to large wildfires in the western U.S., while a dry winter induced by anomalous large-scale meteorological patterns is critical to large fires in the southeastern U.S.
The Impact
The intensity and frequency of wildfires have significantly increased in recent decades across the U.S. Explainable AI can be used to improve prediction of wildfires and explain the key factors controlling burned areas, which can guide monitoring and prevention efforts. Importantly, explainable AI identified large-scale meteorological patterns as an important predictor of wildfires in the U.S. for the first time. This suggests an additional pathway, besides changes in temperature, drought, and fuel moisture, for climate change to affect wildfires in the contiguous U.S. The machine learning model developed in this study will help project future fire activity and understand its impacts on air quality and human health.
Summary
Wildfire burned areas in the U.S. have increased tenfold since the 1980s, posing greater threats to property, human life, and air quality. A route to improving wildfire controls involves more accurate predictions and a better understanding of what factors determine how much area wildfires burn. Researchers built a machine learning model and incorporated a game-theory-based interpretation to understand the complex relationships between wildfire and multiple factors, including meteorology, vegetation, landscape, and human impacts. The results showed that including predictors representing large-scale meteorological patterns favorable for wildfires significantly improves burned area predictions. Researchers used the novel interpretation method to identify the role of coordinate variables (i.e., geographical locations) in distinguishing fire regimes with different temporal patterns of burned areas. They also examined the physical relationships between local meteorology and burned areas. They found that fuel dryness is the most important predictor of large burned areas in the western U.S., while the large-scale meteorological patterns featuring dry winters contribute more in the southeastern U.S. This study better elucidates the complex processes contributing to wildfires using machine learning tools and a game theory interpretation.
PNNL Contact
Ruby Leung, Pacific Northwest National Laboratory, Ruby.Leung@pnnl.gov
Funding
This work was partly supported by the U.S. Department of Energy’s Office of Science Biological and Environmental Research through the Regional and Global Model Analysis and Multisector Dynamics program areas.
Published: July 2, 2021
S. S.-C. Wang, Y. Qian, L. R. Leung & Y. Zhang. “Identifying key drivers of wildfires in the contiguous US using machine learning and game theory interpretation.” Earth’s Future, 9, e2020EF001910, (2021). [DOI: 10.1029/2020EF001910]