Deep Learning Uses Stream Discharge to Estimate Watershed Subsurface Permeability
Researchers used deep learning methods to estimate the subsurface permeability of a watershed from stream discharge measurements
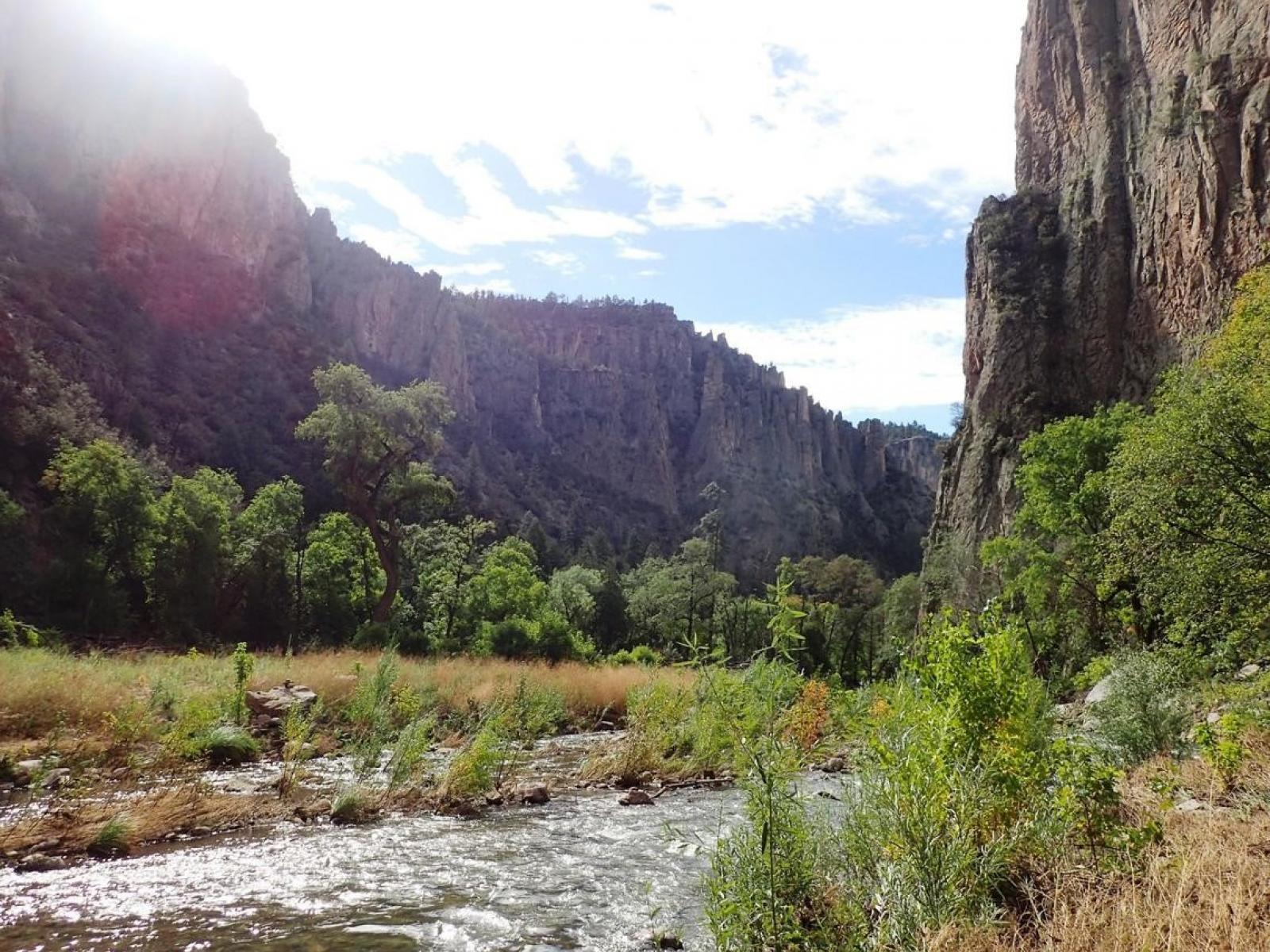
Deep learning improves stream discharge-based estimations of subsurface permeability, allowing for more accurate watershed modeling.
(Image by Alan Cressler | The United States Geological Survey)
The Science
Subsurface permeability, a measure of how well liquids flow through belowground rocks and soils, is a key parameter that determines subsurface flow and transport processes in watersheds. However, permeability is difficult and expensive to measure directly at the scale and resolution required by watershed models. On the other hand, stream flow monitoring data is widely available. The links between permeability and stream flow provide a new route to estimating subsurface permeability. Scientists used deep learning that more accurately estimates the subsurface permeability of a watershed from stream discharge data than is possible with traditional methods. This improvement will help calibrate watershed models and reduce the uncertainty in stream discharge predictability.
The Impact
The deep learning method yielded realistic permeability estimations for a real watershed system, with an improved match between the predicted and observed stream discharges. This work demonstrates that deep learning can be a powerful tool for estimating watershed parameters from indirect but relevant observations. By successfully using deep learning to map the nonlinear relationship between permeability and stream discharge, this work presents new opportunities for improving the subsurface characterization of large-scale watersheds. It paves the way to help develop more generalized watershed model calibration strategies for complex systems that involve multiple parameters and multiple types of observation data.
Summary
Subsurface permeability is a key parameter that controls the contribution of the subsurface flow to stream flows in watershed models. Since directly measuring permeability at the spatial extent and resolution required by watershed models is difficult and expensive, researchers commonly estimate it through inverse modeling. The wide availability of stream surface flow data compared to groundwater monitoring data provides a new data source for integrated surface and subsurface hydrologic models to infer soil and geologic properties.
Scientists trained deep neural networks (DNNs) to estimate subsurface permeability from stream discharge hydrographs. First, the DNNs are trained to map the relationships between the soil and geologic layer permeabilities and the simulated stream discharge obtained from an integrated surface-subsurface hydrologic model of the studied watershed. The DNNs yielded more accurate permeability estimates than the traditional inverse modeling method. The DNNs then estimated the permeability of a real watershed (Rock Creek Catchment in the headwaters of the Colorado River) using observed stream discharge from the study site. The watershed model with permeability estimated by DNNs accurately predicted the stream flows. This research sheds new light on the value of emerging deep learning methods to assist integrated watershed modeling by improving parameter estimation, which will eventually reduce the uncertainty in predictive watershed models.
PNNL Contact
Xingyuan Chen, Pacific Northwest National Laboratory, xingyuan.chen@pnnl.gov
Funding
This work was funded by the ExaSheds project, which was supported by the U.S. Department of Energy, Office of Science, Biological and Environmental Research program, Earth and Environmental Systems Sciences Division, Data Management Program, under Award Number DE-AC02-05CH11231.
Published: May 7, 2021
E. Cromwell, et al., “Estimating Watershed Subsurface Permeability From Stream Discharge Data Using Deep Neural Networks.” Front. Earth Sci. 9, 613011, (2021). [DOI: 10.3389/feart.2021.613011]