Deep Learning to Predict Interspecies Spatial Interactions from Microbial Assembly Patterns
Deep learning enables incorporating microscopic images as a new data source to predict microbial spatial interactions
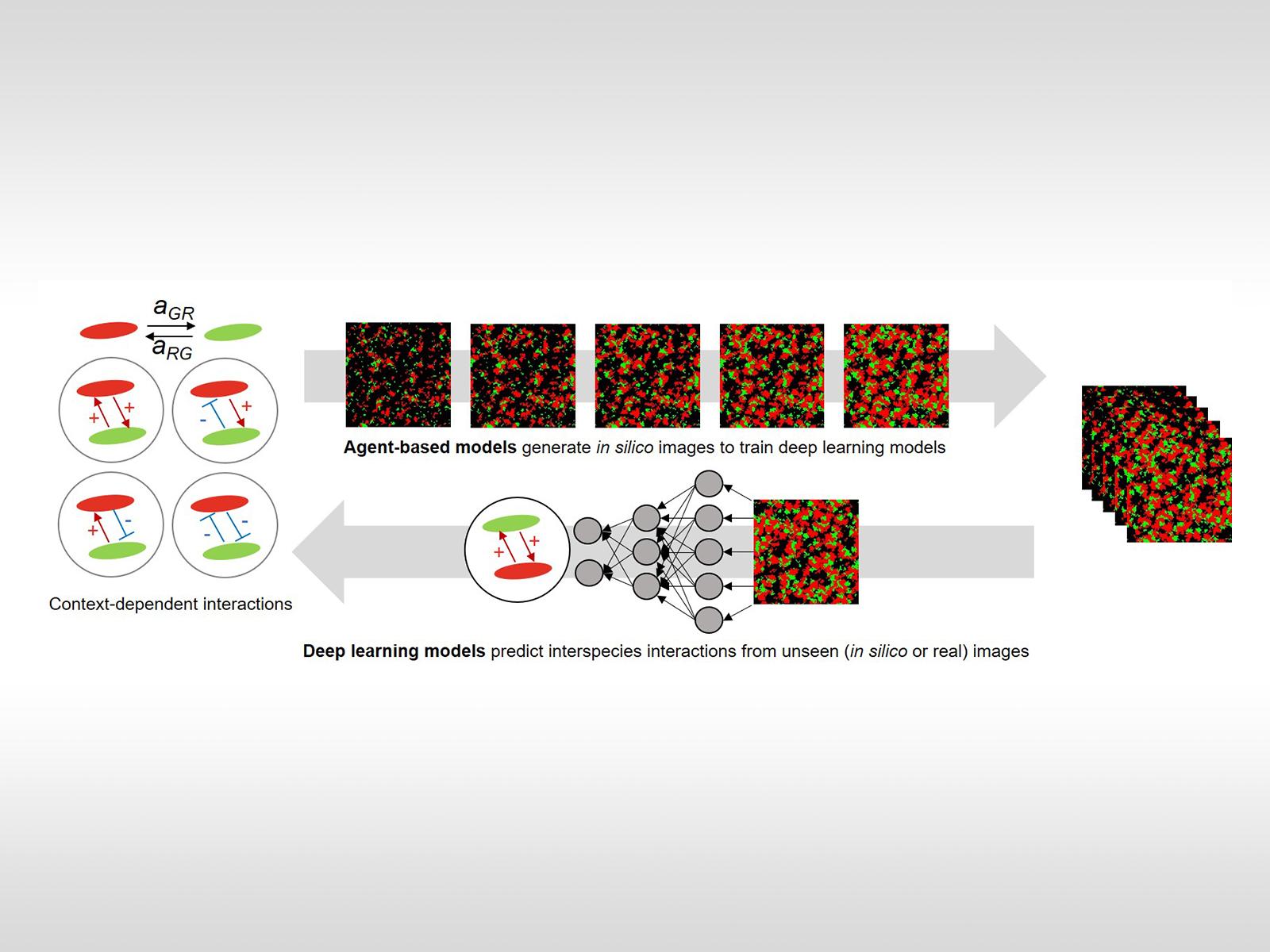
Principles of microbial interactions (left) are integrated with modeling to create simulated fluorescent microscopy images (right). These simulated images are used to train a neural network to predict microbial spatial interactions in novel microscopy images. Image from J.-Y. Lee, et al., "Deep Learning Predicts Microbial Interactions from Self-organized Spatiotemporal Patterns." Computational and Structural Biotechnology Journal 18, 1259-1269 (2020). [DOI: 10.1016/j.csbj.2020.05.023]
The Science
Interactions between different species in a microbial community govern how members self-assemble in specific spatial patterns. However, methods that use physical features of ecological assembly to predict microbial interactions do not exist. Now researchers trained deep neural networks to accurately predict microbial interactions captured by fluorescence microscopy. They trained the networks using data collected experimentally and simulated from modeling.
The Impact
This work shows how data-driven modeling can leverage visualization techniques to tackle key science questions in microbial ecology. The developed deep learning workflow can significantly improve understanding of how microorganisms colonize habitats and interact with each other in spatially varied environments such as soils.
Summary
Rapid advancement in experimental and instrumental technologies is enabling the generation of high-resolution and high-throughput microscopic images that reveal the spatial distribution of microorganisms. These spatial interactions are key to carrying out coordinated metabolic reactions within microbial communities, but the use of spatial patterns for predicting microbial interactions is currently lacking. Conventional population-based computational methods that use species abundance data as a primary input to predict interspecies interactions have yet to be extended to incorporate spatial organizations of microorganisms. To fill this gap, the research team proposed supervised deep learning as a new network inference tool.
Currently, developing deep neural networks directly from experimental microscopy image data is infeasible due to unknown input-output relationships and insufficient amounts of training data. The team overcame these limitations by using high-fidelity agent-based models to perform 5000 simulations of the growth of two interacting microorganisms. This generated usable image data to effectively train deep learning networks. The resulting neural networks accurately predicted microbial interactions and their spatial variations not only from in silico images, but also from actual microscopic images obtained through carefully co-designed experiments. Therefore, the combined use of the agent-based model, machine learning algorithms, and experiments successfully demonstrated how to infer microbial interactions from spatially distributed data. This combination of techniques is a useful tool to reveal key—but previously unknown—interaction mechanisms in complex microbial communities that have been underexplored to date.
PNNL Contacts
Janet K. Jansson, Pacific Northwest National Laboratory, janet.jansson@pnnl.gov
Kirsten Hofmockel, Pacific Northwest National Laboratory, kirsten.hofmockel@pnnl.gov
Funding
This research was supported by the U.S. Department of Energy Office of Science, Biological and Environmental Research Program, and is a contribution of the Scientific Focus Area "Phenotypic response of the soil microbiome to environmental perturbations" at the Pacific Northwest National Laboratory. A portion of the research described in this paper was also performed at EMSL- the Environmental Molecular Sciences Laboratory.
Published: July 23, 2020
J.-Y. Lee, et al., "Deep Learning Predicts Microbial Interactions from Self-organized Spatiotemporal Patterns." Computational and Structural Biotechnology Journal 18, 1259-1269 (2020). [DOI:10.1016/j.csbj.2020.05.023]
This work has been published in Computational and Structural Biotechnology Journal as an invited paper for a special issue organized by the Editor-in-Chief.