Atomic Fortune-Telling: Forecasting the Evolution of Chemical Reactions
A first-of-its-kind predictive model can analyze chemical reactions in an electron microscope
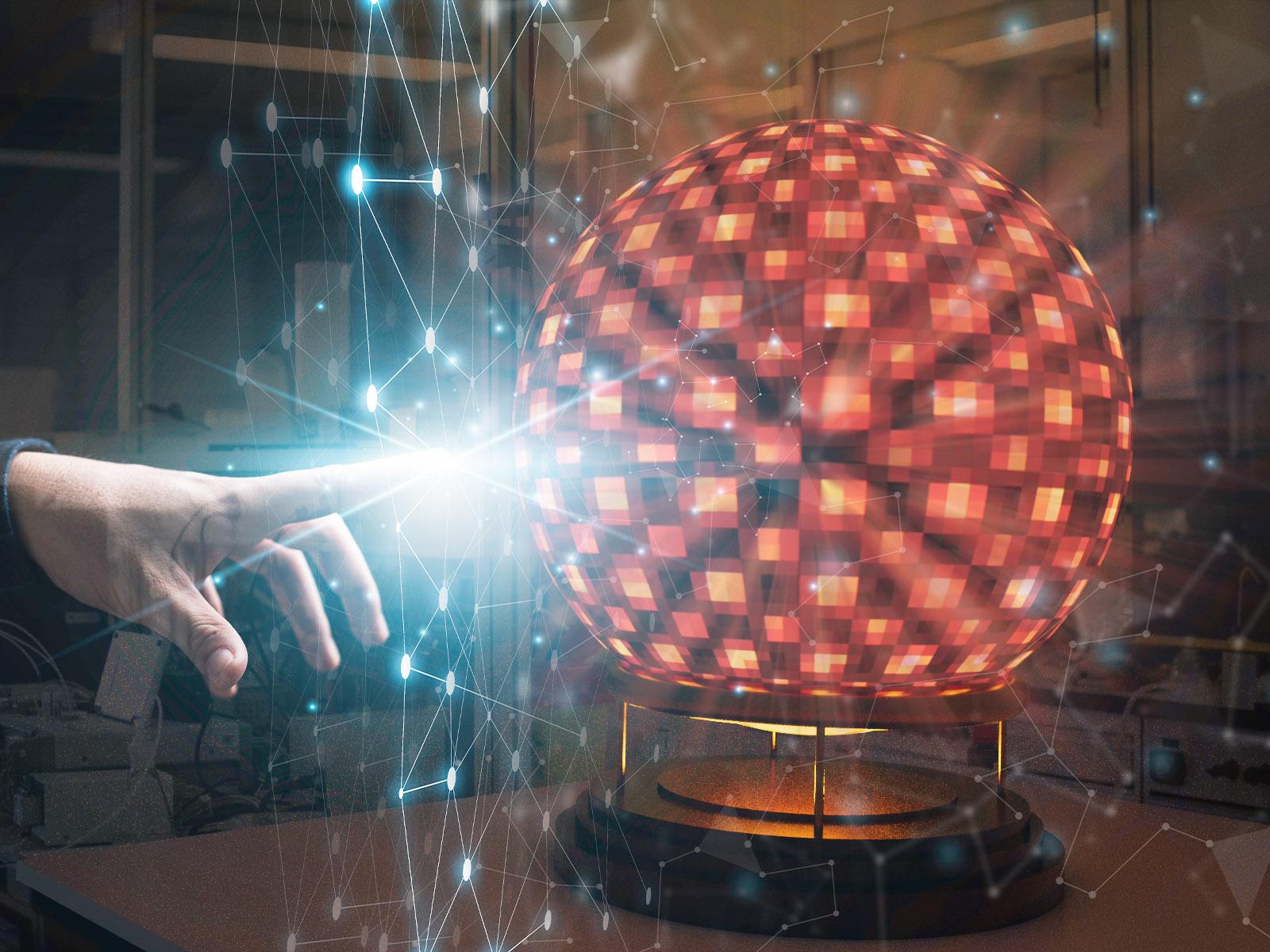
A new machine learning model will help researchers understand how chemical reactions evolve in real time.
(Illustration by Cortland Johnson | Pacific Northwest National Laboratory)
Materials are surrounded by chemical reactions, whether they’re exposed to wind and rain or sealed inside a battery. Chemical reactions are fast and small, making it challenging to watch materials change at an atomic level. Researchers are looking for ways to model processes as they happen, while anticipating the next step.
To do this, scientists can use a type of machine learning model, known as long short-term memory (LSTM), to take already available data and predict what comes next. This model uses previous data to forecast what a system may look like in the future.
A collaboration between Pacific Northwest National Laboratory (PNNL) and the University of Washington (UW) developed a customized LSTM model and applied it to dynamic electron microscopy data for the first time. The team, led by PNNL materials scientist and UW affiliate professor Steven Spurgeon, included students in the UW Data Intensive Research Enabling Clean Technologies (UW-DIRECT) program.
The team studied a specific type of microscopy called electron energy loss spectroscopy, known as EELS. EELS provides rich information about the chemical state and bonding of materials with atomic-level precision. PNNL researchers collected dynamic EELS data on strontium titanium oxide, watching as it changed its chemical state and structure upon exposure to different electron beam environments. It is important for researchers to control these changes because they impact the oxide’s properties for catalysis, electronics, and other applications.
After data collection, the UW students began their work under the guidance of the PNNL team. The DIRECT program focuses on training young scientists and engineers “to apply modern data science tools to the traditional aspects of materials design, synthesis, and characterization.” The group investigated methods for processing the data, structuring the model, and training the model specifically for EELS data.
Modeling data to predict how reactions unfold
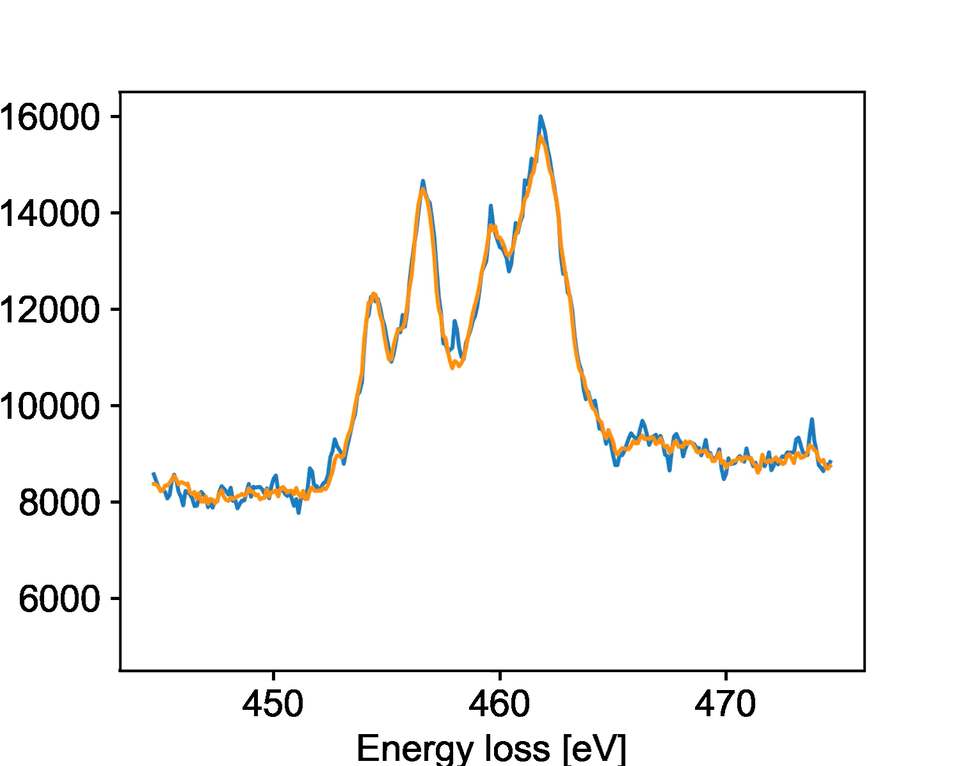
The model specifically focused on identifying when the oxide material would change while being bombarded by electrons. The team developed new solutions to processing the raw data that allowed the model to identify key changes faster than a human could notice. They showed that the model is highly accurate at predicting when a reduction reaction will occur.
While the team trained their model for a specific reaction, the general process should work for other reactions and transformations. Their work describes key aspects of training a machine learning model to work with EELS data. This provides an overview for other researchers exploring different systems and can facilitate easier model development in the future.
Where does Spurgeon see the future of this research? “We’ve begun to see the emergence of artificial intelligence-guided platforms to manipulate matter with incredible precision,” said Spurgeon. “Creating predictive machine learning models represents an important step toward developing autonomous electron microscopes that can both observe and direct materials.”
This research was supported by the Chemical Dynamics Initiative at PNNL. UW-DIRECT is supported by the Clean Energy Institute, the UW eScience Institute, and the National Science Foundation. In addition to Spurgeon, the PNNL authors are Christina Doty, Sarah Akers, and Bethany Matthews. The UW team is Nicholas Lewis, Yicheng Jin, Xiuyu Tang, and Vidit Shah.
Published: December 12, 2022