A machine learning framework for DCAT to assess power grid security for future grid planning
POC: Bharat Vyakaranam
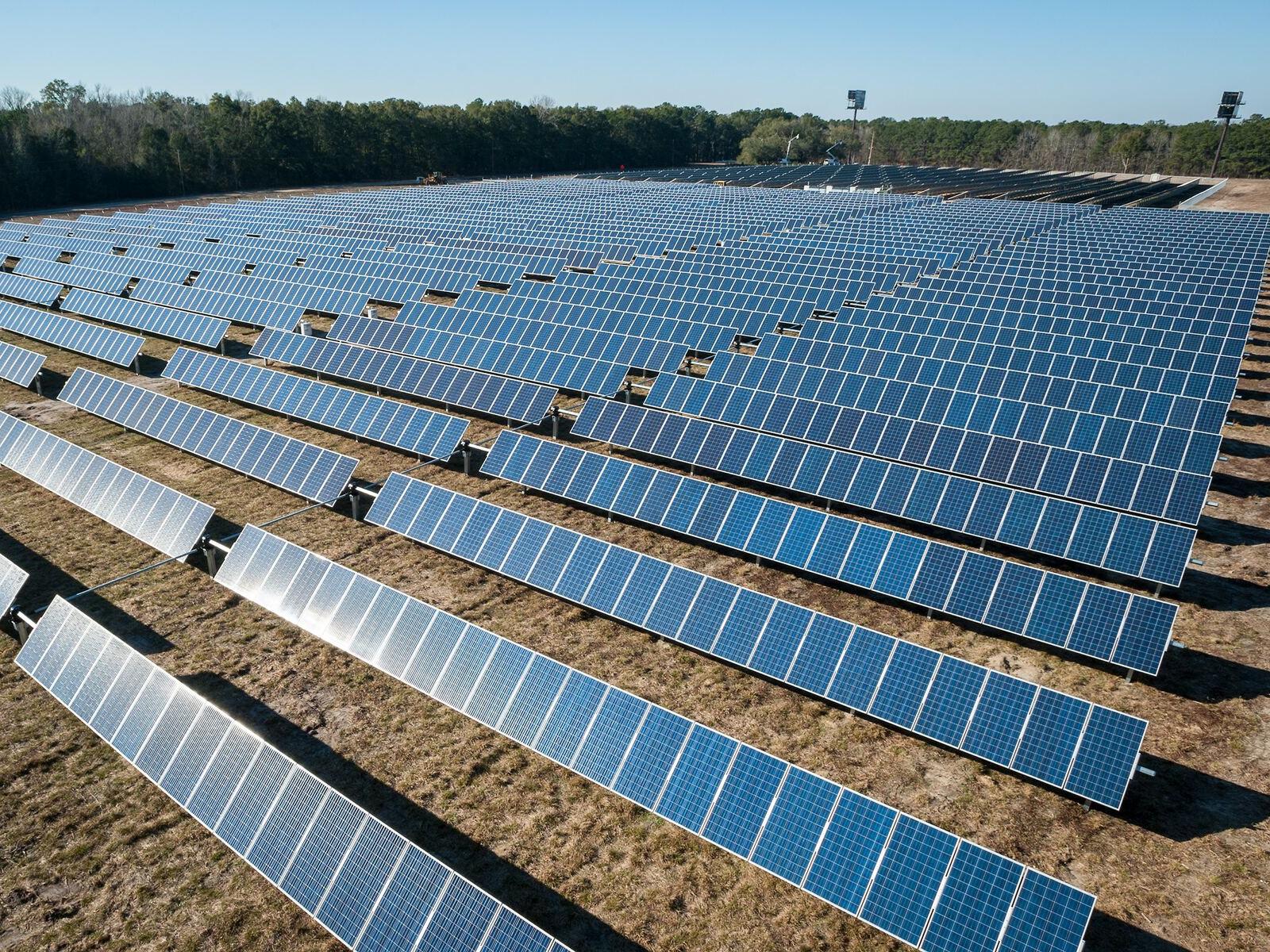
Lab-Level Communications Priority Topics
Grid
POC: Bharat Vyakaranam
Power system planning engineers currently perform planning studies based on a few snapshots that represent typical system operating points (aka base power flow cases). However, that approach is not sufficient to fully understand and evaluate operational impacts of the current and future grid, especially under high uncertainty and extreme events. We need to examine the solutions of thousands of chronological power flow cases rather than picking a small number of selected operating conditions as is done conventionally. Load is no longer the only consideration, but wind, solar, gas availability, and hydro generation can change significantly over time. To perform robust short- and long-term planning, there is a need to analyze a large number of scenarios to cover unexpected situations. To assess a large number of scenarios and conduct detailed modeling, an efficient framework is required to overcome the existing computational challenges associated with large scale networks.