CDI Project: Uncertainty Quantification for Complex Systems
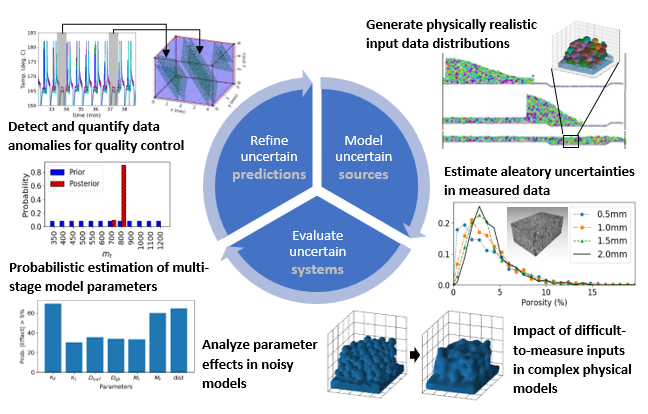
Return to Data and Integration Activities
PI: W. Steven Rosenthal
Project Team: Francesca Grogan, Amra Peles, Marvin Warner
Project Term: November 2019 to September 2021
Key Science Questions:
- Can the microstructure in additively manufactured parts be predicted accurately by assimilating experimental data and physical models while accounting for input and measurement uncertainties?
- Can chemical process model parameters be constrained by noisy, sparse, and partial observations to improve model fits and predict the impact of new assays?
- Can uncertainty in components of complex chemical models be analyzed and used to improve model selection and understanding of underlying chemical mechanisms?
Project Description: For several complex chemical systems, there are critical needs for accurate quantification of input and data uncertainties, efficient parameter estimation techniques, and statistical understanding of the impact of model and data quality on our understanding of chemical mechanisms. This project works across the CDI with a focus on:
- improvements in the representation of uncertainty in dynamic systems
- propagation of uncertainty in complex systems and dimension reduction
- parameter uncertainty in computational chemical models constrained by data
To achieve this, the project team has:
- assimilated phase-field modeling with non-destructive thermal and X-ray imaging techniques to validate a model for microstructure prediction in components additively manufactured by partial-sintering processes
- refined statistical analysis techniques for chemical reaction networks by deriving models to account for spatial/temporal inhomogeneity in measured data, trade-offs between data frequency and accuracy (NMR), and test potential side reactions to validate proposed reaction models
- analyzed measurement error and developed model selection techniques for thermo-gravimetric analysis of polymers, which account for both model and data uncertainties and enables scientists to identify the most likely underlying chemical mechanisms