CDI Project: State Space Discovery and Data-Driven Modeling of Chemical Systems
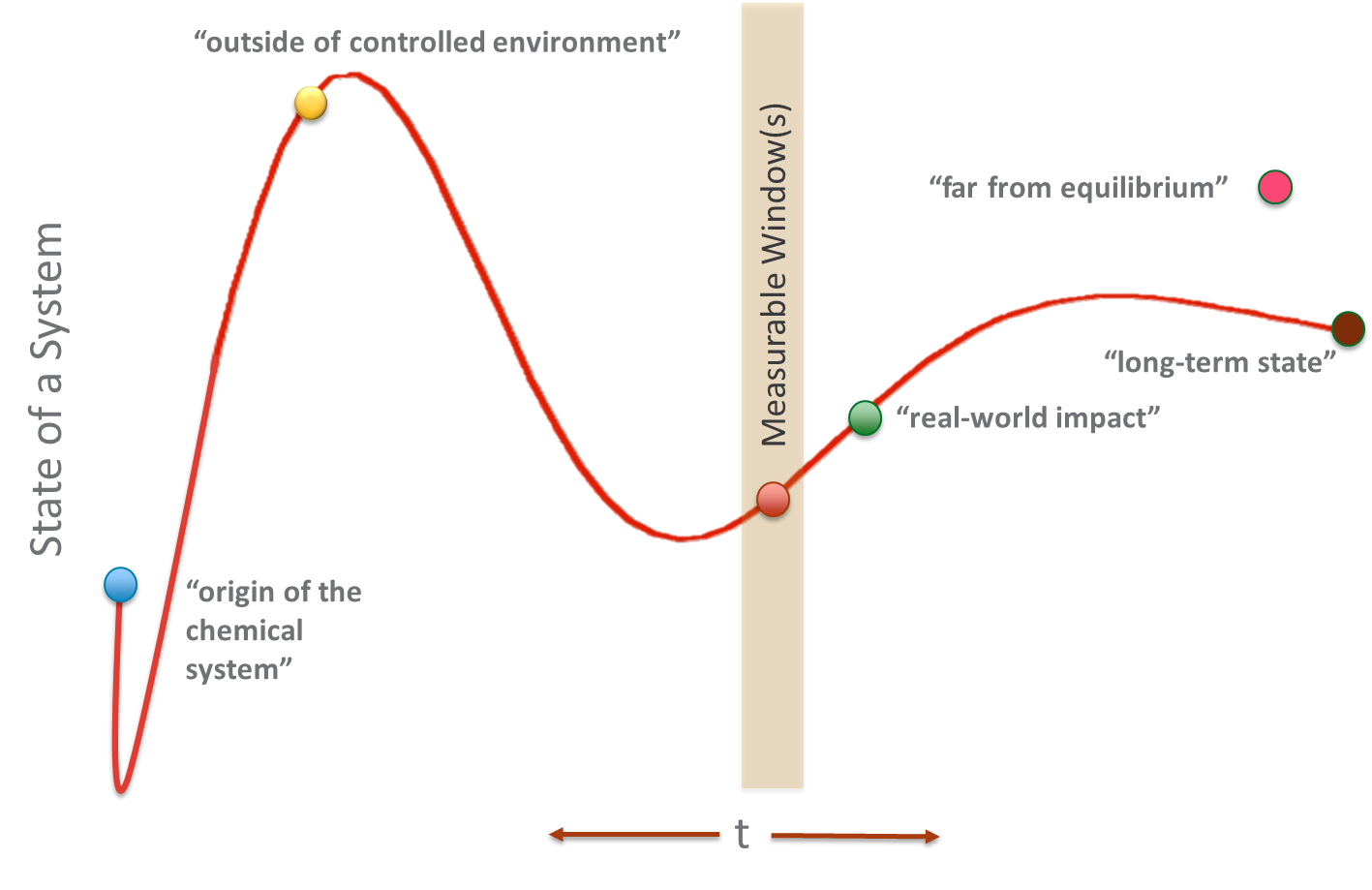
Return to Data and Integration Activities
PI: Carlos M. Ortiz Marrero
Project Team: Michael S. Hughes, Francesco Luzi, Jenna Pope
Project Term: May 2018 to September 2020
Key Science Questions:
- What data-driven tools describe time-evolving chemical systems?
- How can data-driven models take advantage of chemical knowledge and problem structure?
- What tools should be applied when dealing with limited experimental data?
Project Description: This project has developed a variety of data-driven models that extract insights about chemical processes. The project goal is to identify, extend, and implement data-driven tools that leverage knowledge of the underlying chemical system of interest for a spectrum of experimental scenarios (e.g., limited data, big data). The project team has:
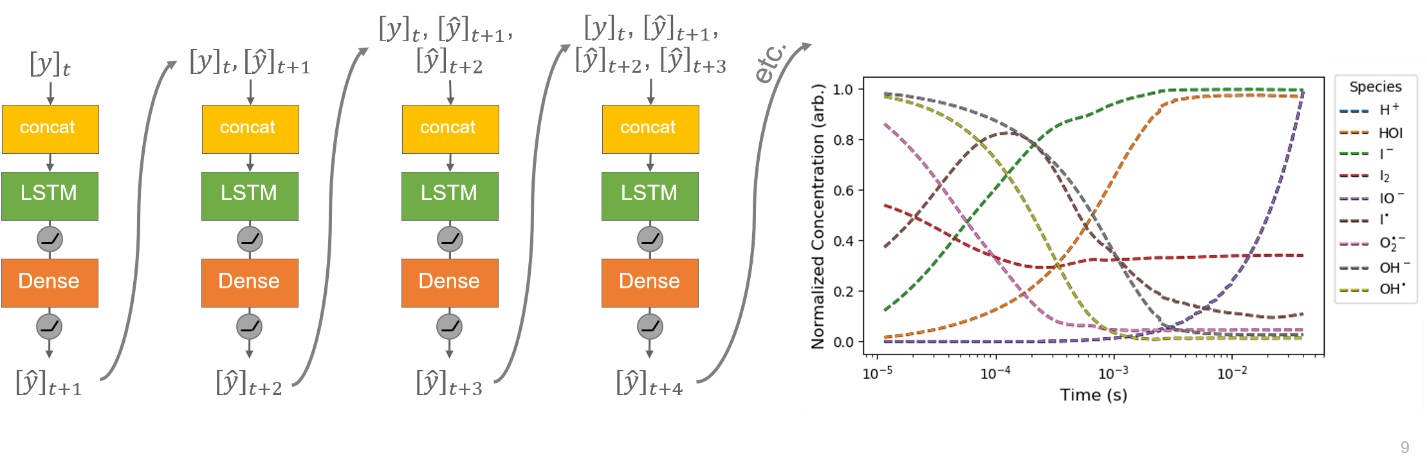
- utilized delay-embedding theorems (e.g., Takens’ Theorem) to get an appropriate reconstruction of the chemical system with limited data
- developed predictive data-driven models for large dimensional iodine systems using a chemistry-informed neural network
- developed a classification algorithm to detect annealed 3D printed components using sparse ultrasonic measurements obtained from limited samples
Project Publications:
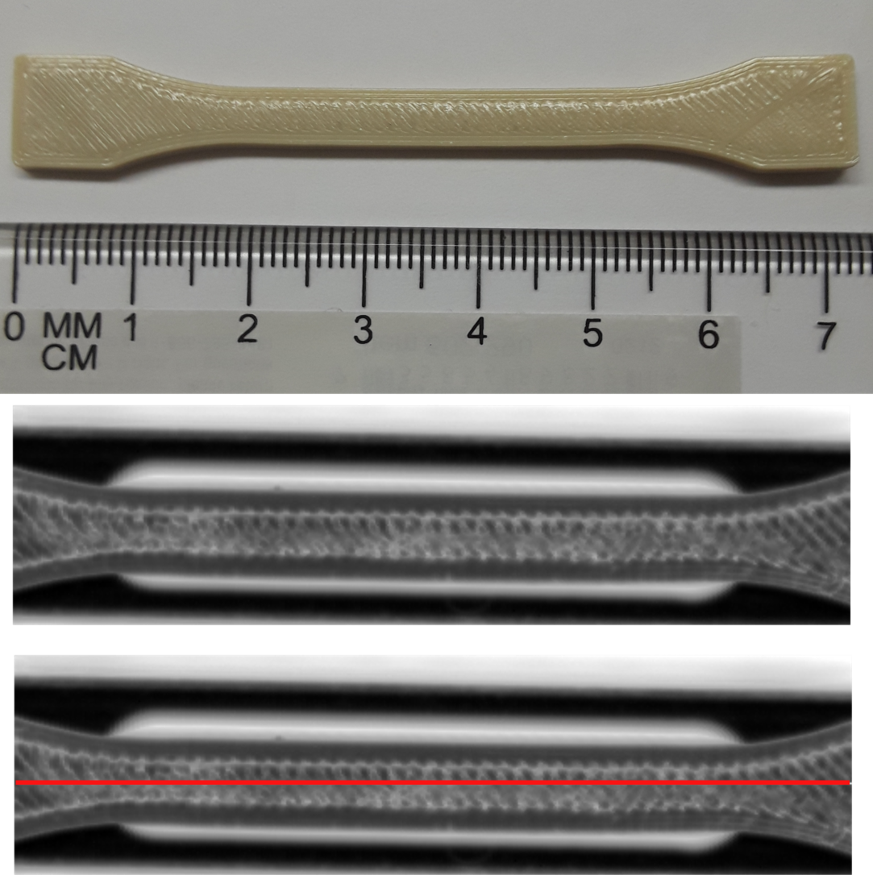
- Bilbrey J, C Ortiz-Marrero, M Sassi, A Ritzmann, N Henson, M Schram. 2020. “Tracking the Chemical Evolution of Iodine Species Using Recurrent Neural Networks.” ACS Omega, vol. 5, no. 9, pp. 4588–4594, 10.1021/acsomega.9b04104
- Luzi F, M Fenn, J Christ, Z Kennedy, T Varga, M Hughes, C Ortiz-Marrero. 2020. “Acoustic Properties of 3D Printed Polyetherketoneketone Components,” Journal of the Acoustical Society of America (Submitted March 2020)
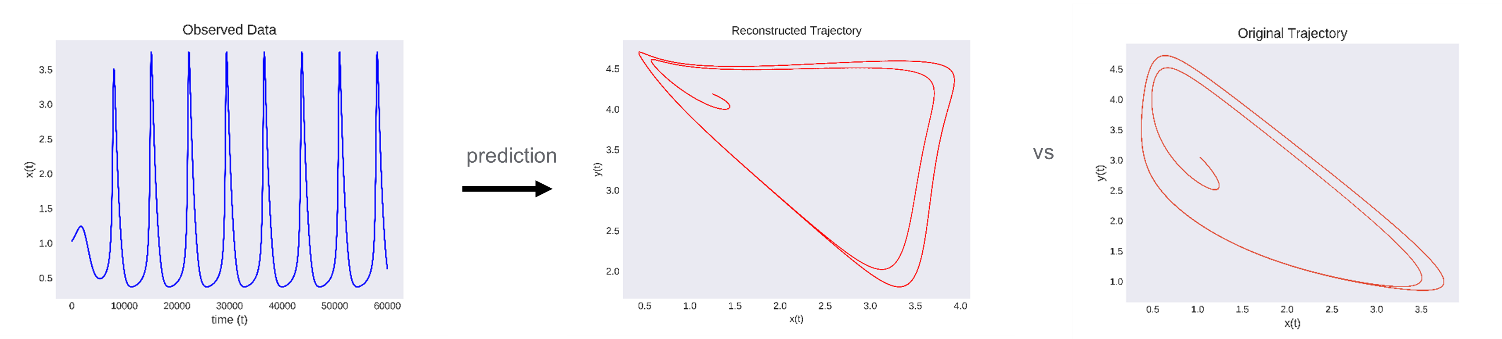